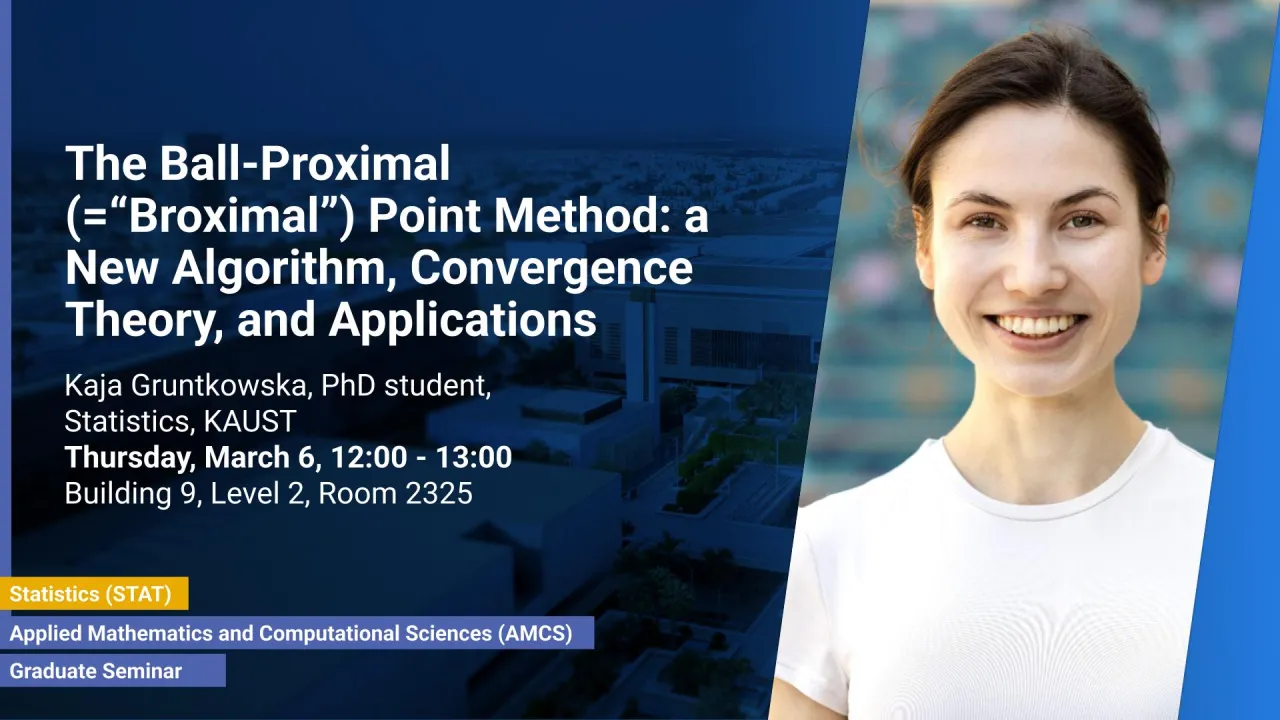
The Ball-Proximal (=“Broximal”) Point Method: a New Algorithm, Convergence Theory, and Applications
B9, L2, R2325
Non-smooth and non-convex global optimization poses significant challenges across various appli- cations, where standard gradient-based methods often struggle. We propose the Ball-Proximal Point Method, Broximal Point Method, or Ball Point Method (BPM) for short – a novel algorith- mic framework inspired by the classical Proximal Point Method (PPM) (Rockafellar, 1976), which, as we show, sheds new light on several founda- tional optimization paradigms and phenomena, including non-convex and non-smooth optimiza- tion, acceleration, smoothing, adaptive stepsize selection, and trust-region methods.
Overview
At the core of BPM lies the ball-proximal (“broximal”) operator, which arises from the classical proximal opera- tor by replacing the quadratic distance penalty by a ball constraint. Surprisingly, and in sharp contrast with the sublinear rate of PPM in the nonsmooth convex regime, we prove that BPM converges linearly and in a finite number of steps in the same regime. Furthermore, by introducing the concept of ball-convexity, we prove that BPM retains the same global convergence guarantees under weaker assumptions, making it a powerful tool for a broader class of potentially non-convex optimization problems. Just like PPM plays the role of a conceptual method inspiring the devel- opment of practically efficient algorithms and al- gorithmic elements, e.g., gradient descent, adap- tive step sizes, acceleration (Ahn & Sra, 2020), and “W” in AdamW (Zhuang et al., 2022), we be- lieve that BPM should be understood in the same manner: as a blueprint and inspiration for further development.
Presenters
Kaja Gruntkowska, PhD student, Statistics, KAUST
Brief Biography
Kaja Gruntkowska is a PhD student in the Statistics department at KAUST, advised by Prof. Peter Richtárik. Her research focuses on developing the algorithmic and mathematical foundations of randomized optimization, with a particular emphasis on distributed computing. She works on designing practically motivated algorithms with provable convergence guarantees, bridging theory and real-world applications to advance scalable machine learning. She completed her Bachelor's in Mathematics and Statistics at the University of Warwick in 2022 and earned a Master's in Statistical Science from the University of Oxford in 2023.