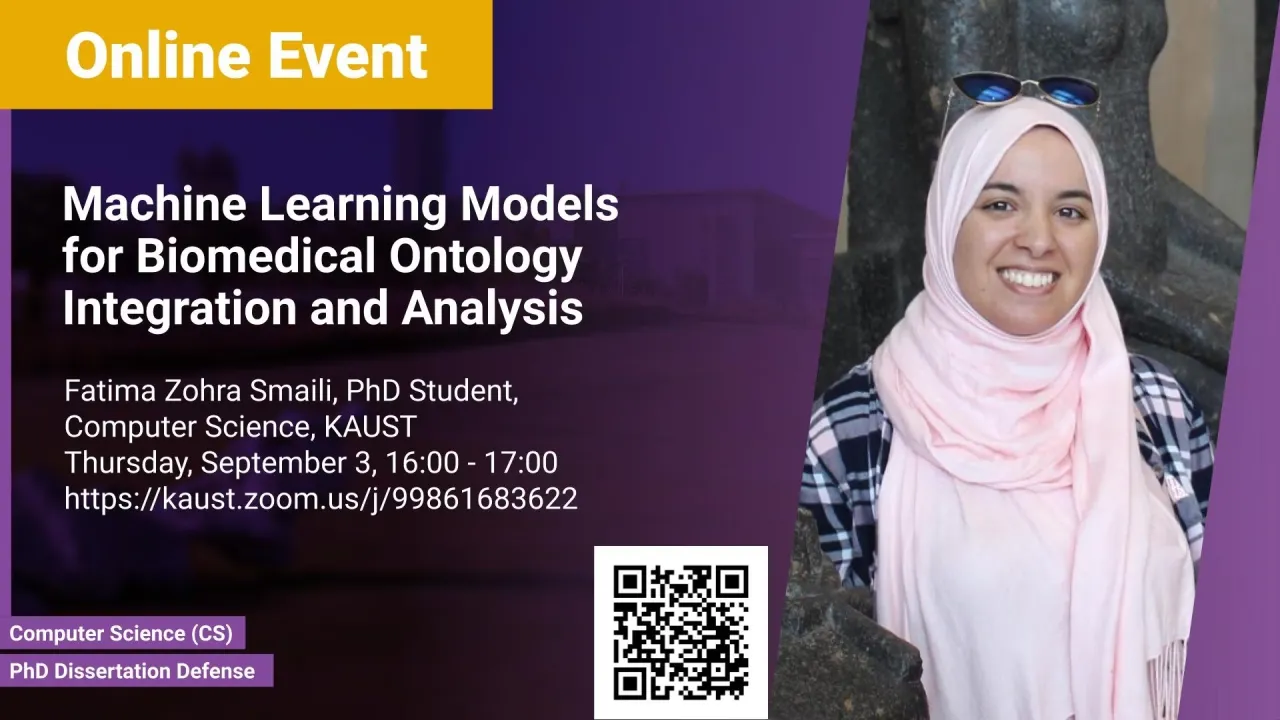
Machine Learning Models for Biomedical Ontology Integration and Analysis
Biological knowledge is widely represented in the form of ontologies and ontology-based annotations. The structure and information contained in ontologies and their annotations make them valuable for use in machine learning, data analysis and knowledge extraction tasks. In this thesis, we propose the first approaches that can exploit all of the information encoded in ontologies, both formal and informal, to learn feature embeddings of biological concepts and biological entities based on their annotations to ontologies by applying transfer learning on the literature. To optimize learning that combines ontologies and natural language data such as the literature, we also propose a new approach that uses self-normalization with a deep Siamese neural network to improve learning from both the formal knowledge within ontologies and textual data. We validate the proposed algorithms by applying them to generate feature representations of proteins, and of genes and diseases.
Overview
Abstract
Biological knowledge is widely represented in the form of ontologies and ontology-based annotations. The structure and information contained in ontologies and their annotations make them valuable for use in machine learning, data analysis and knowledge extraction tasks. In this thesis, we propose the first approaches that can exploit all of the information encoded in ontologies, both formal and informal, to learn feature embeddings of biological concepts and biological entities based on their annotations to ontologies by applying transfer learning on the literature. To optimize learning that combines ontologies and natural language data such as the literature, we also propose a new approach that uses self-normalization with a deep Siamese neural network to improve learning from both the formal knowledge within ontologies and textual data. We validate the proposed algorithms by applying them to generate feature representations of proteins, and of genes and diseases. The generated features are then used in combination with machine learning to perform different prediction tasks including the prediction of protein interactions, gene--disease associations and the toxicological effects of chemicals. The proposed algorithms can be applied to a wide range of other bioinformatics research problems including similarity-based prediction and classification of interaction types using supervised learning, or clustering.
Brief Biography
Fatima Zohra Smaili is a computer science PhD student of Professor Xin Gao. Her research focuses on combining knowledge representation from biomedical ontologies with machine learning to solve biomedical prediction tasks. Prior to her PhD, Fatima Zohra obtained a MSc in CS from KAUST in 2016 and a BSc in CS from Al Akhawayn University in Morocco in 2014.