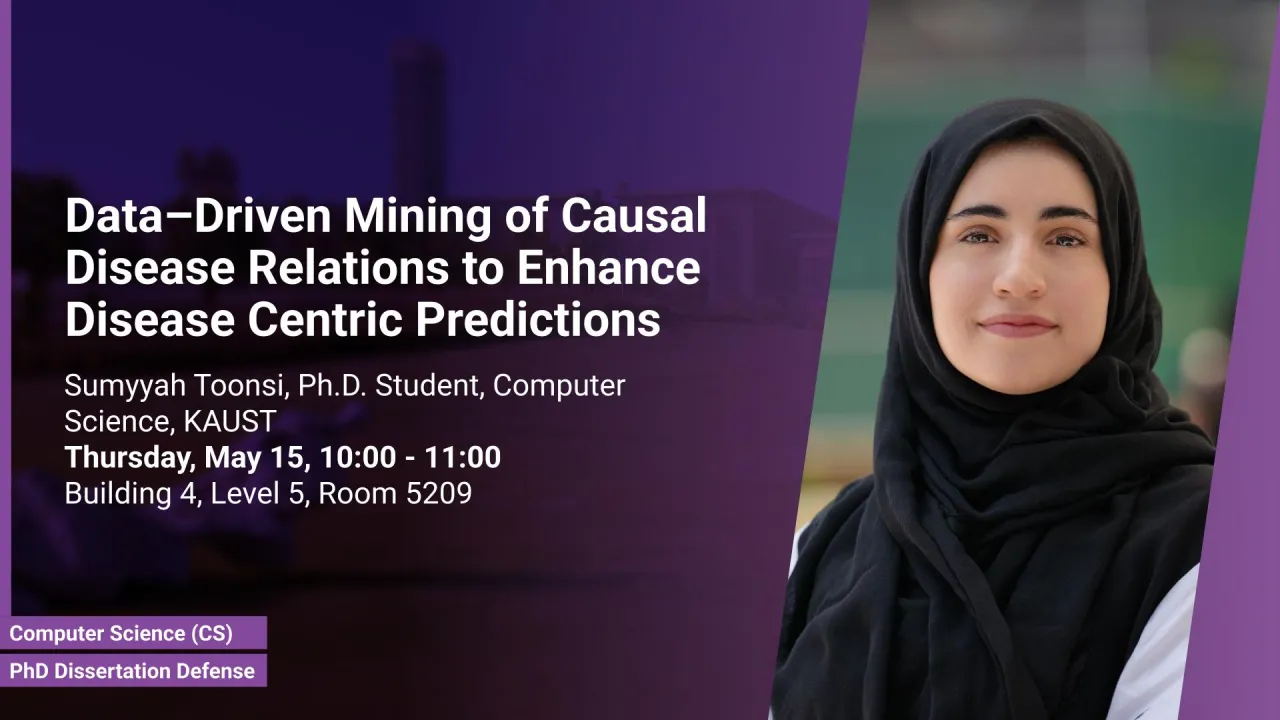
Data–Driven Mining of Causal Disease Relations to Enhance Disease Centric Predictions
B4 L5 R5209
This thesis develops a framework to extract and leverage inter-disease causal relations from biomedical literature, thereby advancing disease-centric predictions, enhancing our understanding of disease mechanisms, and demonstrating the potential for causal knowledge-guided therapeutic discovery.
Overview
Understanding disease etiology is essential for advancing medical knowledge, improving diagnosis, and developing effective therapeutics. Complex diseases, influenced by both genetic and environmental factors, remain challenging to study. Identifying predictors and causal mechanisms of diseases is a central problem in the biomedical fields, driving the development of various computational methods. While comorbidity-based associations enhance disease prediction, they lack directionality and causality, limiting their utility for understanding causal pathways and applying causal inference. However, causal knowledge between diseases is abundant in the biomedical literature. This presents an opportunity for relation extraction from text to be utilized by computational methods.
This thesis presents a framework for extracting and leveraging inter-disease causal relations to enhance disease-centric predictions. First, I address the challenge of identifying disease mentions in unstructured text. Although supervised named entity recognition (NER) models achieve high performance, generating manually curated training datasets is costly. Distant supervision using controlled vocabularies and biomedical ontologies helps mitigate this challenge, yet the full potential of ontology components, such as formal axioms, remains underexplored. I investigate how various ontology components can enhance distant supervision for NER and propose a generalized normalization method to improve entity linking.
Next, I develop a method to extract causal relationships between diseases from biomedical texts, addressing limitations in existing resources, such as restricted scope, lack of validation, and the absence of linked disease identifiers. By integrating standardized disease representations and validating extracted relations, I construct a dataset that supports the integration of causal relationships between diseases into bioinformatic applications. Furthermore, I provide an acyclic directed graph of the relations and demonstrate how it can be used for causal inference.
Finally, I demonstrate applications that leverage these mined causal relations to improve disease prediction and inference. Specifically, I show how incorporating causal knowledge enhances polygenic risk prediction of complex diseases and explains pleiotropic effects of genetic variants. Furthermore, I explore causal inference techniques, applying causal mediation analysis to identify drugs that mediate disease-disease relationships. Through these contributions, this thesis advances our understanding of disease mechanisms and demonstrates the potential of causal knowledge-guided approaches for predictive modeling and therapeutic discovery.
Presenters
Brief Biography
Sumyyah Toonsi is a PhD candidate in Computer Science at King Abdullah University of Science and Technology (KAUST), with a focus on bioinformatics. Her work integrates text mining, genetic risk prediction, and causal inference to advance understanding of complex biomedical data. She applies computational methods to support data-driven discoveries in health and disease.