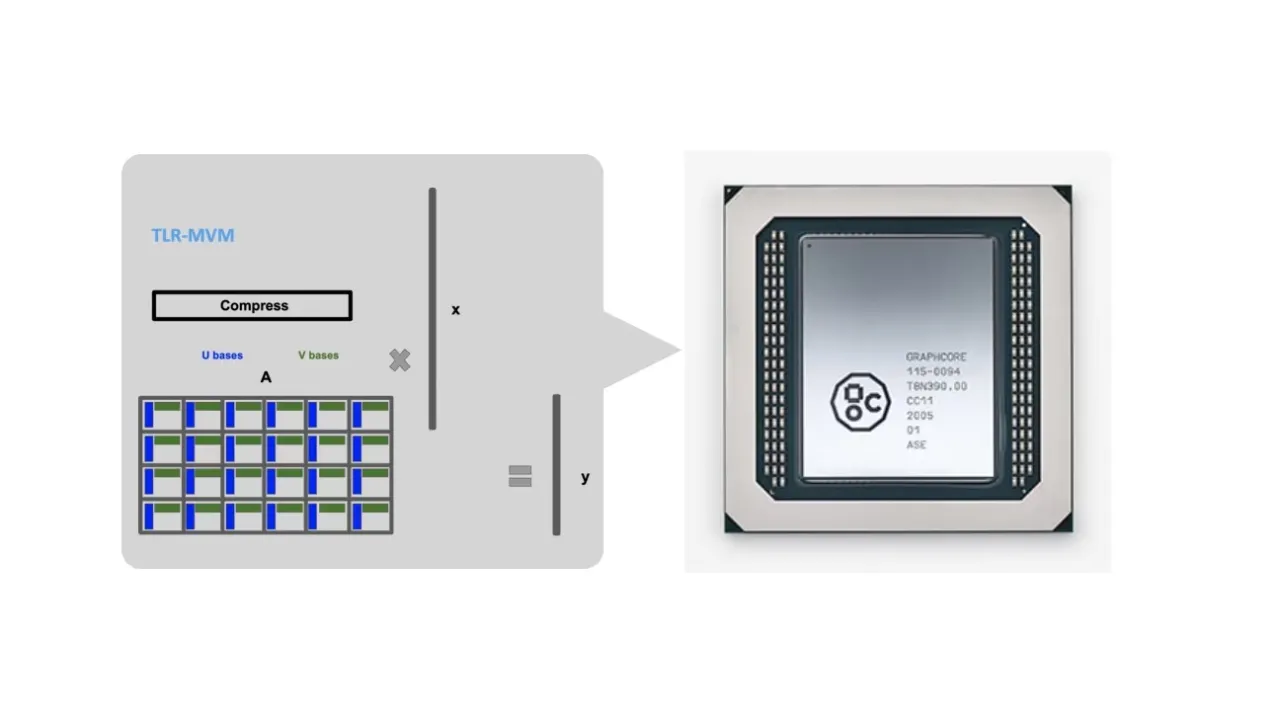
Steering Customized AI Architectures for HPC Scientific Workloads: A Bird of Feather at SC22!
About
Abstract:
We explore the possibilities of a hybrid system capable of solving both HPC and AI scientific problems. Such a hybrid architecture combines the synergism between classical HPC platforms and dedicated AI chip systems, which is important due to the computational challenges brought to the fore by massively parallel Exascale systems.
We discuss the system functionality, the algorithmic software adaptations, and performance considerations. We present efforts in supporting AI/ML applications in addition to seismic imaging, climate/weather prediction, and computational astronomy on hybrid systems. In particular, we investigate how Graphcore’s IPU can accelerate hybrid HPC applications, beyond the originally intended AI workloads.
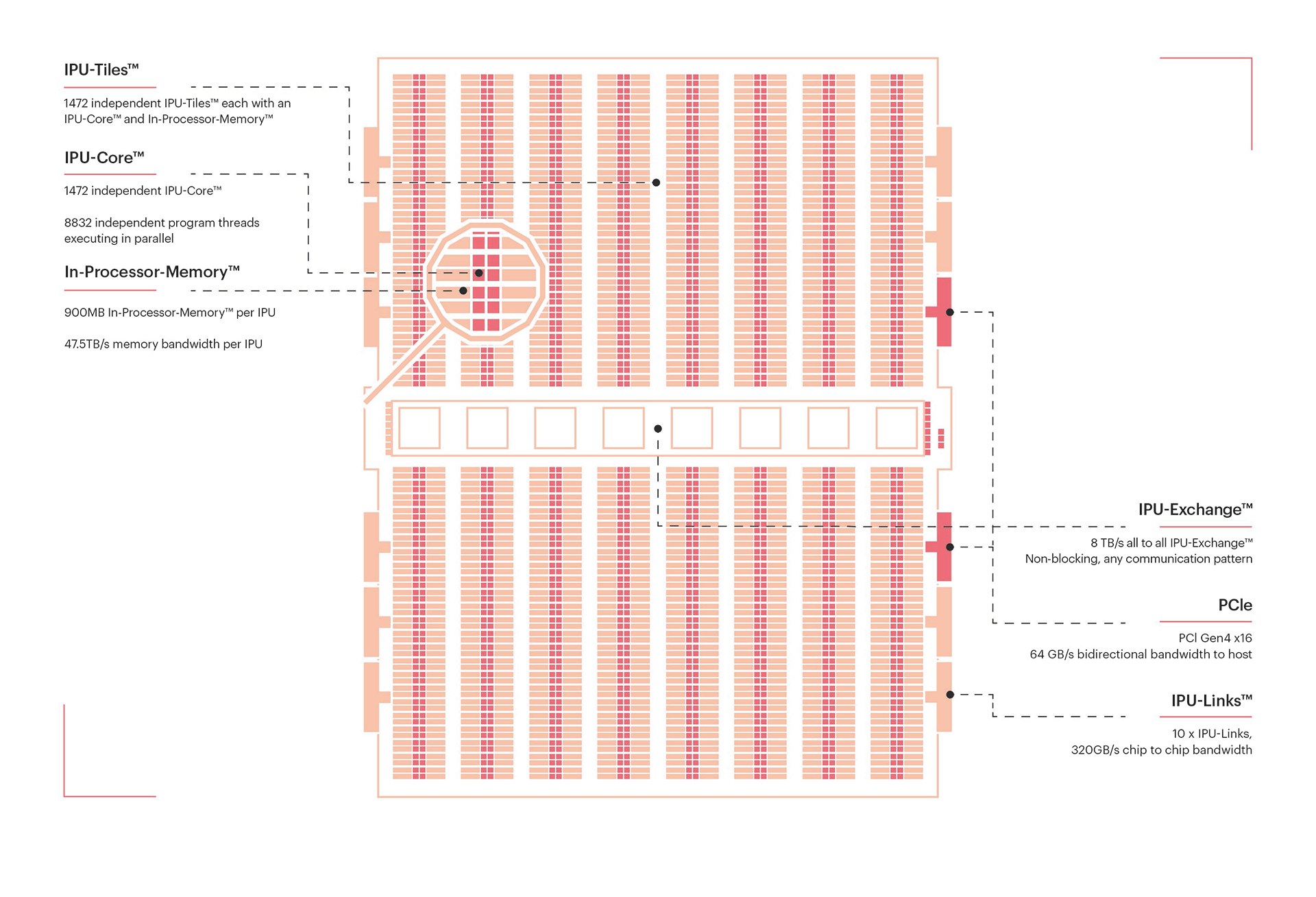
Motivations:
HPC scientific applications are undergoing a transformational change. These HPC workloads are increasingly accelerated using AI methods and deployed on general-purpose hardware accelerators and innovative hardware such as the Graphcore’s Intelligence Processing Unit (IPU). At the same time, there are attempts for some of these HPC applications resisting this trend to map them onto these customized AI chips by leveraging algorithmic adaptations.
In this BoF, we explore the possibilities of a heterogeneous system architecture capable of addressing both HPC and AI scientific problems. Such an architecture would need to utilize classical HPC platforms and dedicated AI chip systems, which is particularly important in the context of the computational challenges and energy consumption considerations raised by exascale systems. Beyond the hardware implications at the chip level, we also discuss the system functionality, the programming model, the necessary algorithmic software adaptation, and performance considerations.
Besides highlighting the latest hardware advancements for tackling AI workloads at an unprecedented throughput, we present the algorithmic redesign necessary to migrate HPC legacy applications into AI-friendly chips. The three existing hardware engines, i.e., matrix engines (from AMD/NVIDIA/Intel GPUs), wafer-scale engine (from Cerebras), and graph engines (from Graphcore's IPU) can be all exploited after performing algorithmic adaptations.
We show the effectiveness of these versatile numerical techniques, which cope with expensive data movement and on-chip memory scarcity characterizing these chips, using applications in seismic redatuming, wireless communications, and computational astronomy.

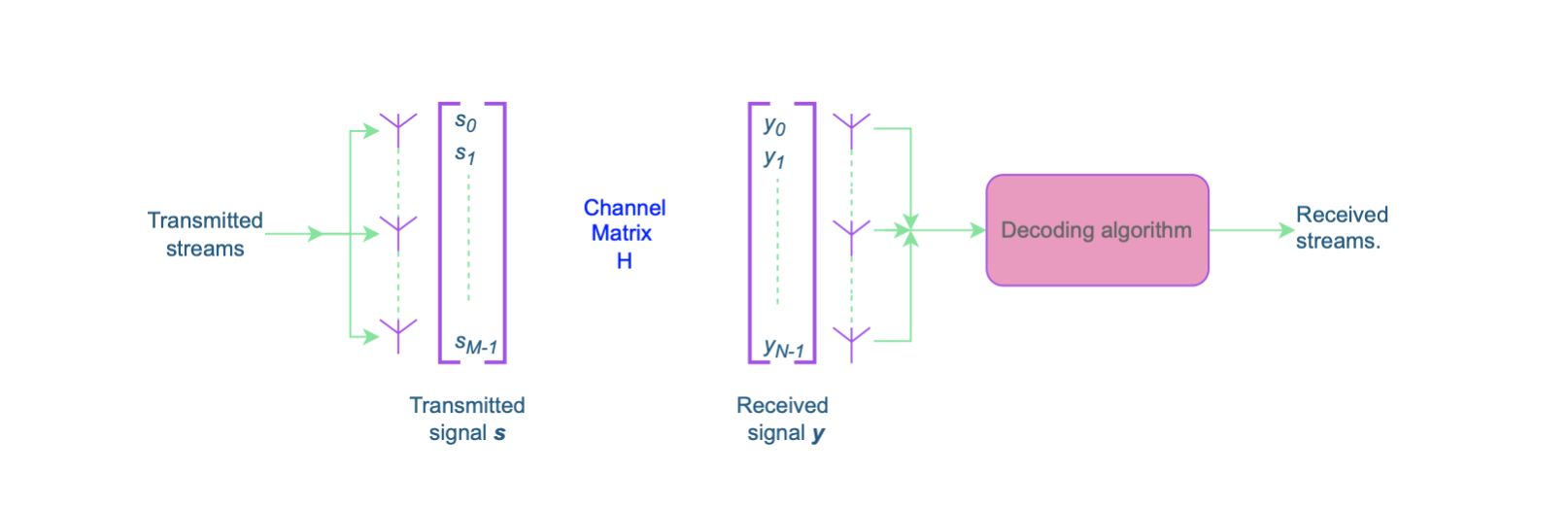
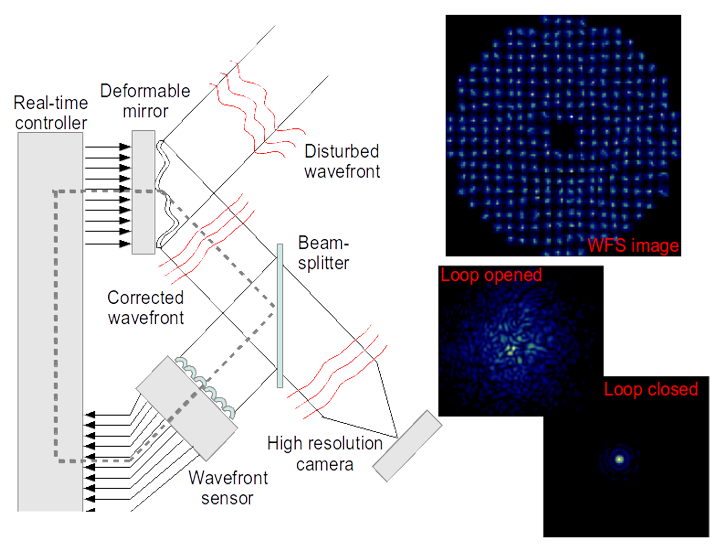
Because AI customized chips may turn out to have a broader mandate than initially intended, join the live discussions with experts and engage with the audience on defining the next steps for embracing the advent of a computing world, where heterogeneity plays a central role.
Organizers:
- Paweł Gepner, Senior Hardware & Systems Field Application Engineer, Graphcore
- Hatem Ltaief, Principal Research Scientist, ECRC, KAUST
- Lukasz Anaczkowski, HPC AI Customer Engineering Team Manager, Graphcore
- Hubert Chrzaniuk, HPC AI developer, Graphcore
SC22 BoF Date, Time and Location:
Wednesday, November 16th 17:15pm-18:45pm
Room D-172