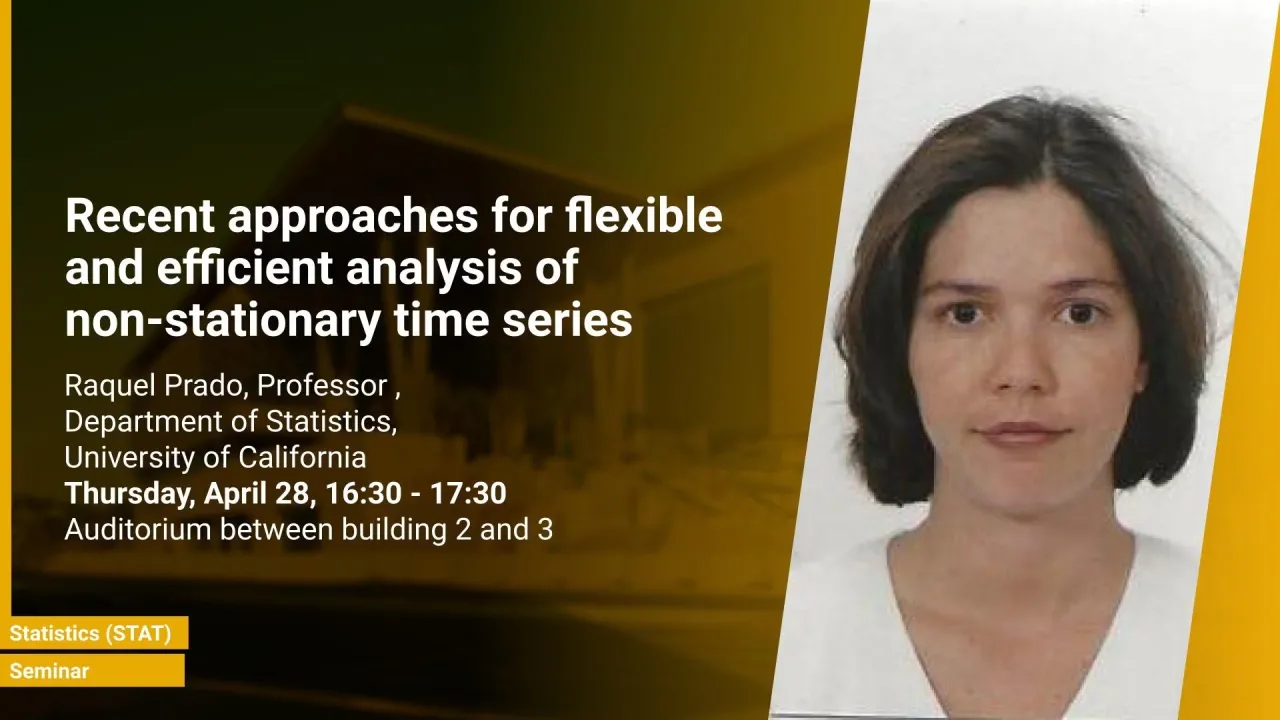
Recent approaches for flexible and efficient analysis of non-stationary time series
During the first part of the talk we present an approach that allows for flexible analysis of multivariate non-stationary time series via dynamic models on the partial autocorrelation domain. We discuss various aspects of these models, including the use of shrinkage priors to deal with overfitting issues, as well as hierarchical extensions.
Overview
Abstract
We present two model-based approaches for analyzing large-dimensional time series data with non-stationary features.
During the first part of the talk we present an approach that allows for flexible analysis of multivariate non-stationary time series via dynamic models on the partial autocorrelation domain. We discuss various aspects of these models, including the use of shrinkage priors to deal with overfitting issues, as well as hierarchical extensions. We summarize methods for posterior inference within this class of models, focusing on algorithms for efficient approximate filtering and smoothing. We illustrate the performance of these models and methods in the analysis of simulated and real data arising from environmental and neuroscience applications.
In the second part of the talk we discuss fast inference for time-varying quantiles using a dynamic quantile linear model that utilizes the extended asymmetric Laplace family of distributions. The model allows us to incorporate the potential current and lagged effects of covariates on the time-varying quantiles using a transfer function component. We discuss approaches for inference within this class of models, including an efficient importance sampling variational Bayes algorithm for fast approximate inference in large-dimensional settings. We show how these models can be used to estimate the effects of climatological indexes on the estimation of the upper quantiles of integrated water transport (IVT) magnitude data over time, which plays a key role in schemes aiming to automatically detect atmospheric rivers.
Brief Biography
Raquel Prado is Professor in the Department of Statistics of the Baskin School of Engineering at the University of California Santa Cruz, where she has been on the faculty since 2001. In 1998 she graduated with a PhD in Statistics and Decision Sciences from Duke University. From 1998 until 2001 she was an Assistant Professor in Statistics at Universidad Simon Bolivar in Venezuela. She was a co-recepient of the Outstanding Statistical Application Award of the American Statistical Association in 1999. She is a Fellow of the American Statistical Association. She is also a Fellow and Past President of the International Society for Bayesian Analysis and currently a member of the Committee on Applied and Theoretical Statistics (CATS) of the National Academies of Science, Engineering and Medicine.