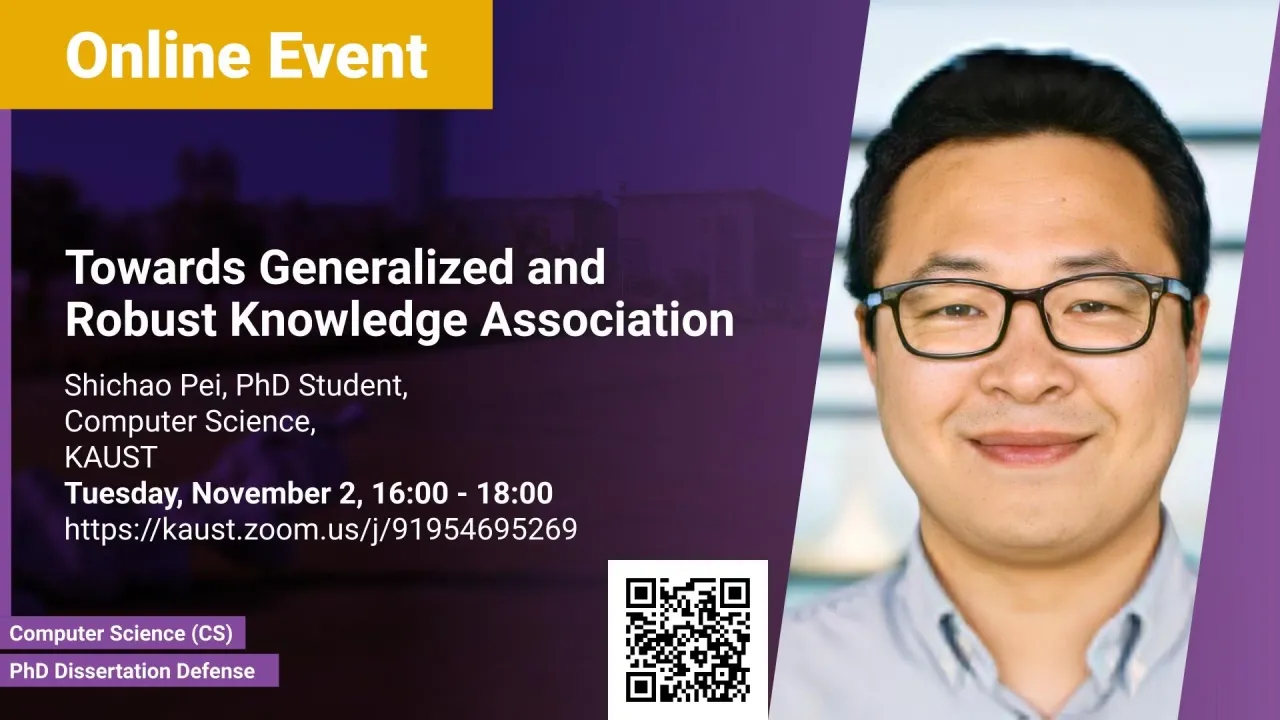
Towards Generalized and Robust Knowledge Association
Human knowledge can facilitate the evolution of artificial intelligence towards learning the capability of planning and reasoning and has been the critical element for developing the next-generation artificial intelligence. Although knowledge collection and organization have achieved significant progress, it is still non-trivial to construct a comprehensive knowledge graph for downstream applications. The difficulty motivates the study of knowledge association to resolve the problem, yet current solutions suffer from two primary shortages, i.e., generalization and robustness. Specifically, most existing methods require a sufficient number of labeled data and ignore the effective utilization of complex relationships between entities, limiting the generalization ability of knowledge association approaches. Moreover, prevailing approaches severely rely on clean labeled data, making the model vulnerable to noises in the given labeled data. These shortages motivate the research on generalization and robustness of knowledge association in this dissertation.
Overview
Abstract
Human knowledge can facilitate the evolution of artificial intelligence towards learning the capability of planning and reasoning and has been the critical element for developing the next-generation artificial intelligence. Although knowledge collection and organization have achieved significant progress, it is still non-trivial to construct a comprehensive knowledge graph for downstream applications. The difficulty motivates the study of knowledge association to resolve the problem, yet current solutions suffer from two primary shortages, i.e., generalization and robustness. Specifically, most existing methods require a sufficient number of labeled data and ignore the effective utilization of complex relationships between entities, limiting the generalization ability of knowledge association approaches. Moreover, prevailing approaches severely rely on clean labeled data, making the model vulnerable to noises in the given labeled data. These shortages motivate the research on generalization and robustness of knowledge association in this dissertation.
In this dissertation, we study two kinds of knowledge association tasks, i.e., entity alignment and entity synonym discovery, and make innovative contributions to address the above drawbacks. First, we design two semi-supervised entity alignment frameworks to enhance the generalization ability by taking advantage of both labeled data with unlabeled data. Second, in order to boost the generalization ability from the perspective of modeling relationships between entities, we propose a set-aware entity synonym discovery model with flexible receptive fields by making a breakthrough in using entity synonym set information to explore and utilize the complex relationship between entities. Third, we propose two robust entity alignment models to enhance robustness by resorting to adversarial learning and non-sampling and curriculum learning, respectively. The experimental results demonstrate the effectiveness of the proposed methods on improving the generalization and robustness of knowledge association.
Brief Biography
Shichao Pei received his B.E. degree in software engineering from Xi'an Jiaotong University, China, in 2016, and the M.S. degree in computer science from KAUST in December 2017. He is a Ph.D. candidate in the computer science department, KAUST, working with Professor Xiangliang Zhang. His research interests broadly lie in data mining and machine learning, in particular graph/network representation learning, knowledge acquisition and integration, weakly supervised learning, and information retrieval, etc.