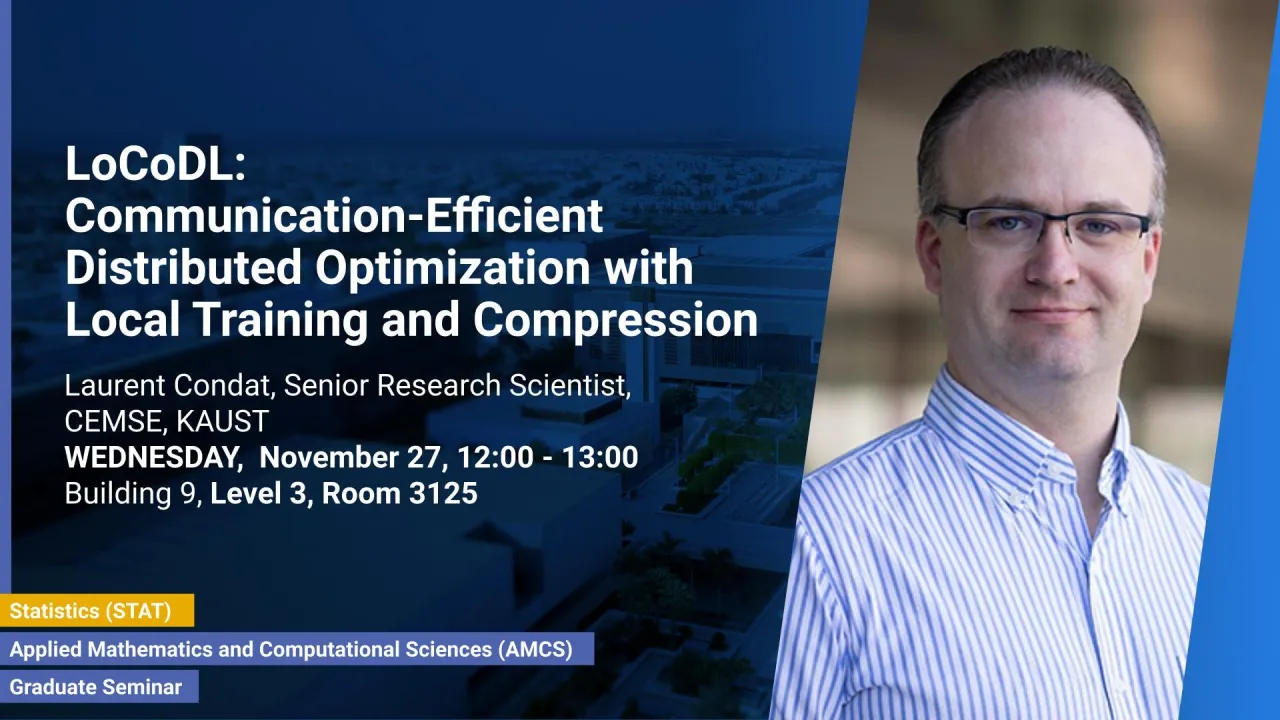
LoCoDL: Communication-Efficient Distributed Optimization with Local Training and Compression
In distributed optimization, and even more in federated learning, communication is the main bottleneck. We introduce LoCoDL, a communication-efficient algorithm that leverages the two techniques of Local training, which reduces the communication frequency, and Compression with a large class of unbiased compressors that includes sparsification and quantization strategies.
Overview
Abstract
In distributed optimization, and even more in federated learning, communication is the main bottleneck. We introduce LoCoDL, a communication-efficient algorithm that leverages the two techniques of Local training, which reduces the communication frequency, and Compression with a large class of unbiased compressors that includes sparsification and quantization strategies. LoCoDL provably benefits from the two mechanisms and enjoys a doubly-accelerated communication complexity, with respect to the condition number of the functions and the model dimension, in the general heterogenous regime with strongly convex functions.
Brief Biography
I got my PhD in 2006 from Grenoble Inst. of Tech., Grenoble, France. After 2 years as a postdoc in Munich, Germany, I was recruited as a permanent researcher by the CNRS in 2008. I spent 4 years in the GREYC, Caen, and 7 years in GIPSA-Lab, Grenoble. From 2016 to 2019, I was a member of the French National Committee for Scientific Research (CoNRS, Section 7). Since Nov. 2019, I am on leave from the CNRS and a senior researcher at KAUST.