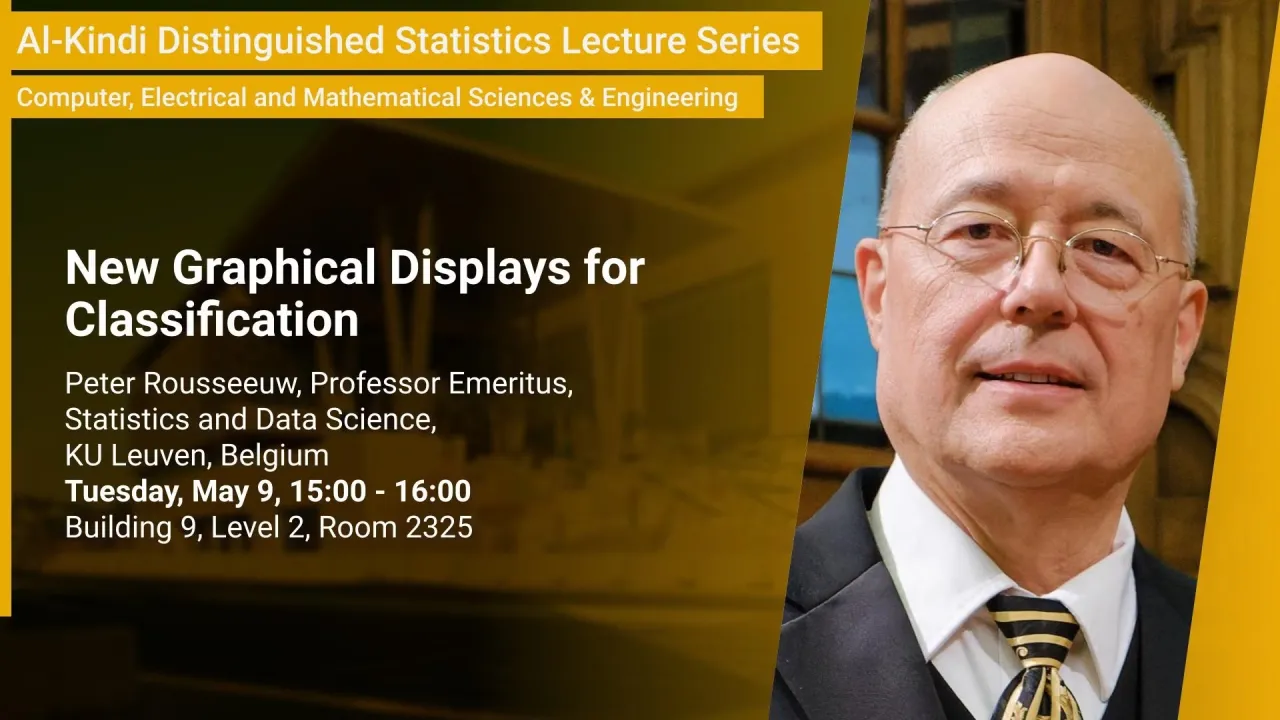
New Graphical Displays for Classification
- Peter Rousseeuw, Professor Emeritus, Statistics and Data Science, KU Leuven, Belgium
B9 L2 H2
Classification is a major tool of statistics and machine learning. Several classifiers have interesting visualizations of their inner workings. Here we pursue a different goal, which is to visualize the cases being classified, either in training data or in test data. An important aspect is whether a case has been classified to its given class (label) or whether the classifier wants to assign it to a different class. This is reflected in the probability of the alternative class (PAC). A high PAC indicates label bias, i.e. the possibility that the case was mislabeled. The PAC is used to construct a silhouette plot which is similar in spirit to the silhouette plot for cluster analysis. The average silhouette width can be used to compare different classifications of the same dataset. We will also draw quasi residual plots of the PAC versus a data feature, which may lead to more insight in the data. One of these data features is how far each case lies from its given class, yielding so-called class maps. The proposed displays are constructed for discriminant analysis, k-nearest neighbors, support vector machines, CART, random forests, and neural networks. The graphical displays are illustrated and interpreted on data sets containing images, mixed features, and texts.
Overview
Abstract
Classification is a major tool of statistics and machine learning. Several classifiers have interesting visualizations of their inner workings. Here we pursue a different goal, which is to visualize the cases being classified, either in training data or in test data. An important aspect is whether a case has been classified to its given class (label) or whether the classifier wants to assign it to a different class. This is reflected in the probability of the alternative class (PAC). A high PAC indicates label bias, i.e. the possibility that the case was mislabeled. The PAC is used to construct a silhouette plot which is similar in spirit to the silhouette plot for cluster analysis. The average silhouette width can be used to compare different classifications of the same dataset. We will also draw quasi residual plots of the PAC versus a data feature, which may lead to more insight in the data. One of these data features is how far each case lies from its given class, yielding so-called class maps. The proposed displays are constructed for discriminant analysis, k-nearest neighbors, support vector machines, CART, random forests, and neural networks. The graphical displays are illustrated and interpreted on data sets containing images, mixed features, and texts.
Brief Biography
Peter Rousseeuw is a Belgian statistician who has mainly contributed to robust methodology, outlier detection, and cluster analysis. He obtained his PhD following research at ETH Zurich, which led to a book on influence functions. Later he was professor at the Delft University of Technology and at the University of Antwerp, Belgium. Afterward he spent over a decade in a financial company in New York. Currently he is professor emeritus at KU Leuven, Belgium. Among the techniques he introduced are the Least Trimmed Squares method and S-estimators for robust regression, as well as the Minimum Covariance Determinant method for covariance matrices. His 1984 paper on robust regression was included in Breakthroughs in Statistics by Kotz and Johnson, which reprinted 60 papers from 1850 to 1990. With L. Kaufman he coined the word medoid when proposing the k-medoids method for cluster analysis. His silhouette display for clustering is often used to select the number of clusters. Other works are the scale estimator Qn with C. Croux, robust principal component methods, regression depth, the bagplot with J. Tukey, and dealing with cellwise outliers. His work has been cited over 100,000 times.