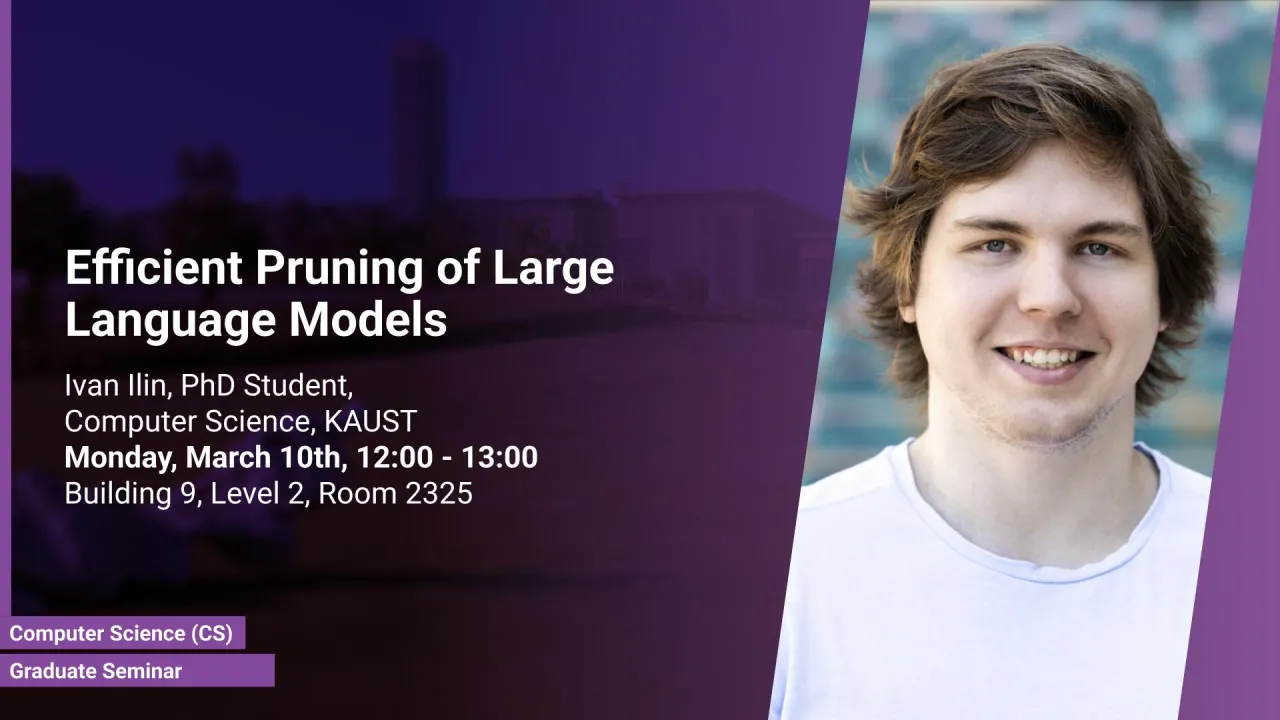
Efficient Pruning of Large Language Models
Thanos, a novel weight-pruning algorithm, efficiently reduces the size and improves performance of large language models by removing redundant weights using a block-wise, adaptively masked strategy that supports flexible sparsity patterns and achieves state-of-the-art results.
Overview
I will present Thanos, a novel weight-pruning algorithm designed to reduce the memory footprint and enhance the computational efficiency of large language models (LLMs) by removing redundant weights while maintaining accuracy. Thanos introduces a block-wise pruning strategy with adaptive masks that dynamically adjust to weight importance, enabling flexible sparsity patterns and structured formats, such as $n:m$ sparsity, optimized for hardware acceleration. Experimental evaluations demonstrate that Thanos achieves state-of-the-art performance in structured pruning and outperforms existing methods in unstructured pruning. By providing an efficient and adaptable approach to model compression, Thanos offers a practical solution for deploying large models in resource-constrained environments. The algorithm is publicly available for further research and application.
Presenters
Brief Biography
Started his academic path at Specialized Educational Scientific Center of NSU, Russia in 2015, then finished B.S. in Automation of Physical and Technical Research at Novosibirsk State University from 2017–2021, and now doing research in King Abdullah University of Science and Technology, Saudi Arabia while MS and PhD.
Besides his academic work he is an author of "Vectozavr" Youtube channel where he presented his research and some fun projects.
In 2021 he created an online school - vectozavr.ru of physics and math for game developers.