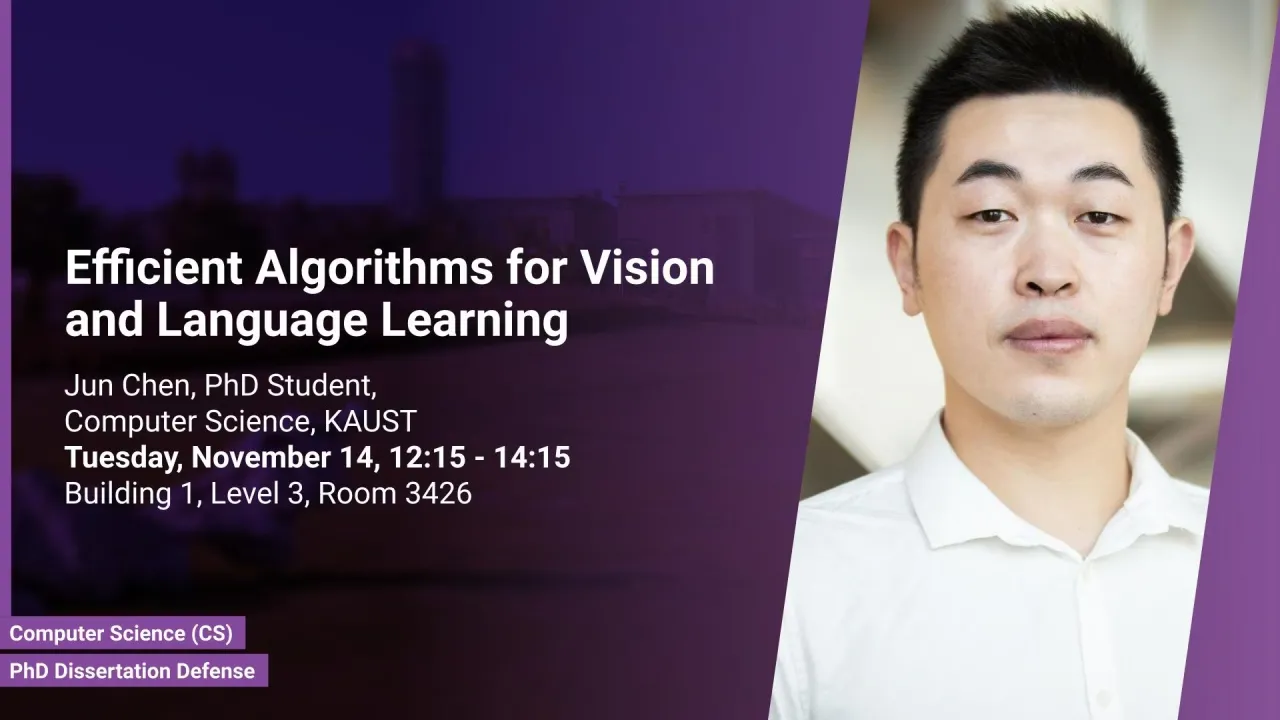
Efficient Algorithms for Vision and Language Learning
The development of advanced vision-language models necessitates considerable resources, both in terms of computation and data. There is growing interest in training these models efficiently and effectively and leveraging them for various downstream tasks. This dissertation presents several contributions aimed at improving both learning and data efficiency in vision-language learning, and how to leverage them into downstream tasks.
Overview
Abstract
The development of advanced vision-language models necessitates considerable resources, both in terms of computation and data. There is growing interest in training these models efficiently and effectively and leveraging them for various downstream tasks. This dissertation presents several contributions aimed at improving both learning and data efficiency in vision-language learning, and how to leverage them into downstream tasks. 1. We introduce VisualGPT, a data-efficient image captioning model that utilizes pre-trained language models to adapt to low-resource domains while preserving valuable linguistic knowledge through a unique self-resurrecting encoder-decoder attention mechanism. 2. We also propose MiniGPT-4, which is developed by efficiently aligning a frozen visual encoder with an advanced large language model to explore the advanced multi-modal generation capability. 3. We propose MiniGPT-v2, which allows the large language model to serve as a general interface and unifies many diverse vision-language tasks together. 4. We also propose ZeroSeg, which shows how a pretrained vision-language model can effectively benefit the semantic segmentation without any pixel-level supervision. 5. We also propose a large-scale video dataset called MammalNet, which facilitates the development of models that compositionally generalize well across different mammal behaviors and taxonomies. We have evaluated our model on multiple benchmarks which demonstrate significant improvements over existing state-of-the-art techniques, thereby contributing to the ongoing evolution of efficient learning in vision-language models. The insights and methodologies presented herein aim to accelerate real-world applications and pave the way for future research and development in this interdisciplinary field.
Brief Biography
Jun Chen is a PhD candidate in the VISION-CAIR team under the supervision of Prof. Mohamed Elhoseiny. His research works focus on vision-language learning. He has published many works in this domain, such as VisualGPT, MiniGPT-4, and MiniGPT-v2. In his long-term goal, he wants to dedicate his career to the AGI in the future.