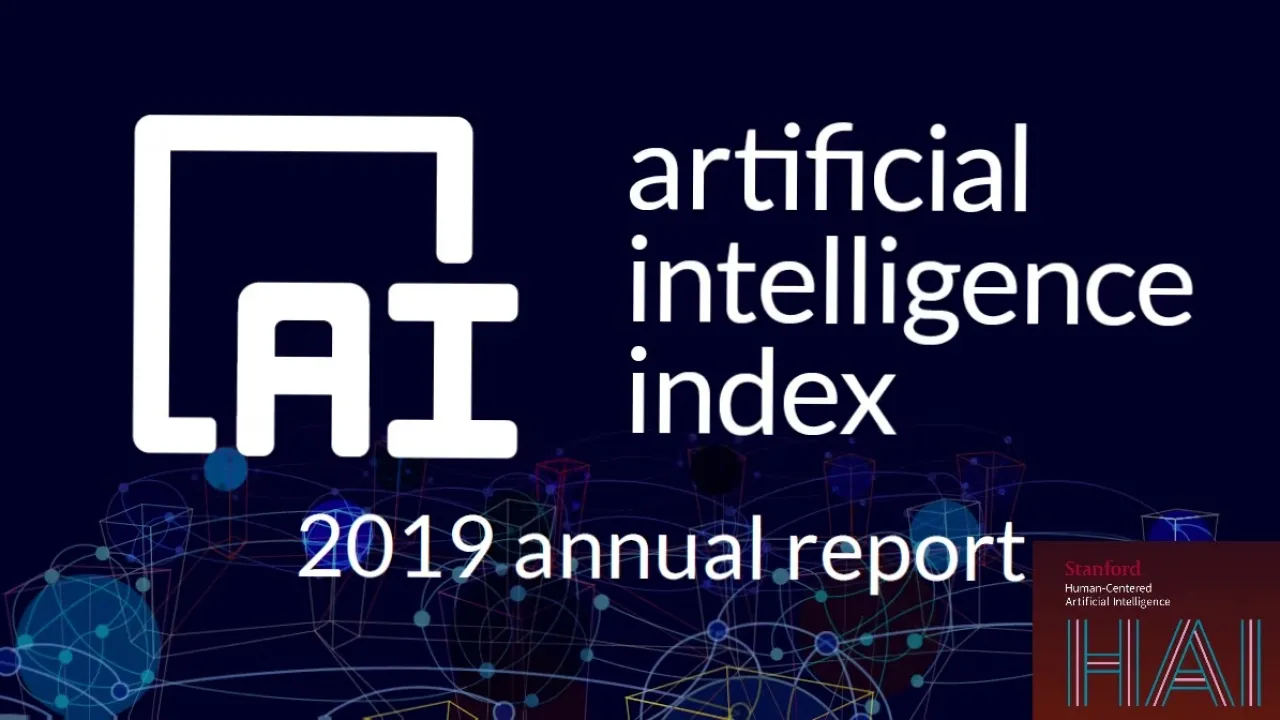
ActivityNet is featured in the 2019 Artificial Intelligence Index
ActivityNet is featured in the AI Index as the benchmark for the algorithms that can recognize human actions and activities from videos.
About
The AI Index is an independent initiative at Stanford University’s Human-Centered Artificial Intelligence Institute (HAI).
The AI Index Report tracks, collates, distills, and visualizes data relating to artificial intelligence. Its mission is to provide unbiased, rigorously-vetted data for policymakers, researchers, executives, journalists, and the general public to develop intuitions about the complex field of AI. Expanding annually, the Report endeavors to include data on AI development from communities around the globe.
Chapter 3 of the AI index tracks technical progress in computer vision (images, videos, and image+language) and natural language processing tasks. ActivityNet is featured as the benchmark for the algorithms that can recognize human actions from videos.
Our benchmark aims at covering a wide range of complex human activities that are of interest to people in their daily living. We illustrate three scenarios in which ActivityNet can be used to compare algorithms for human activity understanding: global video classification,trimmed activity classification and activity detection.
The emergence of large-scale datasets such as ActivityNet and Kinetics has equipped computer vision researchers with valuable data and benchmarks to train and develop innovative algorithms that push the limits of automatic activity understanding. These algorithms can now accurately recognize hundreds of complex human activities such as bowling or sailing, and they do so in real-time. However, after organizing the International Activity Recognition Challenge (ActivityNet) for the last four years, we observe that more research is needed to develop methods that can reliably discriminate activities, which involve fine-grained motions and/or subtle patterns in motion cues, objects, and human-object interactions. Looking forward, we foresee the next generation of algorithms to be one that accentuates learning without the need for excessively large manually curated data. In this scenario, benchmarks and competitions will remain a cornerstone to track progress in this self-learning domain.
-- Bernard Ghanem, Associate Professor of Electrical Engineering (IVUL)