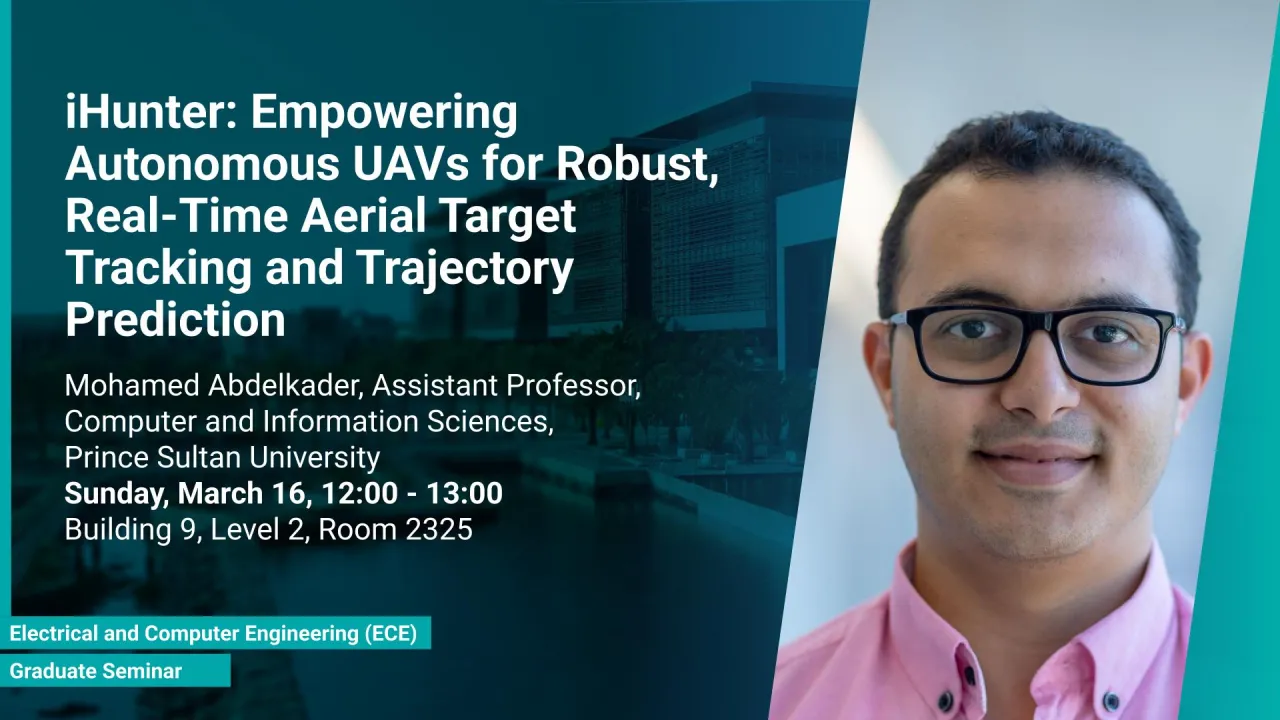
iHunter: Empowering Autonomous UAVs for Robust, Real-Time Aerial Terget Tracking and Trajectory Prediction
This talk introduces iHunter, an integrated framework for UAVs to reliably detect, track, and predict the trajectories of moving targets by fusing multi-modal perception with state estimation, employing kinematic predictors and a GRU-based neural network trained on synthetic data, and demonstrating improved tracking stability and predictive performance for aerial surveillance and target interception.
Overview
Autonomous aerial systems are rapidly transforming surveillance, security, and search–rescue operations—but achieving reliable, real-time detection and tracking of agile aerial targets remains challenging. In this talk, I introduce iHunter, an integrated framework that empowers UAVs to robustly detect, track, and forecast the trajectories of moving targets by addressing two critical challenges: resilient object detection and precise trajectory prediction.
To mitigate intermittent misdetections by primary vision-based systems, we developed our SMART TRACK framework, which fuses multi-modal perception with high-frequency state estimation. By leveraging Kalman filter as feedback to rapidly re-detect targets, SMART TRACK attains sub-meter tracking accuracy. For forecasting agile trajectories, we propose two approaches: an online selection of simple kinematic predictors and a GRU-based neural network for rapid velocity prediction—both validated through simulation and field experiments. Complementing these advances is our FLIGHTGEN framework, which automatically generates large-scale synthetic UAV trajectories using realistic simulations. Remarkably, the GRU model trained solely on this synthetic dataset provided accurate predictions in real experiments with unseen target trajectories, underscoring the effectiveness of our sim2real approach. Together, these innovations dramatically enhance tracking stability and predictive performance, offering a comprehensive solution for aerial surveillance and dynamic target interception.
Presenters
Mohamed Abdelkader, Assistant Professor, Computer and Information Sciences, Prince Sultan University
Brief Biography
Dr. Mohamed Abdelkader is an Assistant Professor in the College of Computer and Information Sciences (CCIS) at Prince Sultan University (PSU) in Riyadh, Saudi Arabia. He is also the lead of the robotics team at the Robotics & Internet of Things (RIOTU) research lab at PSU, where he leads multiple research projects in UAVs, autonomous navigation, and robotic systems. Dr. Abdelkader received his Ph.D. in Mechanical Engineering from King Abdullah University of Science and Technology (KAUST) in 2018, where his research focused on real-time distributed planning in multi-robot systems. His expertise spans SLAM, AI-based GPS-denied localization, UAV control, and multi-robot coordination. Throughout his career, he has been involved in cutting-edge research and development, including UAV swarm intelligence, autonomous perching drones for inspection, and real-time motion planning. He has contributed to 10+ US patents related to innovative robotic inspection and UAV technologies. Dr. Abdelkader has also been actively involved in academic and industrial collaborations, securing multiple research grants, and leading UAV-based projects such as autonomous target tracking, VTOL UAVs, and robotic delivery systems. His work has been recognized through several awards, including achievements in international robotics competitions.