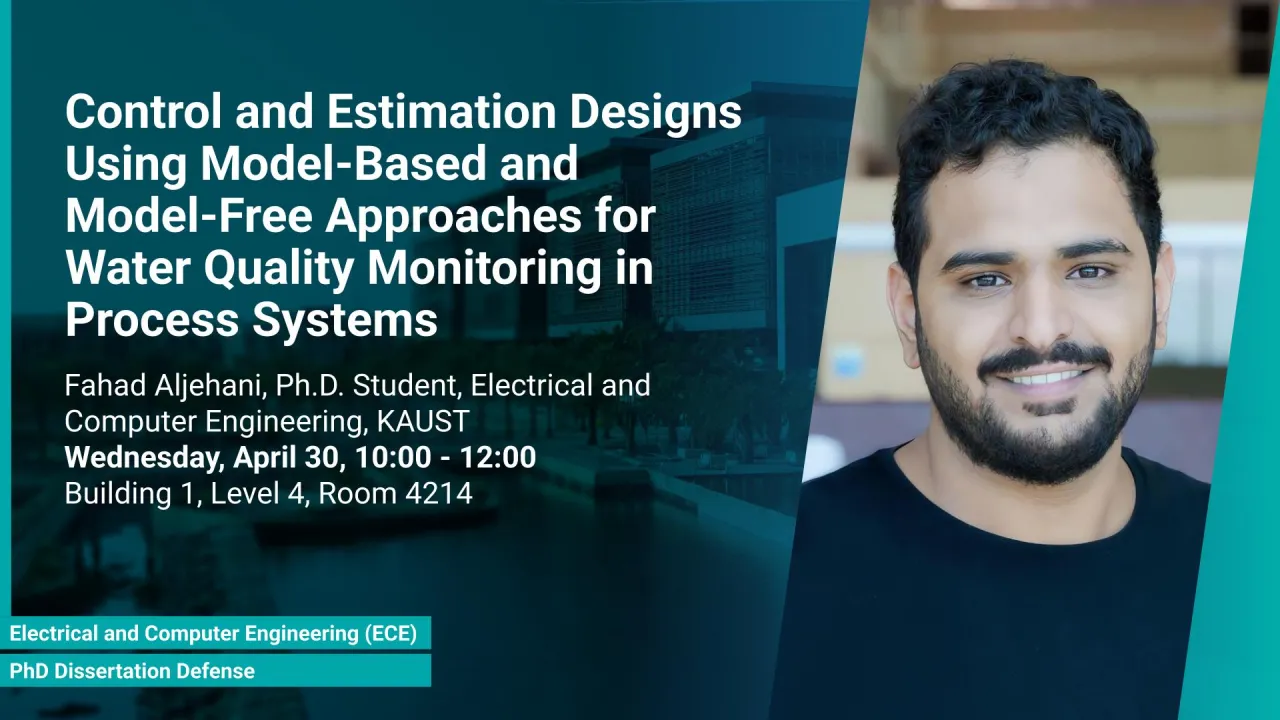
Control and Estimation Designs Using Model-Based and Model-Free Approaches for Water Quality Monitoring in Process Systems
This dissertation develops and evaluates advanced control and estimation strategies to address complex dynamics and measurement limitations in water-related applications, specifically optimizing fish growth in aquaculture and estimating bacterial concentration in wastewater treatment plants.
Overview
This dissertation addresses the design and evaluation of advanced control and estimation approaches in process systems, with a particular emphasis on water-related application systems such as aquaculture systems and wastewater treatment plants (WWTPs). Despite the differences in the environmental operations in aquaculture and WWTP systems, they both share challenges, such as complex system dynamics, limited direct measurements, and the need for robust monitoring and decision-making strategies.
In aquaculture, the ultimate objective is to promote optimal fish growth while minimizing food waste. Various control strategies—including bang-bang control, proportional-integral-derivative (PID) control, model predictive control (MPC), and reinforcement learning (RL) via Q-learning—were investigated under various un-ionized ammonia (UIA), a crucial parameter influencing fish mortality. Each controller effectively tracked the desired fish growth trajectory; however, MPC demonstrated superior efficiency by reducing food consumption due to its ability to incorporate constraints and multi-input objectives. Conversely, reinforcement learning (RL) control provided a feeding policy and maintained a relative food consumption that might underfeed the fish. To address the absence of a direct commercial fish weight sensor, this dissertation introduces an extended Kalman filter (EKF) that effectively estimates fish weight under varying initial conditions and noises.
For WWTPs, this dissertation proposes data-driven approaches for bacterial concentration estimation, a key indicator of treatment effectiveness. Given the challenges of limited data and lack of model generalizability across plants, decision tree models—such as k-nearest neighbors, random forest, gradient boosting regression, and extreme gradient boosting—were evaluated for bacterial concentration estimation. Additionally, a calibration framework was proposed to enhance the generalization of neural network (NN) models by continuously updating the trained model with receiving new samples.
Presenters
Brief Biography
Fahad Aljehani is a Ph.D. candidate in Electrical and Computer Engineering at King Abdullah University of Science and Technology (KAUST), where he is supervised by Professors Taous‑Meriem Laleg‑Kirati and Eric Feron. His research integrates classical control theory with modern AI and machine‑learning techniques to create advanced control and estimation frameworks for complex process systems, particularly optimal feeding in aquaculture and bacteria monitoring in wastewater treatment plants.
Aljehani earned his M.S. in Electrical Engineering from KAUST (2019), developing control strategies for distributed solar collectors and a virtual sensor for solar‑irradiance estimation. He holds a B.S. in Electrical Engineering from University of Dayton (2016).