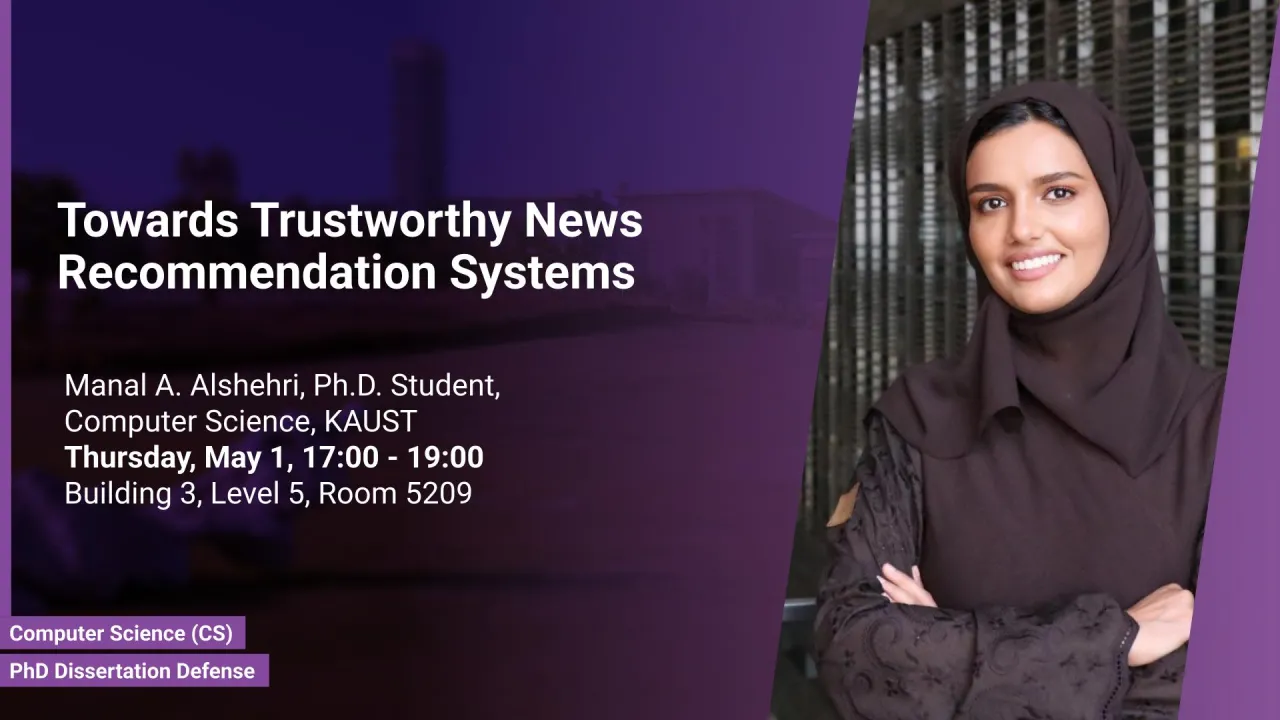
Towards Trustworthy News Recommendation Systems
This thesis enhances the trustworthiness of news recommendation systems by addressing the cold start problem for new users/items, enabling efficient user data removal for privacy, and mitigating multi-dimensional filter bubbles to improve diversity.
Overview
The rapid shift to digital news consumption has led to the widespread adoption of personalized news recommendation systems. While these systems improve content relevance and engagement, they also pose significant challenges in trustworthiness, particularly in ensuring accuracy, privacy, robustness, and diversity. This thesis aims to enhance the trustworthiness of news recommendation systems by addressing three critical challenges: the cold start problem (CSP), recommendation unlearning, and filter bubble formation.
To tackle the cold start problem, we propose GAZRec, a generative adversarial zero-shot learning framework that synthesizes virtual user-news interactions, enabling effective recommendations for new users and articles. Experimental results demonstrate that GAZRec significantly improves accuracy and robustness in cold-start scenarios while maintaining high recommendation performance under standard conditions.
For recommendation unlearning, we introduce FlipRec, a label-flipping-based framework that enables efficient removal of user preferences while preserving model performance. Unlike traditional retraining methods, FlipRec ensures privacy compliance and robustness without excessive computational overhead, making it suitable for large-scale recommendation systems.
Additionally, we investigate the multi-dimensional nature of filter bubbles, analyzing their formation across topic, sentiment, and political leaning dimensions. Unlike prior studies that focus on single-dimensional biases, our work reveals how biases in one dimension reinforce those in others, narrowing user exposure over time. By examining the long-term effects of filter bubbles, this research provides insights into balancing personalization with diversity, fostering a more inclusive and fair news recommendation environment.
The findings of this thesis contribute to the development of more trustworthy news recommendation systems by improving accuracy for cold-start users, enhancing privacy through efficient unlearning, and promoting diversity by mitigating multi-dimensional filter bubbles. Our work paves the way for future advancements in ethical and responsible AI-driven news recommendation.
Presenters
Brief Biography
Manal A. Alshehri is a Doctoral Candidate in Computer Science at King Abdullah University of Science and Technology (KAUST). She holds a B.S. and M.S. degree in Computer Science from King Abdulaziz University, where she also serves as a lecturer. Her research has been published in leading international conferences, including IEEE Big Data and CIKM.