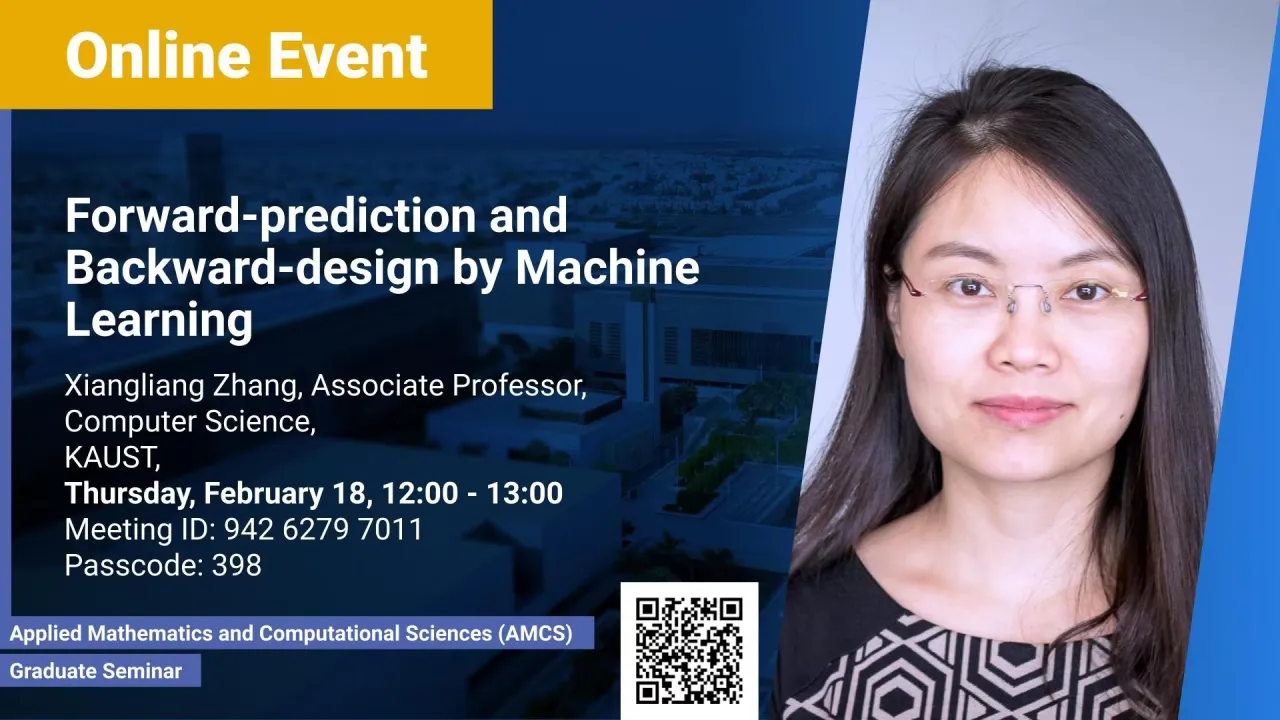
Forward-prediction and Backward-design by Machine Learning
- Prof. Xiangliang Zhang
KAUST
Machine learning has been widely applied to diverse problems of forwarding prediction and backward design. The former problem of forwarding prediction is to predict the reaction of a system given the input x, i.e., y=f(x). This talk will introduce several groups of algorithms for learning the prediction function f. The backward design is an inverse problem, predicting the input x according to the system reaction y, i.e., x=g(y). This is an important problem for the design of chemical material and optical devices. This talk will introduce several successful application examples of machine learning algorithms on the backward design problems.
Overview
Abstract
Machine learning has been widely applied to diverse problems of forwarding prediction and backward design. The former problem of forwarding prediction is to predict the reaction of a system given the input x, i.e., y=f(x). This talk will introduce several groups of algorithms for learning the prediction function f. The backward design is an inverse problem, predicting the input x according to the system reaction y, i.e., x=g(y). This is an important problem for the design of chemical material and optical devices. This talk will introduce several successful application examples of machine learning algorithms on the backward design problems.
Brief Biography
Xiangliang Zhang is an Associate Professor of Computer Science and directs the MINE (http://mine.kaust.edu.sa) group at KAUST, Saudi Arabia. She earned her Ph.D. degree in computer science from INRIA-University Paris-Sud, France, in July 2010. Dr. Zhang's research mainly focuses on learning from complex and large-scale streaming data and graph data. Dr. Zhang has published over 150 research papers in refereed international journals and conference proceedings, including TKDE, SIGKDD, AAAI, IJCAI, NeurIPS, ICDM, etc. Dr. Zhang was invited to deliver an Early Career Spotlight talk at IJCAI-ECAI 2018.