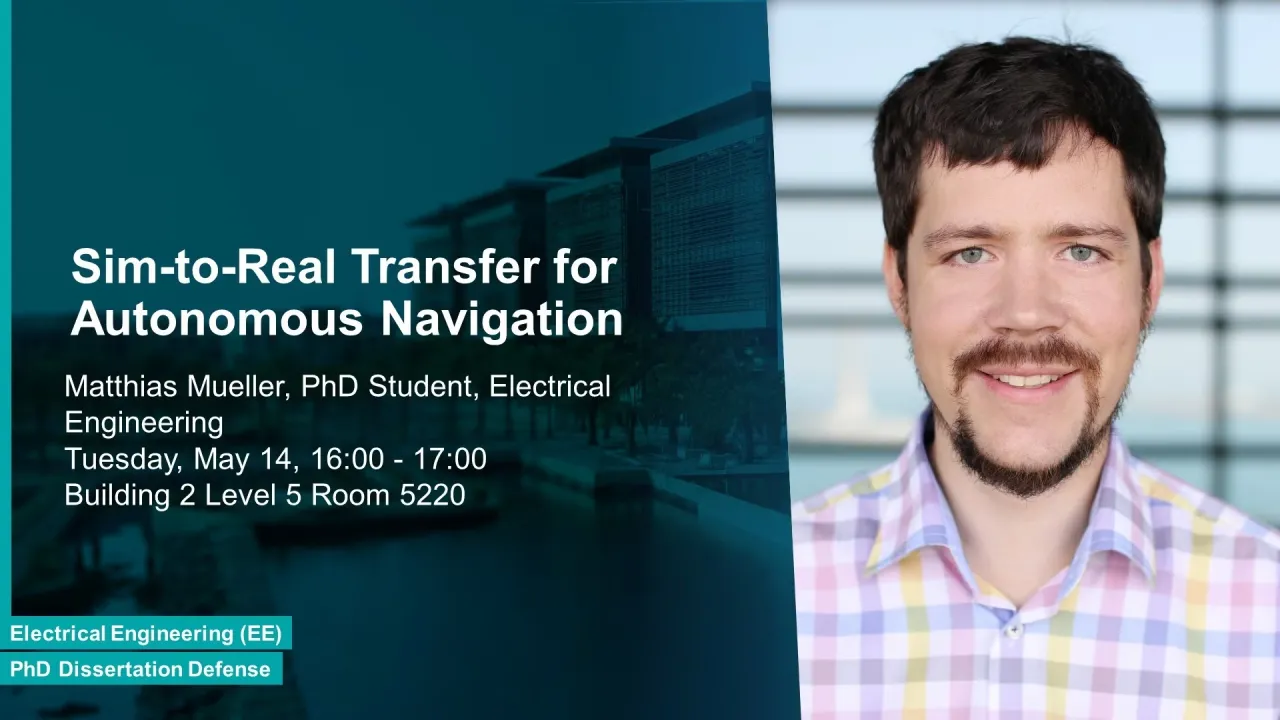
Sim-to-Real Transfer for Autonomous Navigation
This work investigates the problem of transfer from simulation to the real world in the context of autonomous navigation. To this end, we first present a photo-realistic training and evaluation simulator Sim4CV which enables several applications across various fields of computer vision. Built on top of the Unreal Engine, the simulator features cars and unmanned aerial vehicles (UAVs) with a realistic physics simulation and diverse urban and suburban 3D environments. We demonstrate the versatility of the simulator with two case studies: autonomous UAV-based tracking of moving objects and autonomous driving using supervised learning.
Overview
Abstract
This work investigates the problem of transfer from simulation to the real world in the context of autonomous navigation. To this end, we first present a photo-realistic training and evaluation simulator Sim4CV which enables several applications across various fields of computer vision. Built on top of the Unreal Engine, the simulator features cars and unmanned aerial vehicles (UAVs) with a realistic physics simulation and diverse urban and suburban 3D environments. We demonstrate the versatility of the simulator with two case studies: autonomous UAV-based tracking of moving objects and autonomous driving using supervised learning. Using the insights gained from aerial object tracking, we find that current object trackers are either too slow or inaccurate for online tracking from an UAV. In addition, we find that in particular background clutter, fast motion and occlusion are preventing fast trackers such as correlation filter (CF) trackers to perform better. To address this issue we propose a novel and general framework that can be applied to CF trackers in order incorporate context. As a result, the learned filter is more robust to drift due to the aforementioned tracking challenges. We show that our framework can improve several CF trackers by a large margin while maintaining a very high frame rate. For the application of autonomous driving, we train a driving policy that drives very well in simulation. However, while our simulator is photo-realistic there still exists a virtual-reality gap. We show how this gap can be reduced via modularity and abstraction in the driving policy. More specifically, we split the driving task into several modules namely perception, driving policy and control. This simplifies the transfer significantly and we show how a driving policy that was only trained in simulation can be transferred to a robotic vehicle in the physical world directly. Lastly, we investigate the application of UAV racing which has emerged as a modern sport recently. We propose a controller fusion network (CFN) which allows fusing multiple imperfect controllers; the result is a navigation policy that outperforms each one of them. Further, we embed this CFN into a modular network architecture similar to the one for driving, in order to decouple perception and control. We use our photo-realistic simulation environment to demonstrate how navigation policies can be transferred to different environment conditions by this network modularity.
Brief Biography
Matthias graduated with a B.Sc. in Electrical Engineering and Math Minor from Texas A&M University in 2011. After graduation, he joined P+Z Engineering as an Electrical Engineer and worked for 3 years on the development of mild-hybrid electric machines at BMW. In 2014, he started his M.Sc. in Electrical Engineering at KAUST and rolled over into the Ph.D. program in 2016. His research interests lie in the fields of computer vision, robotics and machine learning where it has contributed to more than 10 publications. Matthias has extensive experience in object tracking and autonomous navigation of embodied agents such as cars and UAVs.