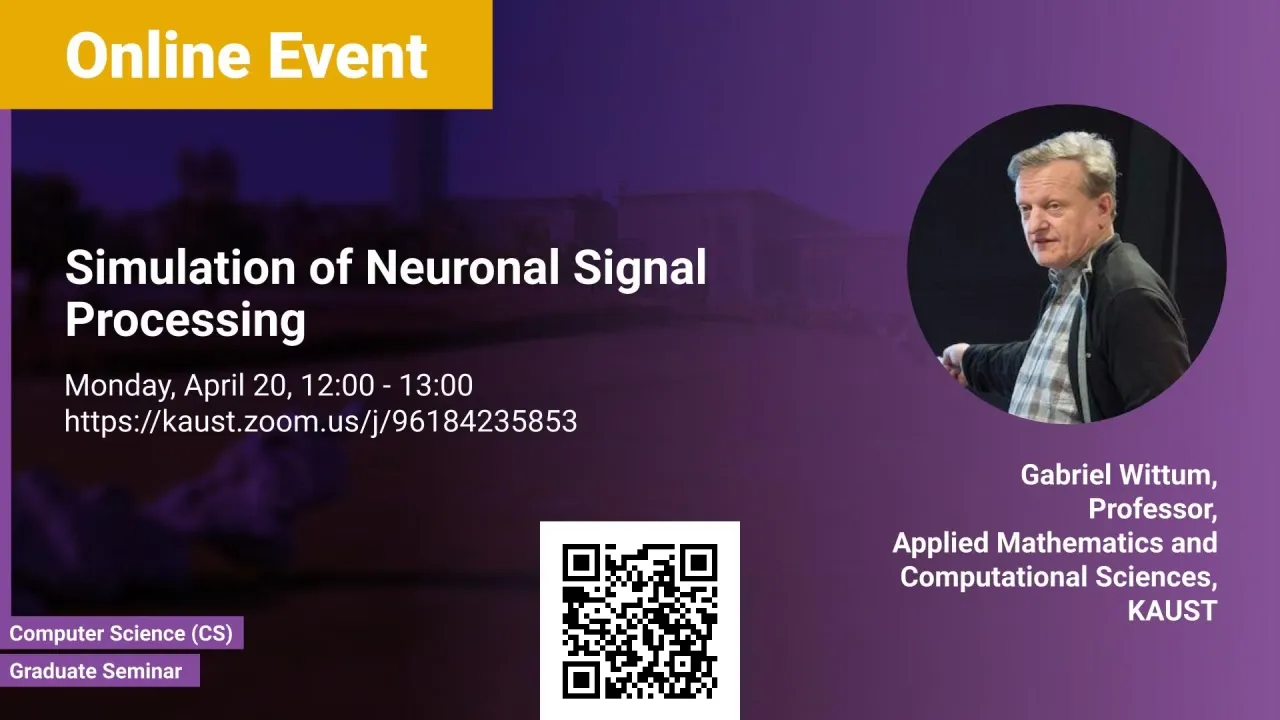
Simulation of Neuronal Signal Processing - 2020-04-20
In the lecture, we present a three-dimensional model for the simulation of signal processing in neurons. Part of this approach is a method to reconstruct the geometric structure of neurons from data measured by 2-photon microscopy. Being able to reconstruct neural geometries and network connectivities from measured data is the basis of understanding coding of motoric perceptions and long term plasticity which is one of the main topics of neuroscience. Other issues are compartment models and upscaling.
Overview
Abstract
The crucial feature of neuronal ensembles is their high complexity and variability. This makes modeling and computation very difficult, in particular for detailed models based on first principles. The problem starts with modeling geometry, which has to extract the essential features from those highly complex and variable phenotypes and at the same time has to take in to account the stochastic variability. Moreover, models of the highly complex processes which are living on these geometries are far from being well established, since those are highly complex too and a couple on a hierarchy of scales in space and time. Simulating such systems always puts the whole approach to test, including modeling, numerical methods and software implementations. In combination with validation based on experimental data, all components have to be enhanced to reach a reliable solving strategy.
To handle problems of this complexity, new mathematical methods and software tools are required. In recent years, new approaches such as parallel adaptive multigrid methods and corresponding software tools have been developed allowing to treat problems of huge complexity. In the lecture, we present a three-dimensional model for the simulation of signal processing in neurons. Part of this approach is a method to reconstruct the geometric structure of neurons from data measured by 2-photon microscopy. Being able to reconstruct neural geometries and network connectivities from measured data is the basis of understanding coding of motoric perceptions and long term plasticity which is one of the main topics of neuroscience. Other issues are compartment models and upscaling.
Brief Biography
Dr. Gabriel Wittum is a Professor of Applied Mathematics and Computational Science and a member of the Extreme Computing Research Center. He holds a Ph.D. in Applied Mathematics, at the University of Karlsruhe (1987) and a Postdoc at SFB 123, University of Heidelberg (1987 – 1991). Wittum’s scientific interests lie in developing software architectures, fast solvers for large systems of equations, multiscale numerics and homogenization, discretization, inverse modeling, and optimization enabling numerical methods for high dimensional problems. His work also focuses on numeric geometry and visualization. His research contributes to advance in Medicine and Neurosciences as well as Economics and Environmental Sciences.