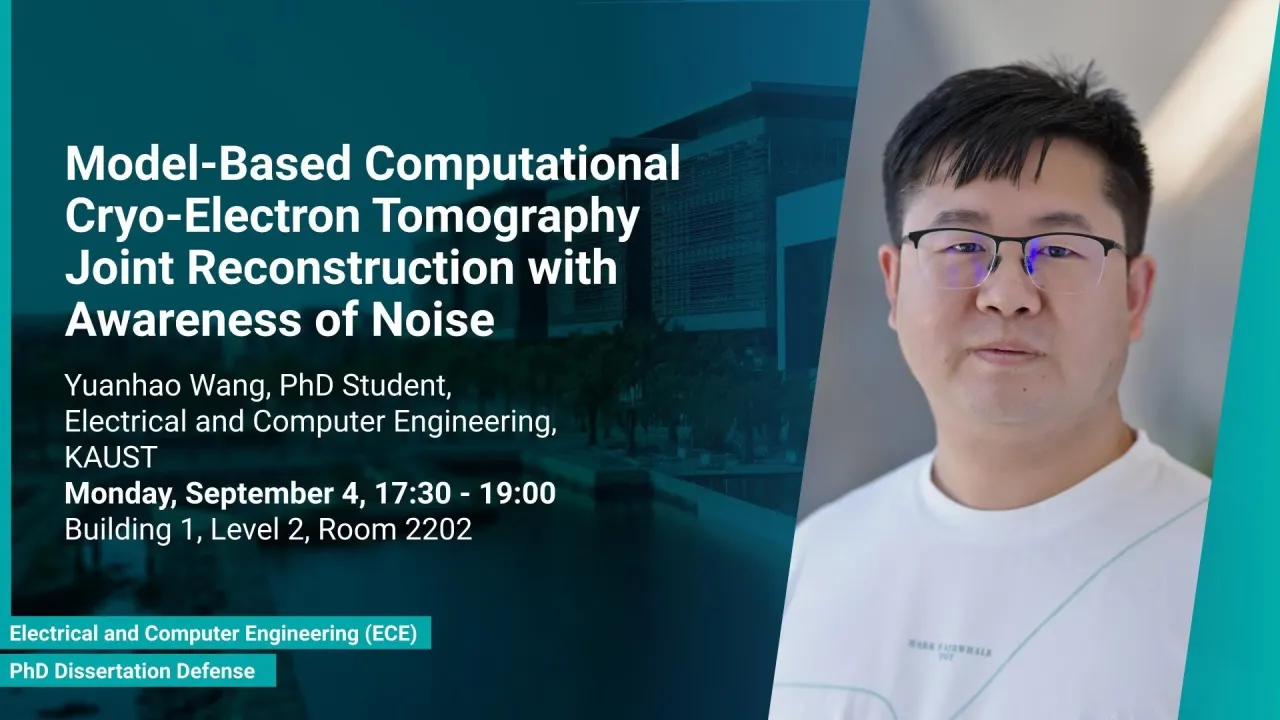
Model-Based Computational Cryo-Electron Tomography Joint Reconstruction with Awareness of Noise
Tilt-series cryo-electron tomography (cryo-ET) is an established imaging tech- nique used in several fields like biology and material science. Despite its success, cryo-ET remains an arduous task. The missing-wedge acquisition, the motion, and the high level noise are the main challenges existing in this field. In this dissertation, we tackle these challenges through the exploration of three distinct approaches: plug and play approach, adaptive differentiable density grids and adaptive tensorial density fields representation.
Overview
Abstract
Tilt-series cryo-electron tomography (cryo-ET) is an established imaging tech- nique used in several fields like biology and material science. Despite its success, cryo-ET remains an arduous task. The missing-wedge acquisition, the motion, and the high level noise are the main challenges existing in this field. In this dissertation, we tackle these challenges through the exploration of three distinct approaches: plug and play approach, adaptive differentiable density grids and adaptive tensorial density fields representation. Firstly, using embedded fiducial markers, our framework first estimates the motion field in the sample in order to correct the captured projections, and then reconstruct the sample using an iterative Plug-and-Play approach. Secondly, we propose an adaptive representation based on an octree structure. Each block of the structure represents a 3D density grid that is optimized from the captured projections. To ensure a denoised output, we update the octree in a multi-scale fashion. We also combined the differentiable image formation model with a cross- nodes non-local constraint, a total variation, and an boundary consistency priors in the loss function. Thirdly, we propose an adaptive tensorial density fields representation. Due to the fact that cyro-EM tomograms is thin along the z-axis, we use a quadtree structure instead of initializing an nulled octree, and represent each node with an vector-matrix factorization. Combined with a total variation, boundary consistency and an isotropic Fourier prior, our framework allow us to reconstruct clean cyro-EM data. Experiments show considerable improvement gradually in Cyro-EM reconstruction.
Brief Biography
Yuanhao Wang is a Ph. D. candidate in the Computational Imaging Group (VCCIMAGING) under the supervision of Professor Wolfgang Heidrich at King Abdullah University of Science and Technology (KAUST).
Yuanhao Wang obtained his bachelor's degree in Communication Engineering from Beijing University of Posts and Telecommunications in China in 2013. In 2016, he received his master's degree in Integrated Circuits Engineering from Tsinghua University in China.
Yuanhao’s research interests encompass a wide range of topics in computational photography, including image processing (ISP), 3D particle tracking velocimetry(PTV), and neural representation for tomography. Currently, he is focusing on applying neural representation to tomography, specifically in the acceleration, reconstruction, and denoising of cryo-electron tomography (cryo-ET) datasets.