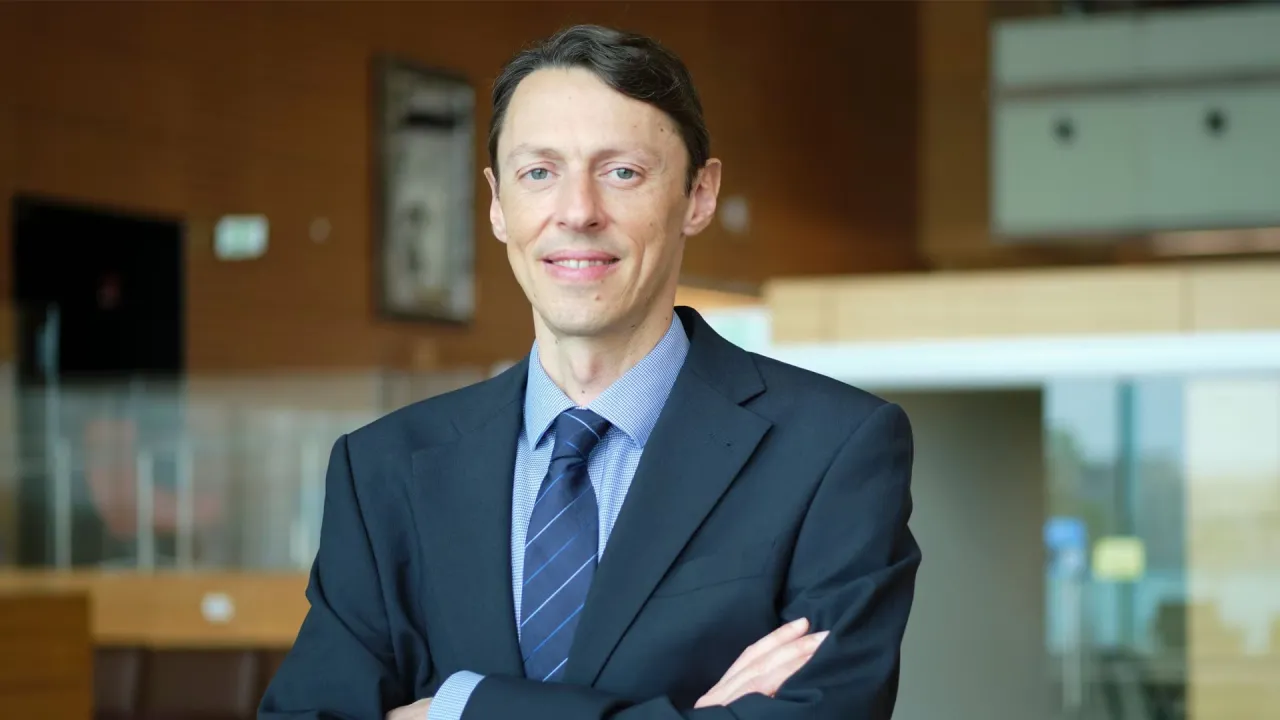
CEMSE New Faculty: Maurizio Filippone, Associate Professor, Statistics
Newly appointed to KAUST, Professor Filippone drives innovation in statistical modeling to advance sustainable solutions and address societal challenges.
About
“Strive for excellence.” These three succinct words convey the guiding principle of one of KAUST’s newest faculty members, Professor Maurizio Filippone. The associate professor of statistics, who joined KAUST earlier this year, believes excellence in research is attainable through constant collaboration and the continual reimagining of one’s work.
“When I was younger, I tended to be protective of my ideas; however, now I believe that sharing ideas is a tremendously powerful way to improve them, ultimately leading to consistently better work,” he emphasized. “It may be easy to say this when one has published many research papers; however, I am convinced a collaborative attitude pays off in the long run.”
Professor Filippone explored the possibility of joining KAUST over a year ago when he first became aware of potential openings in the Statistics program. An existing knowledge of the University’s exceptional talent pool and the unparalleled support they receive greatly influenced his decision to move to Saudi Arabia.
“Moving here, I find it inspiring to discover more about the Kingdom’s vision for a sustainable future, which aligns with my research interests. I feel privileged and honored to have the opportunity to work in such a wonderful collaborative environment,” he noted.
“It has been inspiring to meet so many people driving innovative projects with the potential to positively contribute to the Kingdom and the world. I relish the opportunity to contribute to KAUST’s excellence in research and teaching.”
Making sense of data
Filippone primarily focuses on making sense of data in various scientific domains through statistical models and inferences. More specifically, he is interested in developing techniques capable of quantifying uncertainty in predictions due to data scarcity or the lack of an accurate mechanistic description of the phenomena involved.
At KAUST, he will make Bayesian inference for over-parameterized models practical and tractable. He seeks to equip popular models with the ability to quantify uncertainty so that it is possible to trust when the models “know they don’t know.”
The Italian researcher recognizes that applying Bayesian principles to neural network-based models is extremely challenging in both the computational and statistical sense, chiefly due to mathematical formulation and over-parameterization.
“Neural network-based models are popular as a "one-size-fits-all" solution to many problems due to their stunning predictive performance. However, this performance success generates problems with statistical inference, particularly because these models lack a principled way to handle uncertainty,” Filippone explained.
“The purpose of my research is to make inferences about these models that are practical and scalable. It will require efforts in the theory and methods of statistical inference and validation through compelling and impactful applications. In this respect, I am excited to explore developing new projects with my peers at KAUST where statistical modeling can be useful,” he added.
With a comprehensive research-to-application portfolio, Filippone has worked with neuroscientists to analyze study data to model degenerative diseases like Parkinson’s and Alzheimer’s. Additionally, he has worked on problems combining Bayesian inference with differential equation models to describe phenomena and computer simulators to simulate physical processes, with applications in systems biology and geoscience.
Exploring a sustainable approach to the Kingdom’s future
The University’s commitment to leading and accelerating science-based solutions to achieve its sustainability goals was central to Filippone’s decision to move to KAUST. A drive to reduce machine learning’s carbon footprint has seen the knowledgeable statistician investigate alternatives to transistor-based technology to perform computations, particularly using optics principles.
“It is not just about replacing old hardware with new hardware but also about designing new inference methods that can fully take advantage of a given low-power and fast hardware,” he noted. “In parallel to that direction, my research on rendering statistical inference practical and scalable aims to reduce the computational burden of carrying out inference for popular models, thus improving their carbon footprint.
“Ultimately, all these developments are in the direction of addressing pressing questions stemming from the need to address societal challenges, leveraging statistical methods for applications in sustainability. If we approach applications in sustainability with completely unsustainable computational methods, there is no net benefit in what we do,” Filippone concluded.