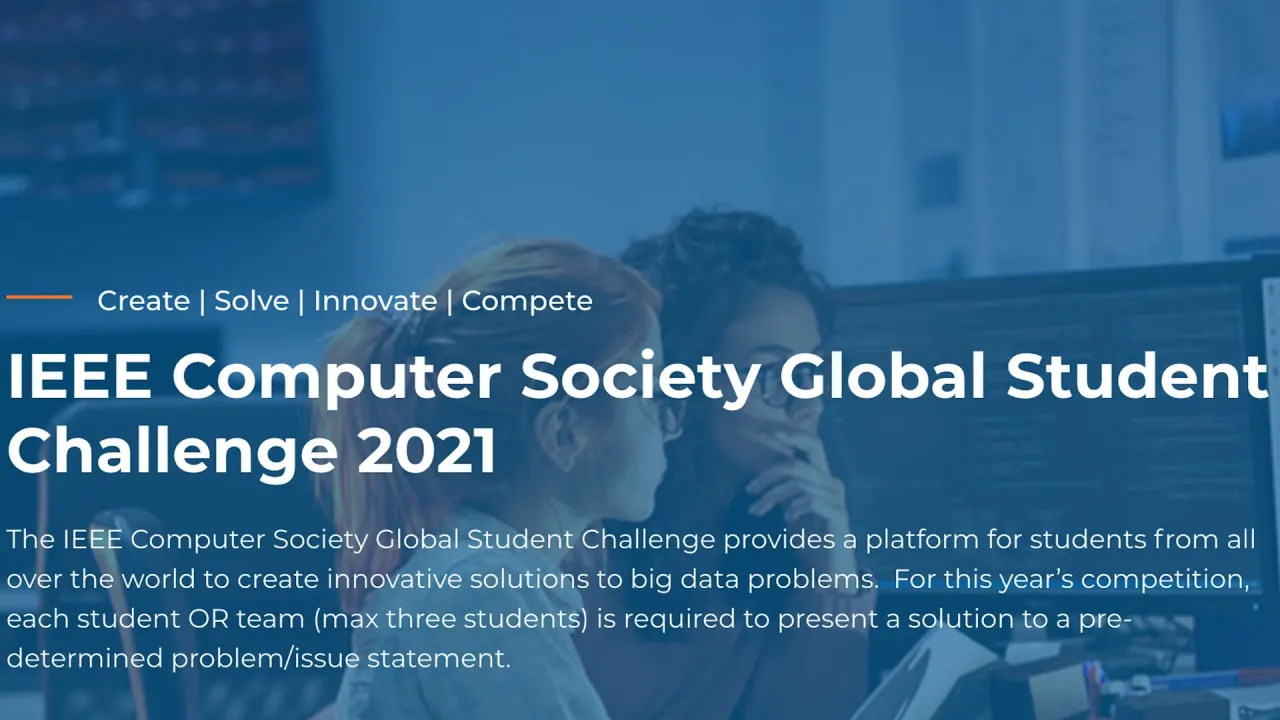
The IEEE CS Global Student Challenge seeks top KAUST CS talent
The annual IEEE Computer Society Global Student Challenge provides an avenue for students worldwide to create innovative solutions to big data problems. This year’s competition, IEEE CS GSC 2021, encourages each individual or team (max three students) to present solutions to pre-determined problem/issue statement of relevance in CS today.
About
By David Murphy
The annual IEEE Computer Society Global Student Challenge provides an avenue for students worldwide to create innovative solutions to big data problems. This year’s competition, IEEE CS GSC 2021, encourages each individual or team (max three students) to present solutions to pre-determined problem/issue statement of relevance in CS today.
Submissions will be evaluated based on quantitative performance on predicting events in an unseen dataset, an oral presentation given by the participants to a team of international judges, and the solution’s novelty. The three winning teams will be awarded virtually.
Co-sponsored by KAUST and Purdue University, U.S., the event is based on two challenge problems created by the respective universities: “Analyzing computer system usage and failure data from a university’s central computing system” (Purdue), and “Analyzing sentiments in tweets related to the COVID-19 pandemic” (KAUST).
Both challenges have been created by KAUST Professor Xiangliang Zhang and her counterpart at Purdue, Professor Saurabh Bagchi. The first challenge aims to predict if a “job” (application execution) submitted to a computing cluster will fail or succeed. Job failure leads to resource wastage and user dissatisfaction.
“The prediction is challenging because jobs are heterogeneous for their compute-intensive, memory-intensive, IO-intensive properties. Therefore, an actionable failure prediction model based on resource usages will be helpful to reduce job failure/resource wastage for both the system user and system admin,” Zhang explained.
The KAUST challenge focuses on sentiment analysis or opinion mining. Sentiment analysis is a natural language processing technique used to determine the sentiment conveyed by natural language text. The challenge is a multi-label text classification task that uses the dataset collected by an open-source Twitter data crawler (Twint) to test participants with assigning multiple labels associated with one COVID-19 related tweet. For example, “optimistic,” “thankful,” “empathetic,” “pessimistic,” “anxious,” “sad,” “annoyed,” etc.
“Most of today’s sentimental analysis tasks usually consider coarse-grained emotion labels like “positive,” “neutral,” and “negative” for reviews/comments of books/products/movies. However, people’s feelings during this pandemic are far more complicated than the sentiments encountered in movie and product reviews,” she noted.
Zhang believes that many of the submissions will focus on deep learning techniques and models: “There are different architectures, designs and application tricks for making the deep learning models successful on specific tasks. However, the sophisticated solutions that have high prediction accuracy are what we are looking for,” she concluded.
IEEE CS GSC 2021 prize information and registration details can be found below.
Prizes:
- First-place: $1,000
- First runner-up: $800
- Second runner-up: $500
- Audience choice winner: $300
- Special CS Award Winner: $400 (all team members must be active IEEE CS Members)
To register, please visit https://syp.computer.org/gsc/. The submission deadline is Tuesday, June 15, 2021.