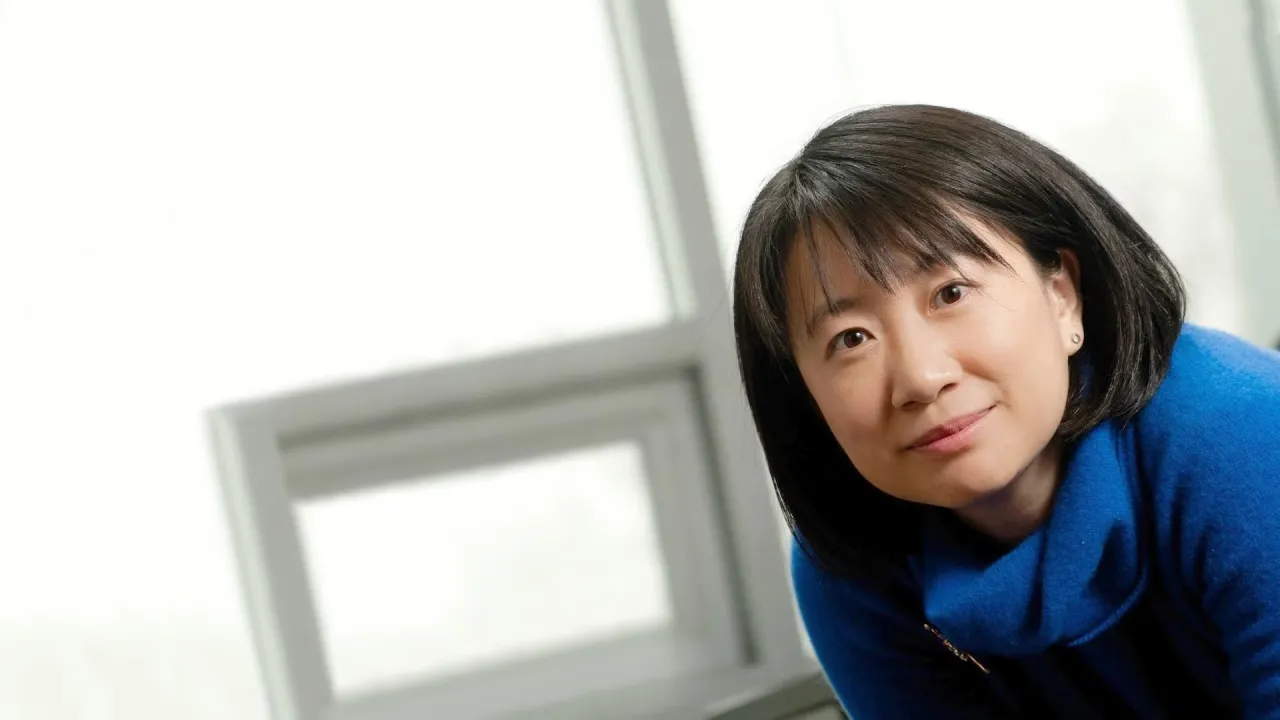
High-dimensional Q-learning for Dynamic Treatment Regimes
Dynamic treatment regimes are a set of decision rules and each treatment decision is tailored over time according to patients’ responses to previous treatments as well as covariate history. There is a growing interest in development of correct statistical inference for optimal dynamic treatment regimes to handle the challenges of nonregularity problems in the presence of nonrespondents who have zero-treatment effects, especially when the dimension of the tailoring variables is high.
Overview
Light Lunch will be provided.
Abstract
Dynamic treatment regimes are a set of decision rules and each treatment decision is tailored over time according to patients’ responses to previous treatments as well as covariate history. There is a growing interest in development of correct statistical inference for optimal dynamic treatment regimes to handle the challenges of nonregularity problems in the presence of nonrespondents who have zero-treatment effects, especially when the dimension of the tailoring variables is high. In this talk, I will introduce a high-dimensional Q-learning (HQ-learning) to facilitate the inference of optimal values and parameters. The proposed method allows us to simultaneously estimate the optimal dynamic treatment regimes and select the important variables that truly contribute to the individual reward. At the same time, hard thresholding is introduced in the method to eliminate the effects of the nonrespondents. The asymptotic properties for the parameter estimators as well as the estimated optimal value function are then established by adjusting the bias due to thresholding. Both simulation studies and real data analysis demonstrate satisfactory performance for obtaining the proper inference for the value function for the optimal dynamic treatment regimes.
Brief Biography
Rui Song is an Associate Professor in Department of Statistics at North Carolina State University. She got her BS from Peking University in 2001 and PhD from University of Wisconsin, Madison in 2006, all in Statistics. Her current research focuses on statistical reinforcement learning, high-dimensional data analysis and precision medicine. Her research has been continuously supported as sole principle investigator by National Science Foundation (NSF). She received the prestigious NSF Faculty Early Career Development (CAREER) Award in 2016. She has published about 50 papers in top tier journals of statistics, including Annals of Statistics, Biometrika, Journal of the American Statistical Association and Journal of the Royal Statistical
Society: Series B. She serves as Associator Editor for several top tier journals of statistics including Journal of the American Statistical Association and Biometrics.