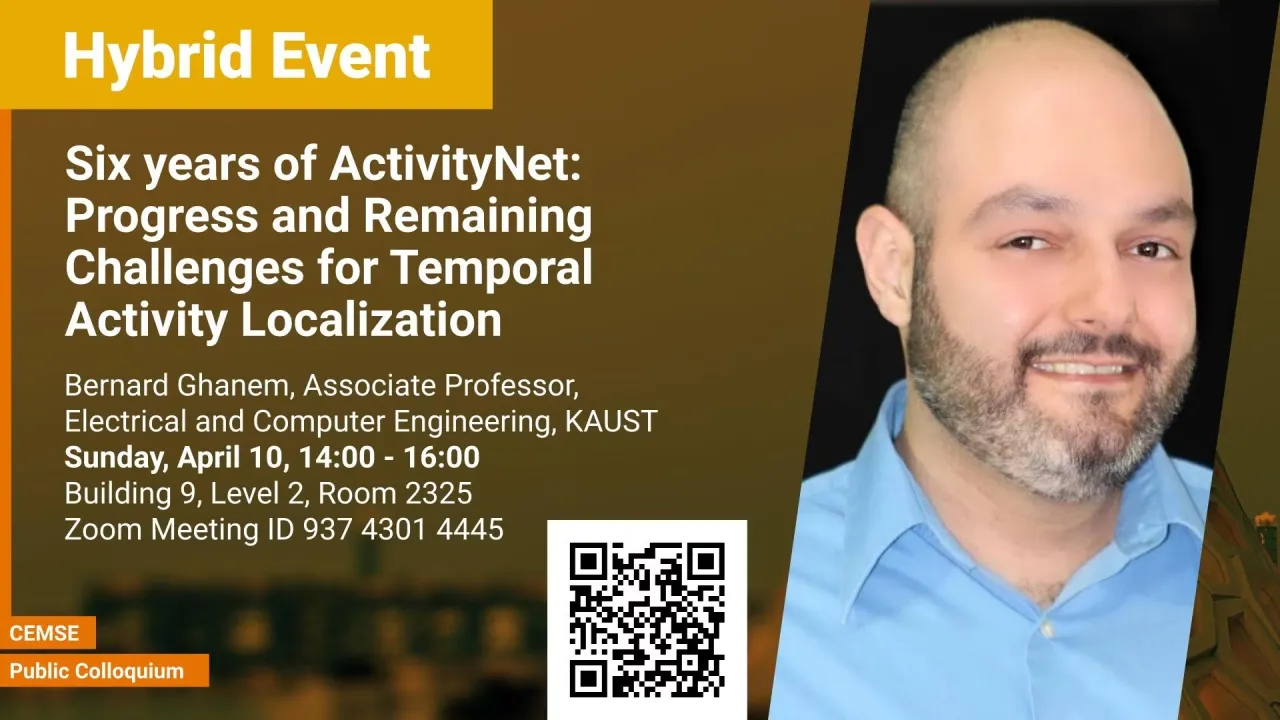
Six years of ActivityNet: Progress and Remaining Challenges for Temporal Activity Localization
Recognizing and localizing human activities in long-form untrimmed video is one of the most important applications of computer vision. This remains a difficult problem given the many challenges inherent to untrimmed video ranging from its large-scale nature to the need for rich video representation and context modeling. In an effort to better understand this problem, ActivityNet was proposed in 2015 to provide a large-scale benchmark to train and evaluate activity localization methods. Since then, it has become a standard in the community, and its annual workshop at CVPR has become a popular venue for research groups to compete and present their newest approaches. Despite the advances in the past several years, the performance of activity localization methods in video remains limited, especially in comparison with human performance. In this colloquium, I will give a compact view of the progress made in the task of temporal activity localization, identify some key remaining challenges, and present some future directions.
Overview
Abstract
Recognizing and localizing human activities in long-form untrimmed video is one of the most important applications of computer vision. This remains a difficult problem given the many challenges inherent to untrimmed video ranging from its large-scale nature to the need for rich video representation and context modeling. In an effort to better understand this problem, ActivityNet was proposed in 2015 to provide a large-scale benchmark to train and evaluate activity localization methods. Since then, it has become a standard in the community, and its annual workshop at CVPR has become a popular venue for research groups to compete and present their newest approaches. Despite the advances in the past several years, the performance of activity localization methods in video remains limited, especially in comparison with human performance. In this colloquium, I will give a compact view of the progress made in the task of temporal activity localization, identify some key remaining challenges, and present some future directions.
Brief Biography
Bernard Ghanem is currently an Associate Professor in the CEMSE division, a theme leader at the Visual Computing Center (VCC), and the Deputy Director of the AI Initiative at KAUST. His research interests lie in computer vision and machine learning with emphasis on topics in video understanding, 3D recognition, and theoretical foundations of deep learning. He received his Bachelor’s degree from the American University of Beirut (AUB) in 2005 and his MS/PhD from the University of Illinois at Urbana-Champaign (UIUC) in 2010. His work has received several awards and honors, including six Best Paper Awards for workshops in CVPR, ECCV, and ICCV, a Google Faculty Research Award in 2015 (1st in MENA for Machine Perception), and a Abdul Hameed Shoman Arab Researchers Award for Big Data and Machine Learning in 2020. He has co-authored more than 150 papers in his field. He serves as an Associate Editor for IEEE Transactions on Pattern Analysis and Machine Intelligence (TPAMI) and has served as Area Chair (AC) for CVPR, ICCV, ECCV, ICLR, and AAAI.