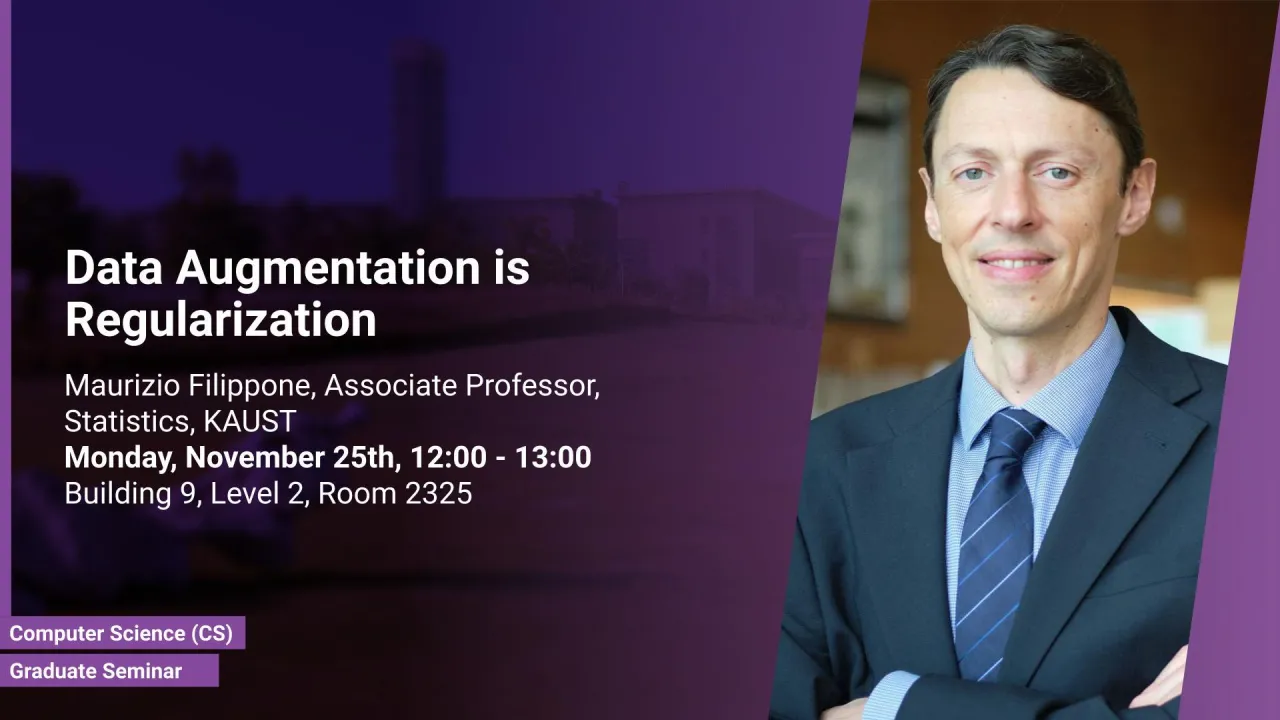
Data Augmentation is Regularization
Data augmentation is a popular technique to considerably improve training of neural networks in various applications, such as computer vision and language modeling.
Overview
Abstract
Data augmentation is a popular technique to considerably improve training of neural networks in various applications, such as computer vision and language modeling. But what makes data augmentation so powerful? In this talk, I will consider one approach to explain the success behind data augmentation by establishing a formal connection with regularization. In particular, it is possible to prove that in linear regression, optimizing the square loss under data augmentation through the addition of Gaussian noise to the inputs yields the regularized least-square solution. After discussing ways in which this can be extended to nonlinear models, I will conclude with some illustrations on supervised and unsupervised computer vision problems, such as classification and generative modeling.
Brief Biography
Maurizio Filippone received a Master's degree in Physics and a Ph.D. in Computer Science from the University of Genova, Italy, in 2004 and 2008, respectively.In 2007, during his Ph.D. studies, he visited George Mason University, Fairfax, VA as a Research Scholar for about eight months. From 2008 to 2011, he was a Research Associate with the University of Sheffield, U.K. (2008-2009), with the University of Glasgow, U.K. (2010), and with University College London, U.K (2011). In 2011, he took up a Lecturer position at the University of Glasgow, U.K, which he left in 2015 to join EURECOM, Sophia Antipolis, France as an Associate Professor. In 2024 he joined the Statistics Program at KAUST as an Associate Professor.His current research interests include the development of tractable and scalable Bayesian inference techniques for Gaussian processes and Deep Learning models with applications in life and environmental sciences.