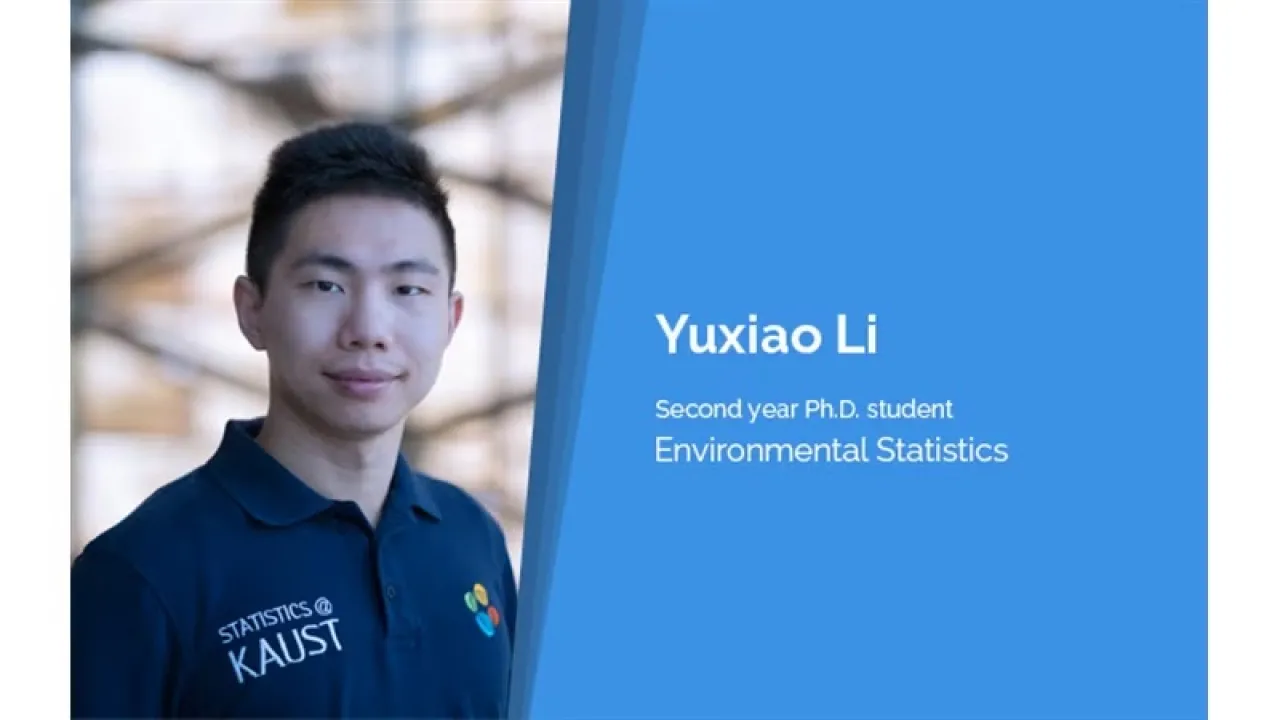
Ph.D. Student Yuxiao Li won the ENVR student paper award
KAUST second year Ph.D. student Yuxiao Li has been selected to receive a Student Paper Award from the American Statistical Association (ASA) for his paper entitled "Efficient Estimation of Non-stationary Spatial Covariance Functions with Application to High-resolution Climate Model Emulation."
About
KAUST second year Ph.D. student Yuxiao Li has been selected to receive a Student Paper Award from the American Statistical Association (ASA) for his paper entitled "Efficient Estimation of Non-stationary Spatial Covariance Functions with Application to High-resolution Climate Model Emulation." Li, who is a member of the Environmental Statistics Group led by Professor Ying Sun in the University's Computer, Electrical and Mathematical Science and Engineering (CEMSE) Division, will receive his award at the ASA's Joint Statistical Meeting (JSM) 2018. The award-winning paper is Li's first research paper at KAUST.
JSM 2018 is the largest annual gathering of statisticians and data scientists held in North America. The event provides the opportunity for statisticians in academia, industry and government to exchange ideas and explore collaboration opportunities. This year's event will be held in Vancouver, Canada, from July 28 to August 2.
Li is quick to acknowledge all those who have assisted him in his research and studies, culminating with the award.
"I feel very grateful for all the support and guidance I have received, especially from my adviser Professor Ying Sun. This award gives me confidence for my future research and encourages me to pursue a promising academic career," Li said.
A space-time precipitation focus
Describing the background research for his paper, Li said, "In our paper, statisticians attempt to avoid unrealistic assumptions and estimate the environmental processes more accurately. The stationarity assumption in spatial covariance functions is convenient but unrealistic. Complex models without the stationarity assumption, however, typically suffer from a heavy computational burden. Therefore, an efficient estimation method for the non-stationary model is necessary."
"In this study, we propose a new estimation procedure to approximate a class of non-stationary Matérn covariances that function by the local-polynomial fitting of the covariance parameters. The proposed method allows for efficient estimation of a richer class of non-stationarity with the local stationary model as a special case," he added.
Li joined KAUST in August 2016 to pursue his Ph.D. studies in the field of statistics, and his current research focus is on short-term space-time precipitation modeling.
"[Short-term space-time precipitation modeling] is an even more challenging problem because we need to model both rainfall zeros and rainfall amounts, and the spatio-temporal dependence structure is rather complex," he noted.
-By David Murphy, KAUST News