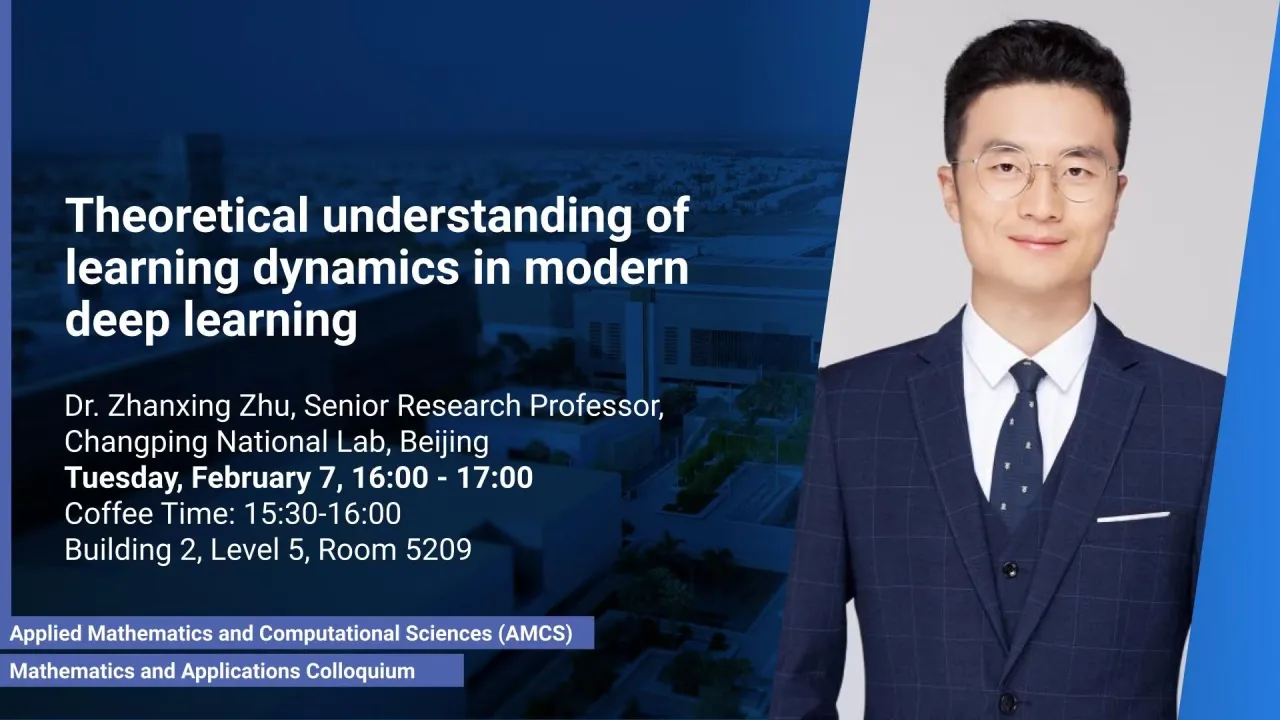
Theoretical understanding of learning dynamics in modern deep learning
- Dr. Zhanxing Zhu, Senior Research Professor. Changping National lab, Beijing
B2 L5 R5209
Coffee Time: 15:30-16:00. It has been a long-standing debate that “Is deep learning alchemy or science? ”, since the success of deep learning mainly relies on various engineering design and tricks, and lack of theoretical foundation. Unfortunately, the underlying mechanism of deep learning is still mysterious, severely limiting its further development from both theoretical and application aspects.
Overview
Coffee Time: 15:30-16:00
Abstract
It has been a long-standing debate that, “Is deep learning alchemy or science? ”, since the success of deep learning mainly relies on various engineering design and tricks, and lack of theoretical foundation. Unfortunately, the underlying mechanism of deep learning is still mysterious, severely limiting its further development from both theoretical and application aspects.
In this talk, I will introduce some of our attempts at theoretically understanding deep learning, mainly focusing on analyzing its training dynamics and tricks, including gradient descent, stochastic gradient descent (SGD), batch normalization and adversarial training. 1) We analyze the implicit regularization property of gradient descent and SGD from both local and global points of view, i.e. interpreting why SGD could find well generalizing minima compared with other alternatives; (2) We comprehensively reveal the learning dynamics of SGD with batch normalization and weight decay, named as Spherical Motion Dynamics, and show how the dynamics achieve its equilibrium state. (3) We theoretically characterize the implicit bias of adversarial training scheme widely used to improve the robustness resistant to adversarial attacks. Our results theoretically justify the longstanding conjecture that adversarial training modifies the decision boundary by utilizing adversarial examples to improve robustness. These new findings shed light on understanding the deep learning towards opening this black box and also inspire new algorithmic design. Finally, a landscape for the next generation of deep learning will be provided for further discussion.
Brief Biography
Dr. Zhanxing Zhu, is Senior Research Professor at Changping National Lab, Beijing, China, leading a group on machine learning and its application for biology. He previously was an assistant professor at Peking University and Beijing Institute of Big Data Research. He obtained a Ph.D. degree in machine learning from the University of Edinburgh in 2016. His research interests cover machine learning and its applications in various domains. Currently, he mainly focuses on the theoretical and methodological foundation of deep learning, and AI for Computational Biology. He has co-authored more than 60 papers in top machine learning journals and conferences (with Google Scholar citation ~5000), such as NeurIPS, ICML, ICLR, etc. He has been serving area chair for top machine learning conferences AISTATS, IJCAI, and AAAI. He was awarded “2019 Alibaba Damo Young Fellow”, and obtained “Best Paper Finalist” from the top computer security conference CCS 2018.Bio text.