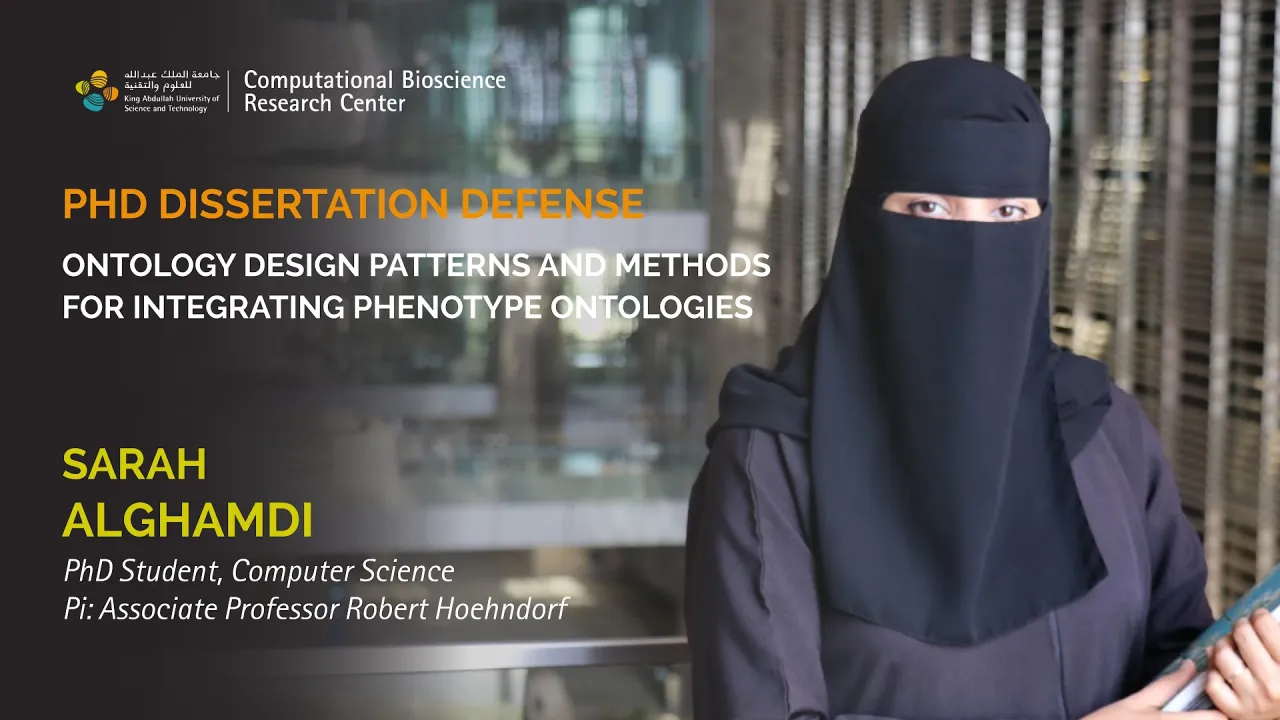
PhD Dissertation Defense | Ontology design patterns and methods for integrating phenotype ontologies
CBRC PhD student Sarah Alghamdi successfully defended her dissertation last week. She has been an essential member of the Bio-Ontology Research Group (BORG) since 2015.Her research interests include the applications of artificial intelligence and statistical methods to genomics and healthcare.
About
Abstract:
Ontologies are widely used in various domains, including biomedical research, to structure information, represent knowledge, and analyze data. Combining ontologies from different domains is crucial for systematic data analysis and comparison of similar domains. This requires ontology composition, integration, and alignment, which involve creating new classes by reusing classes from different domains, aggregating types of ontologies within the same domain, and finding correspondences between ontologies within the same or similar domain.
This thesis presents use cases where we applied ontology composition, integration, and alignment and evaluated the resulting ontologies and alignment. First, I analyzed a large aging dataset of inbred laboratory mice, using Mouse Anatomy and Mouse Pathology ontologies. Second, we integrated phenotype ontologies for human and model organism phenotypes to enable comparisons of phenotypes between and within individual species. We developed Pheno--e, which represents an extension of PhenomeNet. We identified novel abnormal anatomical classes for fly phenotypes, allowing the annotation of fly genes that were not annotated before. We demonstrate the distinct contributions of each species' phenotypic data to detecting human diseases using Pheno--e, and show mouse phenotypic data contributes the most to the discovery of gene-disease associations. This work could guide the selection of model organisms when building methods to find gene-disease associations.
Additionally, We refined class definitions in phenotypic ontologies, specifically targeting cell cardinality phenotypes. This representation resolved incorrect inferences in the utilized ontologies, enabling accurate interpretation of phenotypic descriptions. Our findings reveal this correction enhances gene-disease prediction for diseases associated with cardinality phenotypes. Third, we introduce a novel neural-symbolic method that combines logic fundamentals with machine learning for ontology alignment. This method begins with symbolic representation, followed by iterative neural learning for alignment and symbolic representation consistency checking and reasoning, and back to neural learning. We demonstrate that our system generates noncontroversial alignments first and that these alignments are coherent with respect to OWL EL. This novel method can pave the way for more accurate and efficient ontology-based methods, which can have significant implications for various semantic web applications.
Biography:
Sarah M. Alghamdi is a Ph.D. candidate in Computer Science at the Computer, Electrical, and Mathematical Sciences and Engineering Division (CEMSE) of King Abdullah University of Science and Technology (KAUST). She is a member of the Bio-Ontology Research Group (BORG) and a faculty member in the Computer Science department at King Abdulaziz University (KAU) in Rabigh, Saudi Arabia. Sarah's research interest is in Artificial Intelligence applications in biomedical applications, specifically in leveraging biomedical ontologies to enable computational reasoning over complex biomedical data.
Sarah received her B.S. degree in Computer Science and Artificial Intelligence track from KAU in 2014. She then received her M.S. in Computer Science from KAUST in 2018. Sarah has presented her work at several conferences, including the International Conference on Biomedical Ontology (ICBO), the International Society for Computational Biology (ISMB), and the Computational and Statistical Interface to Big Data. She has also participated in several international workshops and tutorials, including The Semantic Web Applications for Health Care and Life Sciences conference (SWAT4HCLS), The International Workshop on Ontology Matching (OM), the Role of Ontologies in Biomedical AI (ROBI) tutorial and workshop, and the International Conference on Biomedical Ontology (ICBO).
During her PhD, Sarah worked as a teaching assistant for courses including Knowledge Representation and Reasoning, and Special Topics in Artificial Intelligence. She has served as a reviewer for ICBO, the ISMB Bio-Ontologies track, BMC Medical Informatics and Decision Making, and the Journal of Biomedical Semantics (JBMS). In 2022, Sarah's research was recognized with the Best Poster Award at ICBO. Her paper “Contribution of model organism phenotypes to the computational identification of human disease genes” was selected as the Editor’s Choice article in Disease Models and Mechanisms.