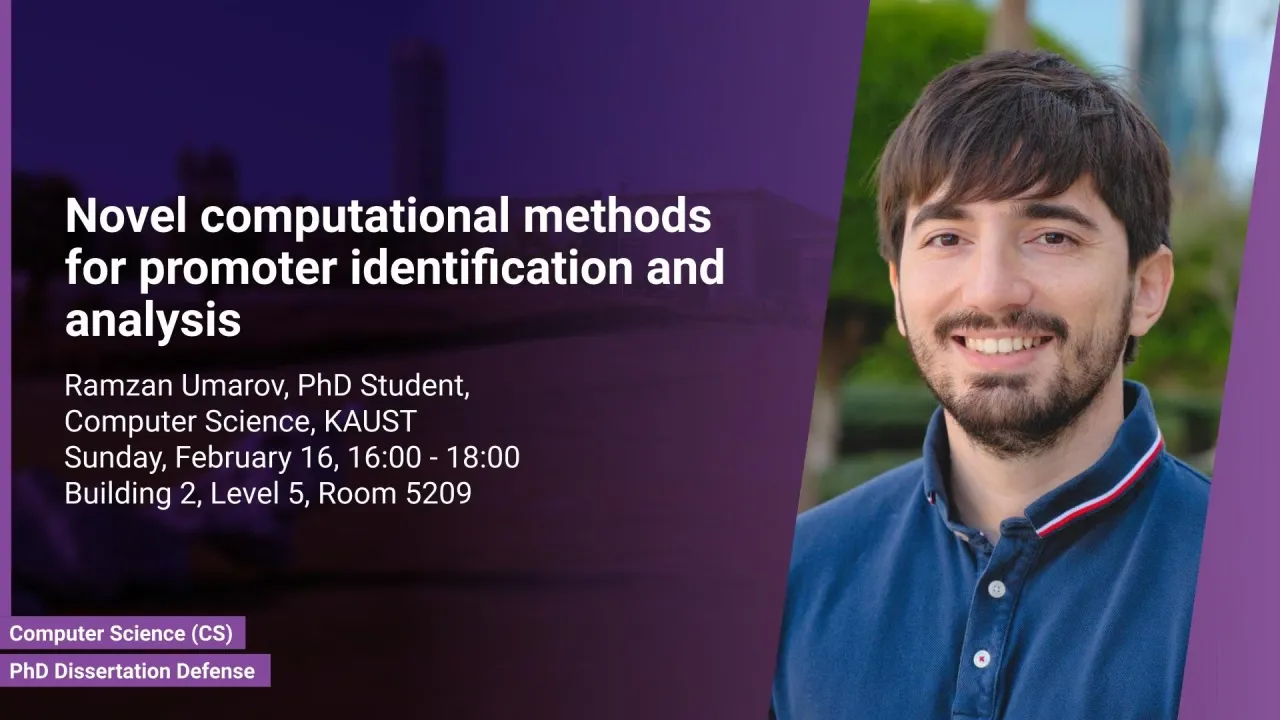
Novel computational methods for promoter identification and analysis
In this dissertation, I present the methods I have developed for prediction of promoters for different organisms. Instead of focusing on the classification accuracy of the discrimination between promoter and non-promoter sequences, I predict the exact positions of the TSS inside the genomic sequences, testing every possible location. The developed methods significantly outperform the previous promoter prediction programs by considerably reducing the number of false positive predictions. Specifically, to reduce the false positive rate, the models are adaptively and iteratively trained by changing the distribution of samples in the training set based on the false positive errors made in the previous iteration. The new methods are used to gain insights into the design principles of the core promoters. Using model analysis, I have identified the most important core promoter elements and their effect on the promoter activity. I have developed a novel general approach to detect long range interactions in the input of a deep learning model, which was used to find related positions inside the promoter region. The final model was applied to the genomes of different species without a significant drop in the performance, demonstrating a high generality of the developed method.
Overview
Abstract
Promoters are key regions that are involved in differential transcription regulation of protein-coding and RNA genes. The gene-specific architecture of promoter sequences makes it extremely difficult to devise a general strategy for their computational identification. Accurate prediction of promoters is fundamental for interpreting gene expression patterns, and for constructing and understanding genetic regulatory networks. In this dissertation, I present the methods I have developed for prediction of promoters for different organisms. Instead of focusing on the classification accuracy of the discrimination between promoter and non-promoter sequences, I predict the exact positions of the TSS inside the genomic sequences, testing every possible location. The developed methods significantly outperform the previous promoter prediction programs by considerably reducing the number of false positive predictions. Specifically, to reduce the false positive rate, the models are adaptively and iteratively trained by changing the distribution of samples in the training set based on the false positive errors made in the previous iteration. The new methods are used to gain insights into the design principles of the core promoters. Using model analysis, I have identified the most important core promoter elements and their effect on the promoter activity. I have developed a novel general approach to detect long range interactions in the input of a deep learning model, which was used to find related positions inside the promoter region. The final model was applied to the genomes of different species without a significant drop in the performance, demonstrating a high generality of the developed method.
Brief Biography
PhD candidate, working on solving biological problems by using applied machine learning with focus on deep learning. He has developed deep learning based methods to solve bioinformatics problems achieving state-of-the-art performance, focusing on various aspects of gene regulation. Mr. Umarov obtained his Master degree from Imperial College London, Advanced Computing course.