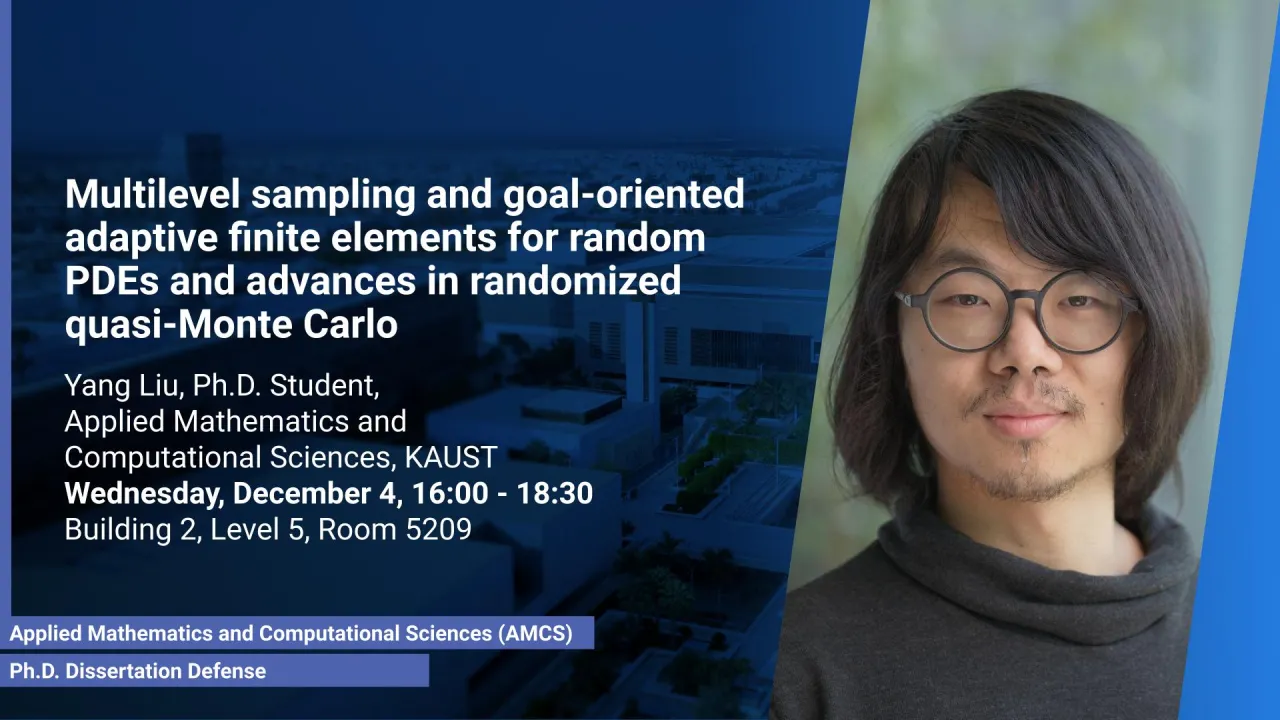
Multilevel sampling and goal-oriented adaptive finite elements for random PDEs and advances in randomized quasi-Monte Carlo
B2, L5, R5209
This thesis explores numerical methods for uncertainty quantification in systems governed by random partial differential equations (PDEs) and advances in randomized quasi-Monte Carlo (RQMC) techniques.
Overview
In the first part, we present an adaptive multilevel Monte Carlo (AMLMC) algorithm to efficiently approximate expected quantities of interest (QoIs). The AMLMC framework integrates isoparametric finite element approximations with dual-weighted residual error representations, employing non-uniform adaptive meshes to handle geometric singularities. This method excels with lognormal diffusivity coefficients, addressing their variability while achieving improved computational complexity over standard multilevel Monte Carlo (MLMC). We also extend AMLMC to a quasi-Monte Carlo (QMC) approach, leveraging its faster convergence and incorporating importance sampling (IS) and control variates for enhanced efficiency.
The second part investigates theoretical advancements in RQMC methods, emphasizing nonasymptotic convergence for linear elliptic PDEs with lognormal coefficients and variance analysis of RQMC estimators. The nonasymptotic analysis establishes error bounds for RQMC in the pre-asymptotic regime, while spectral analysis determines the convergence rate for both smooth and singular integrands.
Presenters
Brief Biography
Yang Liu is a Ph.D. candidate at Stochastic Numerics Research Group (STOCHNUM) under the supervision of Professor Raul F. Tempone at King Abdullah University of Science and Technology (KAUST). His primary research interests involve uncertainty quantification, Monte Carlo methods, and finite element methods. He obtained a Master's Degree in Applied Mathematics and Computational Science from KAUST in 2019.