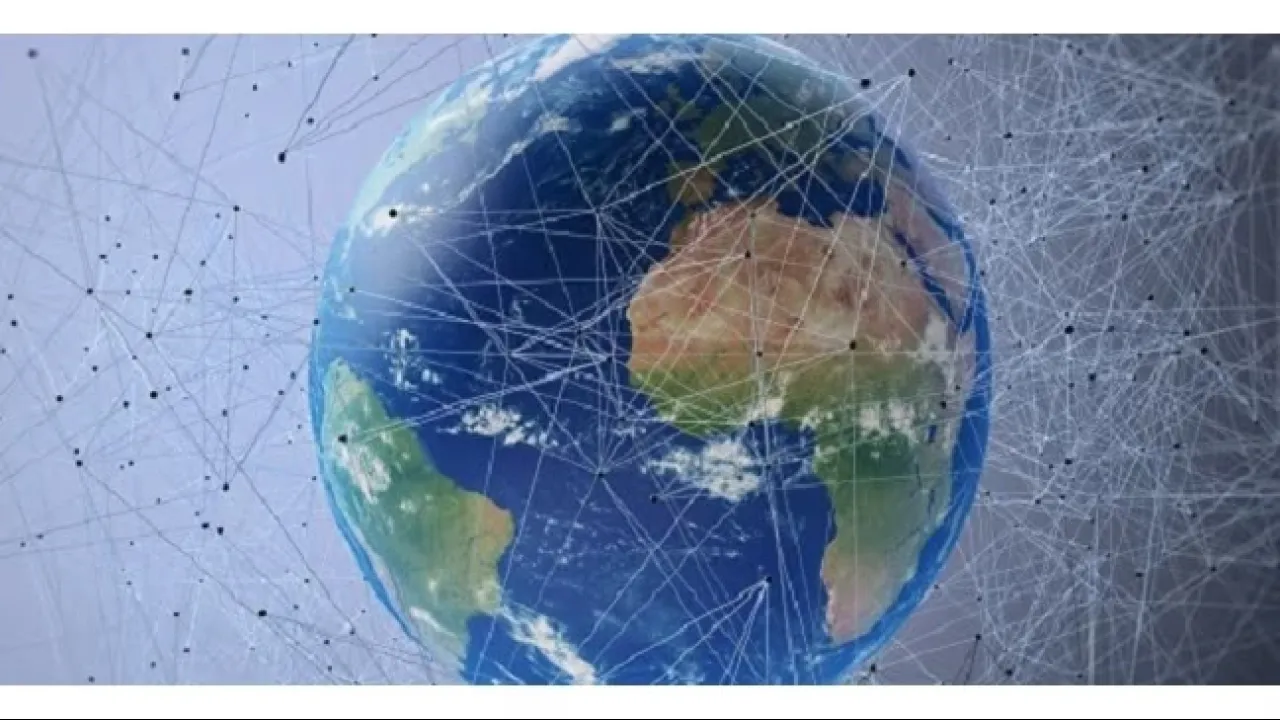
Divide and conquer pattern searching
About
Searching for recurring patterns in network systems has become a fundamental part of research and discovery in fields as diverse as biology and social media. KAUST researchers have developed a pattern or graph-mining framework that promises to significantly speed up searches on massive network data sets.
“A graph is a data structure that models complex relationships among objects,” explained Panagiotis Kalnis, leader of the research team from the KAUST Extreme Computing Research Center. “Graphs are widely used in many modern applications, including social networks, biological networks like protein-to-protein interactions, and communication networks like the internet.”
In these applications, one of the most important operations is the process of finding recurring graphs that reveal how objects tend to connect to each other. The process, which is called frequent subgraph mining (FSM), is an essential building block of many knowledge extraction techniques in social studies, bioinformatics and image processing, as well as in security and fraud detection. However, graphs may contain hundreds of millions of objects and billions of relationships, which means that extracting recurring patterns places huge demands on time and computing resources.
“In essence, if we can provide a better algorithm, all the applications that depend on FSM will be able to perform deeper analysis on larger data in less time,” Kalnis noted.
Kalnis and his colleagues developed a system called ScaleMine that offers a ten-fold acceleration compared with existing methods.
“FSM involves a vast number of graph operations, each of which is computationally expensive, so the only practical way to support FSM in large graphs is through massively parallel computation,” he said.
Read the full article