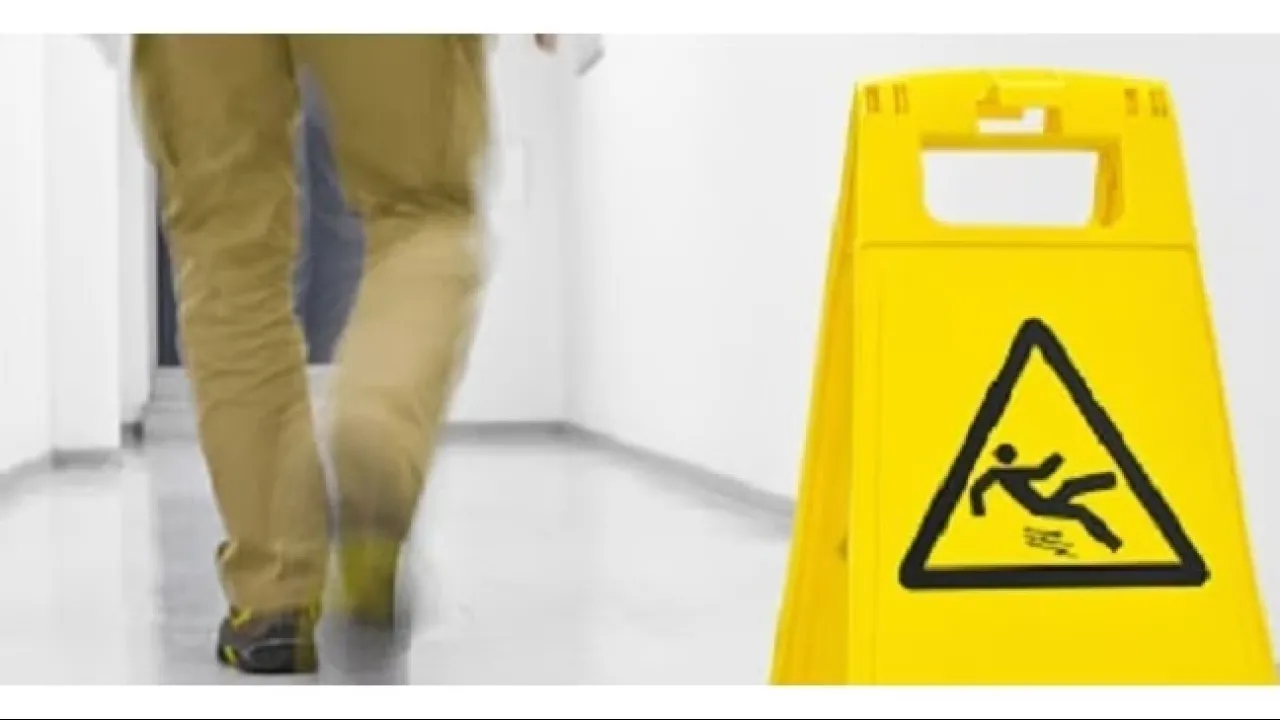
Smart healthcare uses stats to spot a stumble
Statistical monitoring technology can detect serious falls and immediately warn healthcare providers.
About
Falls by elderly people can cause serious injury or death if sufferers remain on the ground for too long. By combining data from both wearable sensors and video surveillance, a team at KAUST has developed a statistical scheme that detects when senior citizens or others need help after falling.
Falls have distinct signs, such as sudden changes in acceleration, which make them trackable with technology. Over the last 20 years, researchers have tackled this problem with sensors that measure movements or computers that analyze shapes in video images. Both approaches, however, have trouble distinguishing mild slips from serious incidents and consequently have high false alarm rates.
The University’s Assistant Professor Ying Sun and co-workers used statistics to improve fall detection. While accelerometers in most wearable sensors use manually set thresholds to trigger an alert signal, the KAUST team used exponentially weighted moving average (EWMA) charts to dynamically monitor acceleration data over time. Any unusual changes to a person’s movements are then identified as sharp deviations from the expected, averaged dataset.
“The EWMA monitoring technique is effective in detecting falls because it is sensitive to small changes,” explained Sun. “Its low computational cost also means it can be easily implemented in real time.”
Read the full article