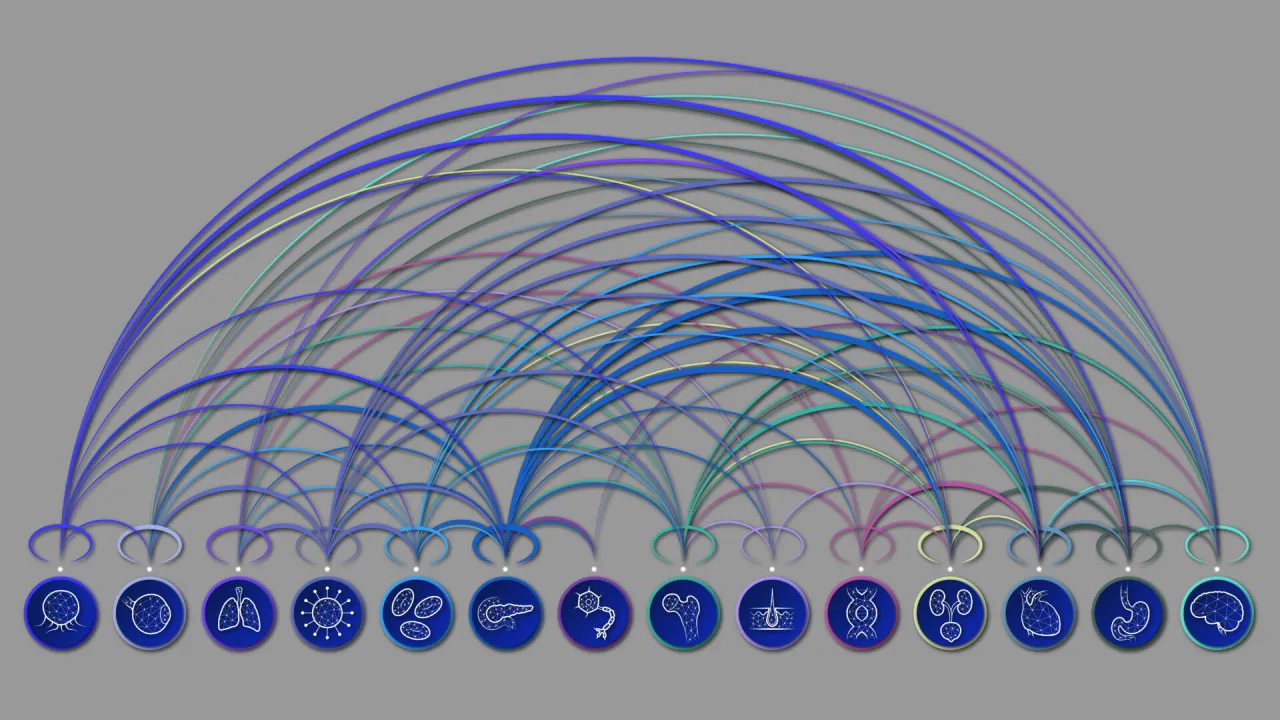
AI tool maps hidden links between diseases
Understanding how diseases trigger one another could lead to better treatments, prevention strategies and improved genetic risk prediction.
About
An AI-powered tool from KAUST researchers is helping scientists trace hidden connections between diseases, revealing insights into how one illness might lead to another and, by extension, how treating one illness could help prevent another.
By systematically combing through medical literature and real-world patient data, this tool maps cause-and-effect relationships, creating a framework that could guide targeted therapeutic strategies and uncover potential for drug repurposing.
Think of it as the ultimate disease relationship detective. Using natural language processing, the tool scans vast quantities of biomedical research to pinpoint causal connections — like how high blood pressure can set the stage for heart failure.
“Instead of treating diseases as unrelated outcomes, our approach facilitates the identification of shared risk factors among causally linked diseases,” says Sumyyah Toonsi, a graduate student in the Bio-Ontology Research Group. “This deepens our understanding of human diseases and enhances the performance of risk-prediction tools for personalized medicine.”
Further Information
Click here to read the full article on KAUST Discovery.
References
Toonsi, S., Gauran, I.I., Ombao, H., Schofield, P.N. & Hoehndorf, R. Causal relationships between diseases mined from the literature improve the use of polygenic risk scores. Bioinformatics 40, btae639 (2024).| article.