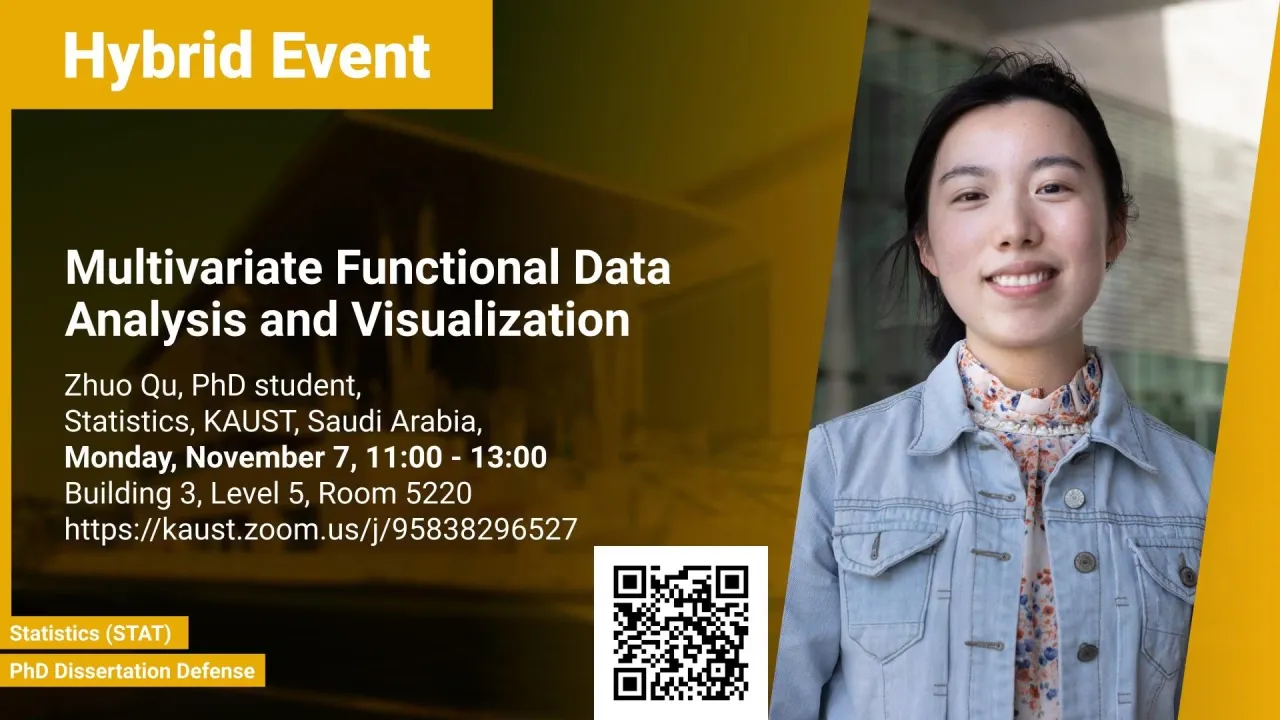
Multivariate Functional Data Analysis and Visualization
As a branch of statistics, functional data analysis studies observations regarded as curves, surfaces, or other objects evolving over a continuum. Current methods in functional data analysis usually require data to be smoothed and analyzed marginally, which may hide some outlier information or take extra time on pretreating the data. After exploring model-based fitting for regularly observed multivariate functional data, we explore new visualization tools, clustering, and multivariate functional depths for irregularly observed (sparse) multivariate functional data.
Overview
Abstract
As a branch of statistics, functional data analysis studies observations regarded as curves, surfaces, or other objects evolving over a continuum. Current methods in functional data analysis usually require data to be smoothed and analyzed marginally, which may hide some outlier information or take extra time on pretreating the data. After exploring model-based fitting for regularly observed multivariate functional data, we explore new visualization tools, clustering, and multivariate functional depths for irregularly observed (sparse) multivariate functional data. The four main chapters that comprise the dissertation are organized as follows. First, median polish for functional multivariate analysis of variance is proposed with the implementation of multivariate functional depths. Second, the sparse functional boxplot and the intensity sparse functional boxplot, as practical exploratory tools that make visualization possible for both complete and sparse functional data, are introduced. Third, a robust two-layer partition clustering of sparse multivariate functional data is introduced. Our clustering method can obtain correct clusters and flag outliers from outlier settings. Lastly, two frameworks for multivariate functional depths based on multivariate depths are proposed. The first framework is the multivariate functional integrated depth, whereas the second one is the multivariate functional extreme depth. In each framework, global and local depth formulas are explored, with theoretical properties being proved and the finite sample depth estimation for irregularly observed multivariate functional data being investigated. In addition, the simplified sparse functional boxplot and simplified intensity sparse functional boxplot for visualization without the need for data reconstruction are introduced. Together, these four extensions to multivariate functional data make them more general and of applicational interest in exploratory multivariate functional data analysis.
Brief Biography
Zhuo Qu has been an MS/Ph.D. student in the Spatio-Temporal Statistics and Data Science Research Group at KAUST since August 2017. She obtained her MS degree in Statistics at KAUST in December 2018, and her Bachelor of Arts degree in Economics and Bachelor of Science degree in Mathematics at China University of Political Science and Law, China in June 2017.