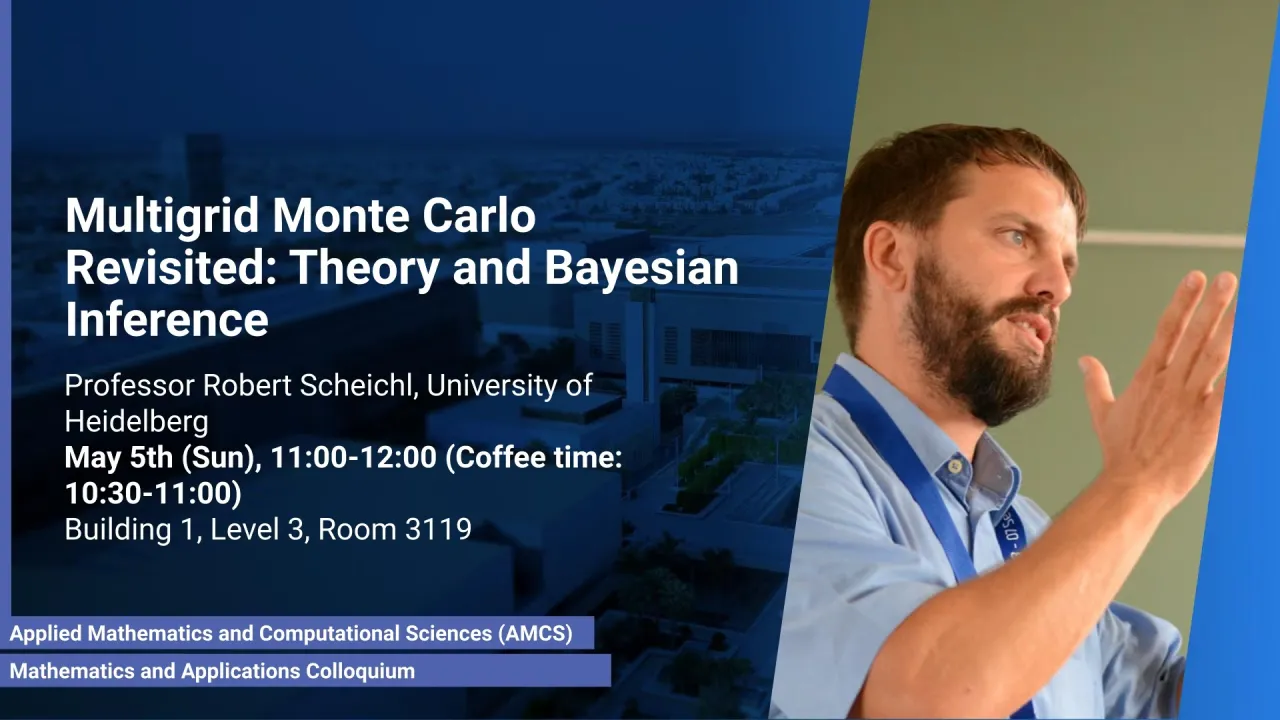
Multigrid Monte Carlo Revisited: Theory and Bayesian Inference
Overview
Abstract
The fast simulation of Gaussian random fields plays a pivotal role in many research areas such as Uncertainty Quantification, data science and spatial statistics. In theory, it is a well understood and solved problem, but in practice the efficiency and performance of traditional sampling procedures degenerates quickly when the random field is discretised on a grid with spatial resolution going to zero. Most existing algorithms, such as Cholesky factorisation samplers, do not scale well on large-scale parallel computers. On the other hand, stationary, iterative approaches such as the Gibbs sampler become extremely inefficient at high grid resolution. Already in the late 1980s, Goodman, Sokal and their collaborators wrote a series of papers aimed at accelerating the Gibbs sampler using multigrid ideas. The key observation is the intricate connection of random samplers, such as the Gibbs method, with iterative solvers for linear systems: both methods have very similar convergence properties. Based on this observation, they proposed the so-called multigrid Monte Carlo (MGMC) method - a random analogue of the multigrid method for solving discretised partial differential equations. We revisit the MGMC algorithm and provide rigorous theoretical justifications for the optimal scalability of the method for large scale problems: we show that the cost for generating an independent sample grows linearly with the problem size. Our analysis also includes the important setting of a Gaussian random field conditioned on noisy data via a Bayesian approach; in this case, the precision matrix is a finite-rank perturbation of some differential operator. By using a bespoke variant of the Gibbs sampler with a low-rank update on each level of the multigrid hierarchy we are able to generate Markov chains with a fixed, grid-independent integrated autocorrelation time. Our theoretical analysis is confirmed by numerical experiments for sampling rough Gaussian random fields in two and three dimensions. In the latter case and at high resolution MGMC is able to produce independent samples at a faster rate than a Cholesky sampler based on the CHOLMOD and Eigen libraries.
Brief Biography
* Professor of Numerical Analysis, University of Heidelberg, Germany (since 2018)
* Chairman of the Graduate School HGS MathComp, University of Heidelberg, Germany (since 2021)
* Professor of Scientific Computing, University of Bath, UK (part-time, 20% since 2018)
* Distinguished Romberg Guest Professorship, University of Heidelberg (2014–2017)
* Deputy Head of Department, University of Bath, UK (2016–2018)
* Lecturer & Senior Lecturer in Applied Mathematics, University of Bath, UK (2002–2011)
* Marie-Curie Postdoctoral Fellow, Institut Français du Pétrole, Paris (2001–2002)
* Ph.D. in Mathematics, University of Bath, UK (2000)