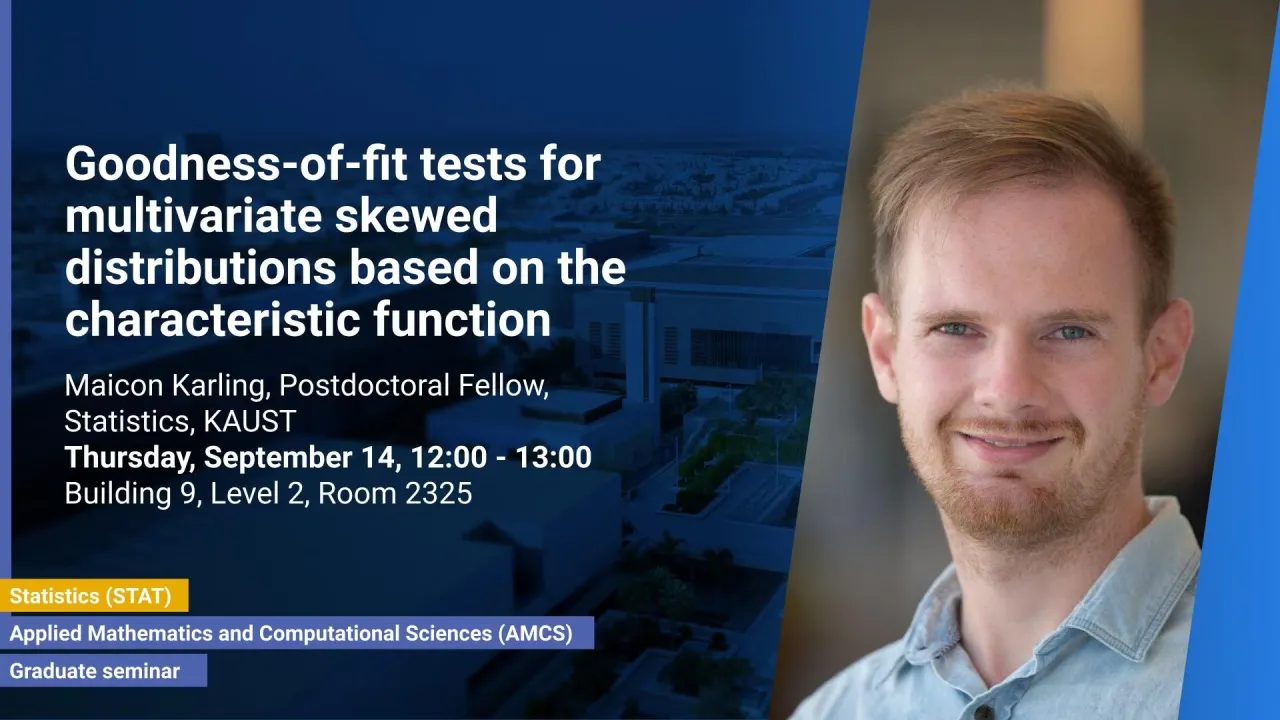
Goodness-of-fit tests for multivariate skewed distributions based on the characteristic function
Goodness-of-fit tests determine how well a set of observed data fits a particular probability distribution. They can also show if some categorical variable follows a hypothesized family of distributions.
Overview
Abstract
Goodness-of-fit tests determine how well a set of observed data fits a particular probability distribution. They can also show if some categorical variable follows a hypothesized family of distributions. Not surprisingly, testing for multivariate normality occupies a prominent place in this setting as a wide range of procedures is available, including characteristic function-based tests. Outside the multivariate Gaussian context, however, the range of goodness-of-fit procedures is limited to only a handful of distributions, most belonging to the elliptical class. In that connection, the proposed seminar will focus on a general Monte Carlo method to test composite hypotheses of goodness-of-fit for several types of multivariate distributions that can accommodate asymmetry and heavy tails. Specifically, we consider weighted L2-type tests based on a discrepancy measure involving the distance between empirical characteristic functions and thus avoid the need for employing corresponding population quantities which may be unknown or complicated to work with. The only requirements of our tests are that we should be able to draw samples from the distribution under test and possess a reasonable estimation method of the unknown distributional parameters. Monte Carlo studies will be presented to show the performance of the test criteria in finite samples for several families of skewed distributions. Real-data examples will also be included to illustrate our method.
Brief Biography
Maicon J. Karling is a Postdoctoral Fellow working in Prof. Marc Genton's Spatio-Temporal Statistics & Data Science Research Group. His current research focuses on multivariate skewed distributions, statistical inference, time series, and Bayesian models.