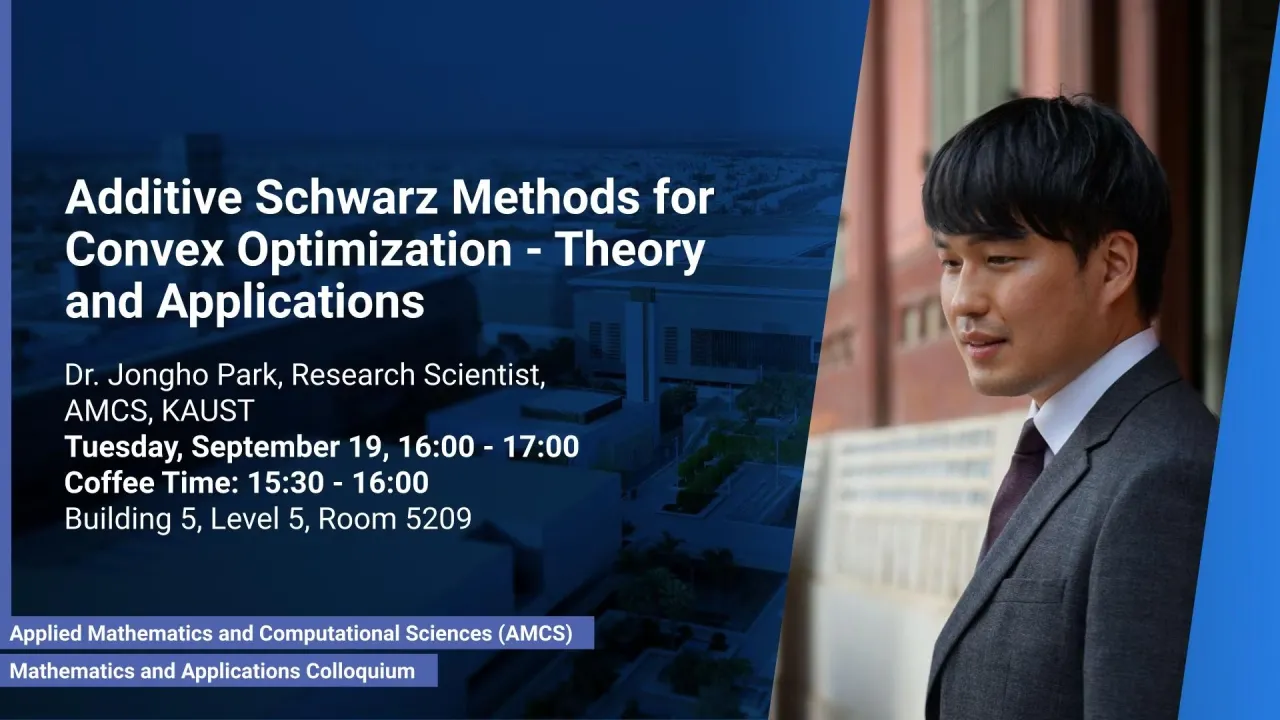
Additive Schwarz Methods for Convex Optimization - Theory and Applications
This talk is devoted to additive Schwarz methods for convex optimization. First, we propose an abstract framework for additive Schwarz methods for convex optimization. The framework's flexibility allows it to handle composite optimization problems and inexact local solvers. Moreover, it establishes a sharp convergence theory that agrees with the classical theory when addressing linear problems.
Overview
Abstract
This talk is devoted to additive Schwarz methods for convex optimization. First, we propose an abstract framework for additive Schwarz methods for convex optimization. The framework's flexibility allows it to handle composite optimization problems and inexact local solvers. Moreover, it establishes a sharp convergence theory that agrees with the classical theory when addressing linear problems. Next, we present various applications of the proposed framework, including nonlinear elliptic partial differential equations, variational inequalities, mathematical imaging, and machine learning. Within each application, the proposed framework enables us to uncover new intriguing properties of the methods.
Brief Biography
Jongho Park is a research scientist in CEMSE at KAUST. He received a B.S. degree in Mathematical Sciences/Computer Science in 2013, and M.S. and Ph.D. degrees in Mathematical Sciences in 2015 and 2019, respectively, all from KAIST. From 2019 to 2020, he was a postdoctoral researcher in the Department of Mathematical Sciences at KAIST. From 2020 to 2023, he was a research assistant professor in the Natural Science Research Institute at KAIST. His research interests include multilevel methods for mathematical optimization, scientific machine learning, numerical methods for structural mechanics, and data-driven methods in combustion dynamics.