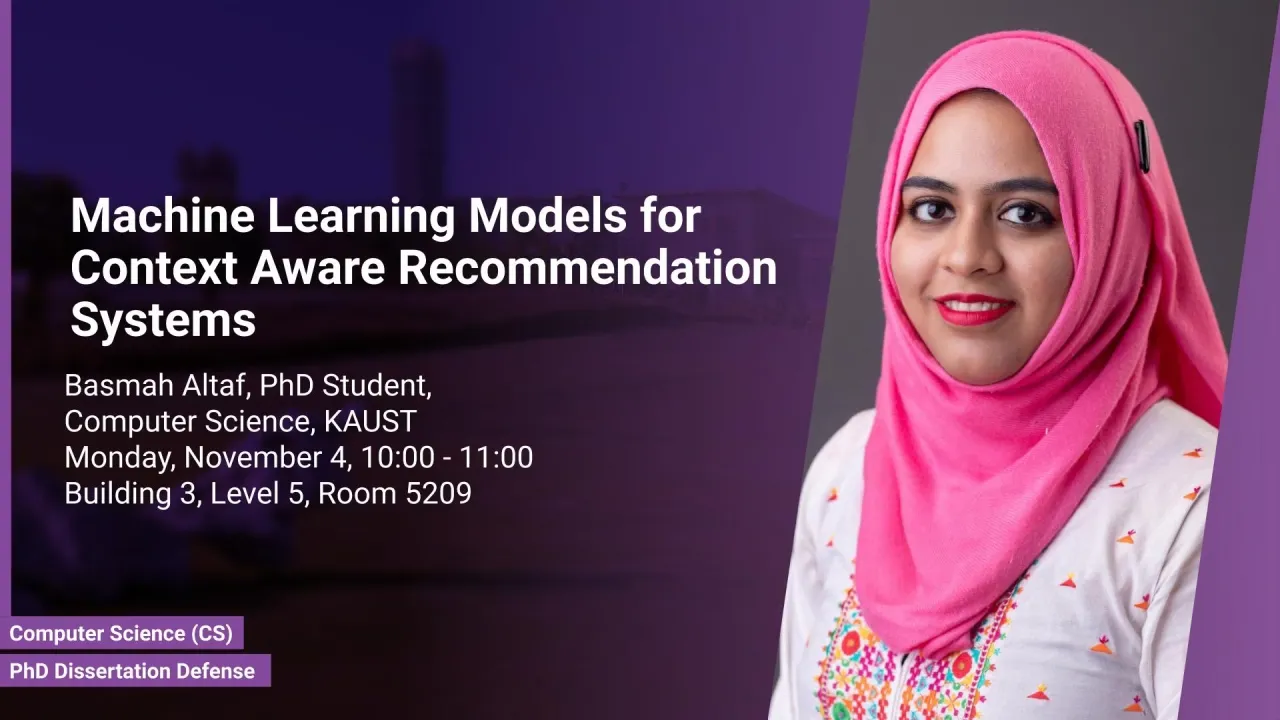
Machine Learning Models for Context Aware Recommendation Systems
The goal of this thesis is to pave the way towards the next generation of recommendation systems tackling such real-world challenges to improve the user experience while giving good recommendations.
Overview
Abstract
Recommender systems are an integral and successful application of machine learning in the e-Commerce industry and in the everyday lives of online users. Similar recommendation algorithms are applied for news, music, books, points of interest, or travel recommendation. Although much focus has been paid on improving recommendation quality, however, some real-world aspects are not considered: How to ensure that top- n recommendations are not biased due to any popularity boosting events, such as awards for movies or songs? How to recommend items to entities by explicitly considering social relationships between them? What is the best way to model sequential recommendation systems as context-aware design, and learning on-the-fly from spatial, temporal and social contexts? Can we recommend items to a group of entities by learning representations of both users and items from their social links and contents?
The goal of this thesis is to pave the way towards the next generation of recommendation systems tackling such real-world challenges to improve the user experience while giving good recommendations. This thesis, bridges techniques from recommendation, contextual constraints, and deep-learning for representation learning by proposing novel techniques to address above real-world problems. We focus on three directions: (1) model the effect of popularity bias over the time on the consumption of items, (2) modeling the contextual constraints on sequential history of users and social links for sequential recommendation, and (3) modeling the social links, and contents of nodes in graph as representation learning problem for interest specified in the form of query recommendation.
Brief Biography
Basmah Altaf received B.E. in Computer and Information Systems from N.E.D. University of Engineering and Technology, Pakistan in 2010 and secured Gold Medal for ranking 1st among 140 candidates. She then joined KAUST for studying Masters in Computer Science in 2012. She is currently pursuing a Ph.D. degree in Computer Science under the supervision of Prof. Xiangliang Zhang in the Machine Learning and Intelligence (MINE) group. During Ph.D., she has co-authored 3 top-tier conference papers and presented her research at various international conferences. Her research interests include designing machine learning models for context-aware recommendations with a focus on different domains. She also has practical experience of working as a Software Engineer intern in TrgTech, Telenor and Survey Legend during 2009-2012.