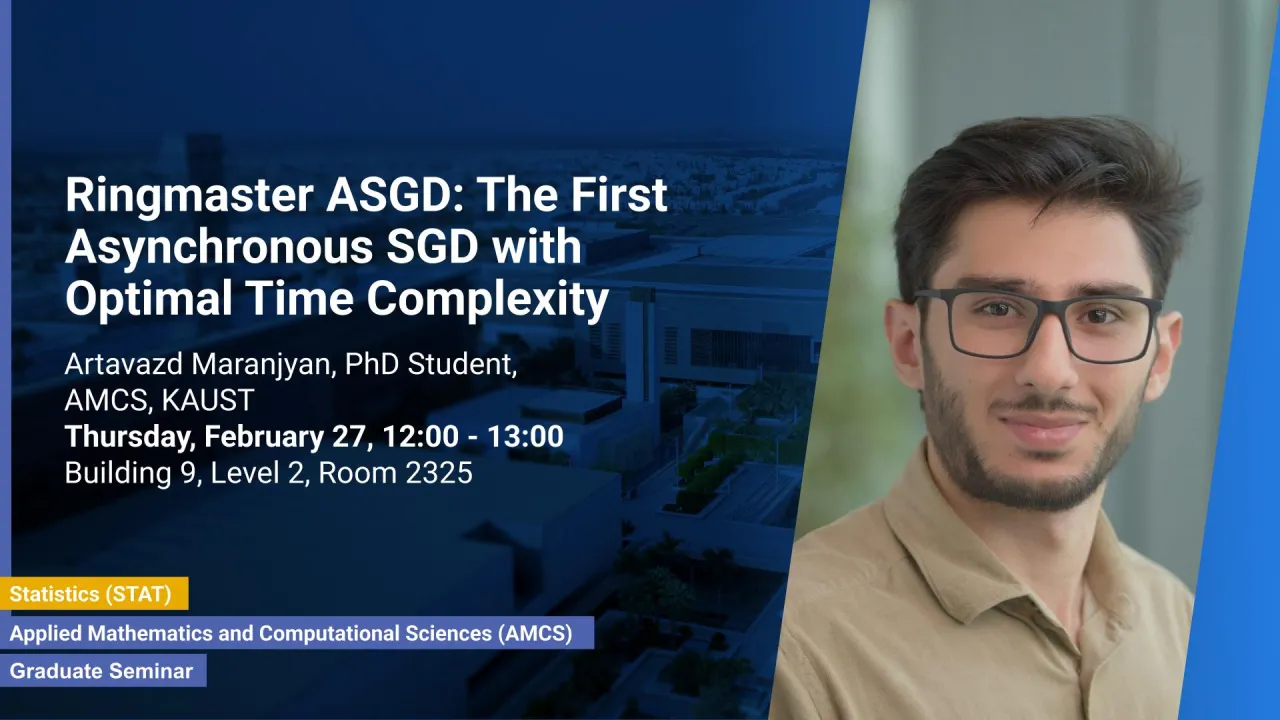
Ringmaster ASGD: The First Asynchronous SGD with Optimal Time Complexity
B9, L2, R2325
Asynchronous Stochastic Gradient Descent (Asynchronous SGD) is a cornerstone method for parallelizing learning in distributed machine learning. However, its performance suffers under arbitrarily heterogeneous computation times across workers, leading to suboptimal time complexity and inefficiency as the number of workers scales.
Overview
While several Asynchronous SGD variants have been proposed, recent findings by Tyurin & Richtárik (NeurIPS 2023) reveal that none achieve optimal time complexity, leaving a significant gap in the literature. In this paper, we propose Ringmaster ASGD, a novel Asynchronous SGD method designed to address these limitations and tame the inherent challenges of Asynchronous SGD. We establish, through rigorous theoretical analysis, that Ringmaster ASGD achieves optimal time complexity under arbitrarily heterogeneous and dynamically fluctuating worker computation times. This makes it the first Asynchronous SGD method to meet the theoretical lower bounds for time complexity in such scenarios.
Presenters
Artavazd Maranjyan, PhD Student, AMCS, KAUST
Brief Biography
Artavazd Maranjyan is a second-year Ph.D. student at KAUST, advised by Prof. Peter Richtárik. His research focuses on optimization for machine learning (ML) and federated learning (FL), contributing to the development of distributed and randomized optimization algorithms. His current work addresses system heterogeneity issues in distributed ML and FL, with an emphasis on asynchronous methods.Before starting his Ph.D., he earned an MSc and BSc from Yerevan State University. During his bachelor's studies, he co-authored several papers in Harmonic Analysis under the guidance of Prof. Martin Grigoryan.