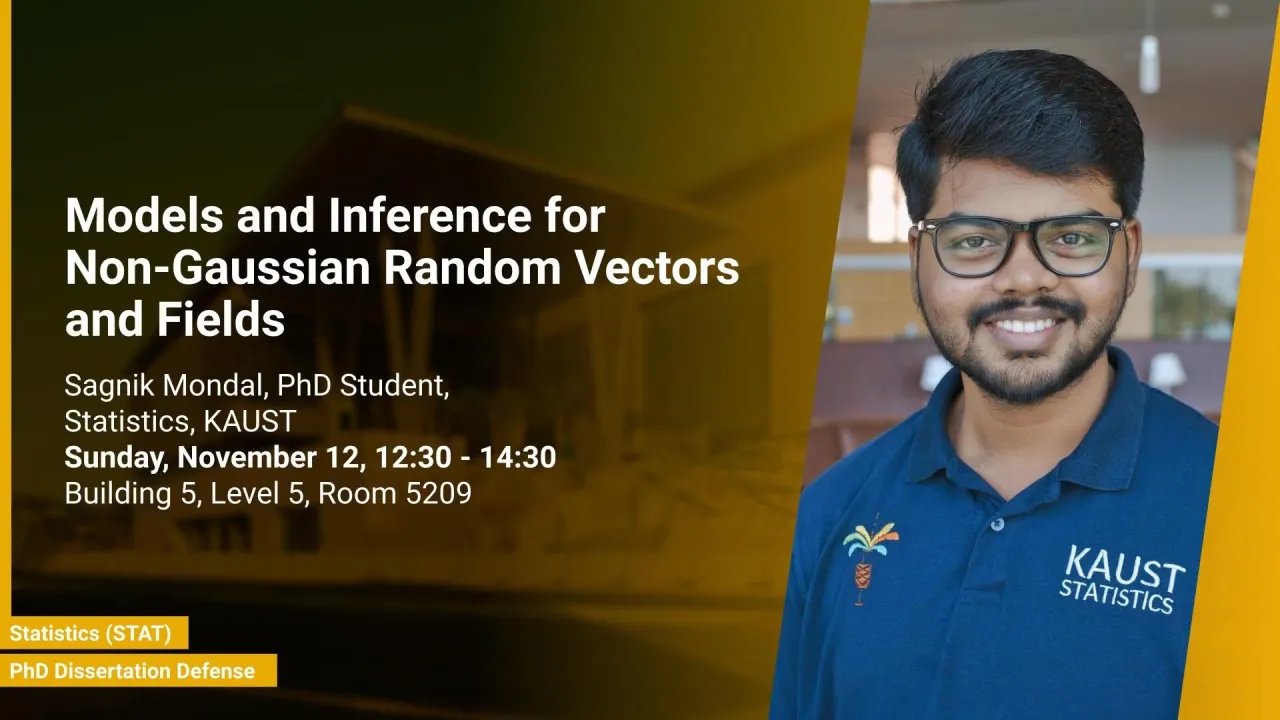
Models and Inference for Non-Gaussian Random Vectors and Fields
B5 L5 R5209
The multivariate Gaussian distribution is widely used in many statistical applications due to its appealing features. However, real-world data often violate its assumptions, showing skewness and/or tail-thickness.
Overview
Abstract
The multivariate Gaussian distribution is widely used in many statistical applications due to its appealing features. However, real-world data often violate its assumptions, showing skewness and/or tail-thickness. One can use other parametric distributions which incorporate skewness and kurtosis in the model such as the skew-normal distribution proposed by Azzalini (ASN) and its various extensions, which are widely popular among practitioners.In this thesis, the first part addresses the singularity problem of the Fisher information matrix in the ASN family when skewness parameters are set to zero by introducing the multivariate modified skew-normal (MSN) distribution. Moreover, defining the MSN with an alternative parameterization, we can make the profile log-likelihood of its skewness parameter bounded, unlike the ASN family. While the MSN distribution resolves these two problems of the ASN distribution, it sacrifices stochastic features like closed-form expressions for marginal and conditional distributions, as well as for moments, making it less practical for statistical applications.In the second part, we tackle one limitation of the ASN and the MSN distributions, namely their incapability of handling heavy-tailed data. We introduce the skew-normal-Tukey-h distribution by applying Tukey’s h transformation to the marginals of an ASN-distributed random vector but with the alternative parameterization used in defining the MSN distribution, enabling it to capture heavy-tail features. Varying Tukey’s h parameters for different marginals can have different tail-thickness, unlike the skew-t distribution, another ASN extension for modelling heavy tails. The skew-normal-Tukey-h distribution preserves appealing stochastic properties of the skew-normal distribution, making it apt for many statistical applications.Non-Gaussian models can also be constructed using copulas. In the third part, we introduce a copula-based model for non-Gaussian, non-stationary replicated spatial data. We study its extremal properties and inferential procedures. Yet another approach for modelling non-Gaussianity is transforming a Gaussian random vector, like the Tukey g-and-h random field, defined by applying the Tukey g-and-h transformation to induce skewness and tail-thickness. In the fourth part, we provide a parameter estimation method for the Tukey g-and-h random field using high-performance computing (HPC), for large scale geostatistical applications. Additionally, we provide the tile-low-rank approximation of the covariance matrix to facilitate even larger applications.
Brief Biography
Sagnik Mondal is currently a PhD student in the Statistics program at KAUST, in Spatio-Temporal Statistics & Data Science group led by Dr Marc G. Genton. Before joining KAUST, Sagnik completed a Master of Science degree in Statistics from the Indian Institute of Technology (IIT), Kanpur, India. His research interest is multivariate non-gaussian distribution and non-gaussian random field.