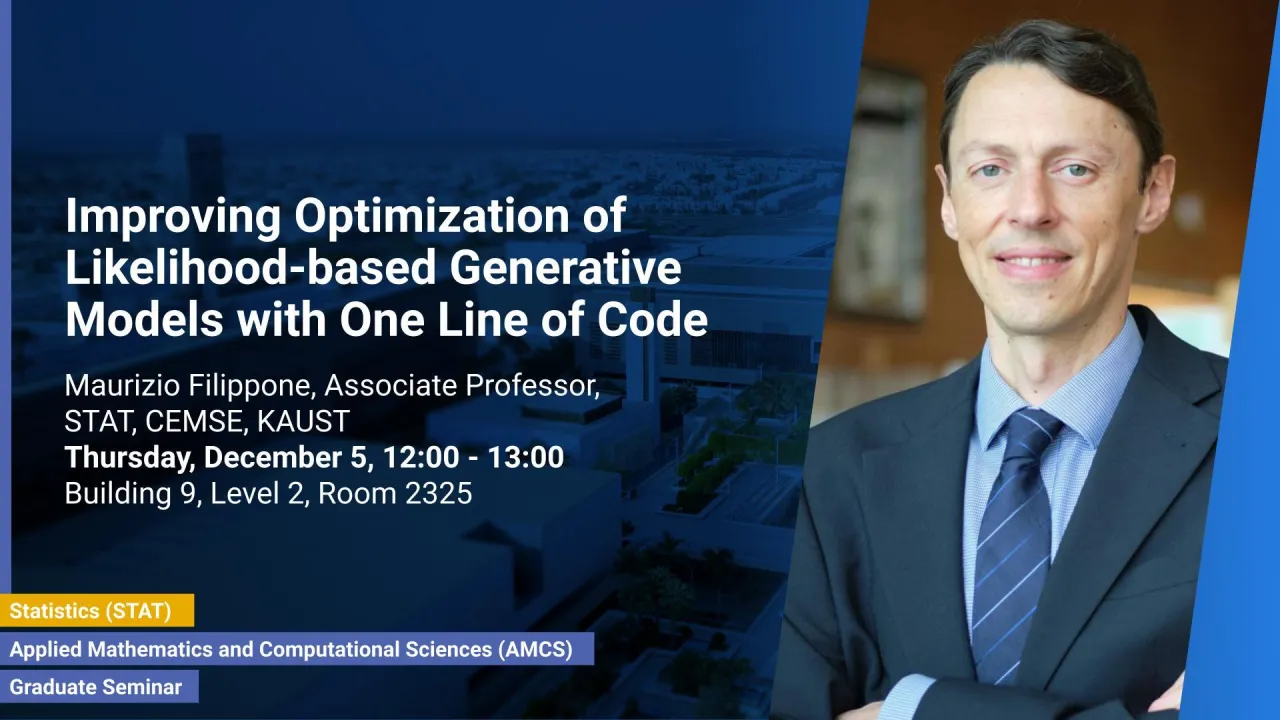
Improving Optimization of Likelihood-based Generative Models with One Line of Code
Generative Models (GMs) have attracted considerable attention due to their tremendous success in various domains, such as computer vision where they are capable to generate impressive realistic-looking images.
Overview
Abstract
Generative Models (GMs) have attracted considerable attention due to their tremendous success in various domains, such as computer vision where they are capable to generate impressive realistic-looking images. Likelihood-based GMs are attractive due to the possibility to generate new data by a single model evaluation. However, they typically achieve lower sample quality compared to state-of-the-art score-based diffusion models (DMs). This paper provides a significant step in the direction of addressing this limitation. The idea is to borrow one of the strengths of score-based DMs, which is the ability to perform accurate density estimation in low-density regions and to address manifold overfitting by means of data mollification. We propose a view of data mollification within likelihood-based GMs as a continuation method, whereby the optimization objective smoothly transitions from simple-to-optimize to the original target. Crucially, data mollification can be implemented by adding one line of code in the optimization loop, and I will show that this provides a boost in generation quality of likelihood-based GMs, without computational overheads. I will then present results on real-world image data sets and UCI benchmarks with popular likelihood-based GMs, including variants of variational autoencoders and normalizing flows, showing large improvements in FID score and density estimation. I will conclude my talk presenting some recent results on supervised learning tasks and some thoughts on a probabilistic interpretation of data mollification.
Brief Biography
Maurizio Filippone received a Master's degree in Physics and a Ph.D. in Computer Science from the University of Genova, Italy, in 2004 and 2008, respectively.
In 2007, during his Ph.D. studies, he visited George Mason University, Fairfax, VA as a Research Scholar for about eight months. From 2008 to 2011, he was a Research Associate with the University of Sheffield, U.K. (2008-2009), with the University of Glasgow, U.K. (2010), and with University College London, U.K (2011). In 2011, he took up a Lecturer position at the University of Glasgow, U.K, which he left in 2015 to join EURECOM, Sophia Antipolis, France as an Associate Professor. In 2024 he joined the Statistics Program at KAUST as an Associate Professor.
His current research interests include the development of tractable and scalable Bayesian inference techniques for Gaussian processes and Deep Learning models with applications in life and environmental sciences.