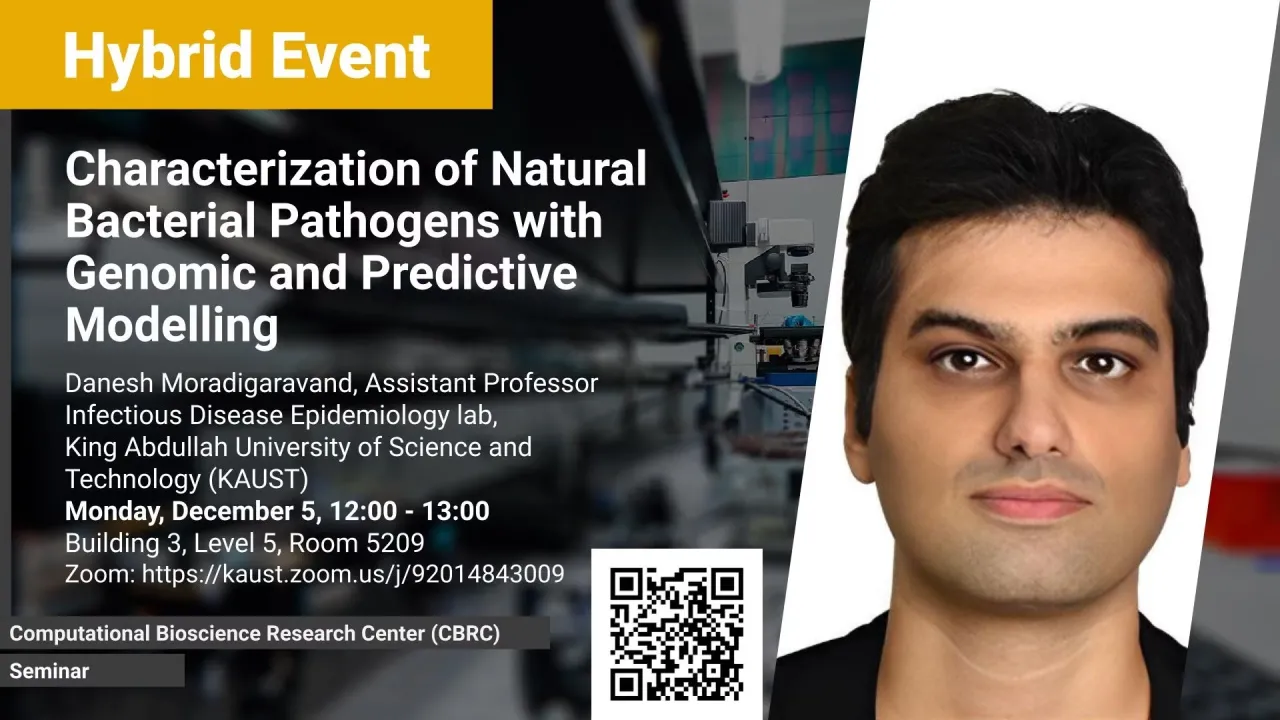
Characterization of Natural Bacterial Pathogens with Genomic and Predictive Modelling
- Dr. Danesh Moradigaravand, Infectious Disease Epidemiology lab, BESE, KAUST
B3 L5 R5209
In this talk, I will first present how the application of phylogenetic and phylodynamic methods to whole genome sequencing data of multidrug resistant bacterial pathogens provided an in-depth understanding of the epidemiology and evolution of these strains on epidemiological time scales. I will then discuss the characterization of the genomic repertoire of bacterial traits using a combination of machine learning, whole genome sequencing and large-scale phenotypic assays. I will then present the leverage of predictive modelling to predict bacterial features, e.g. antimicrobial resistance, growth, and horizontal gene transfer, from genomic biomarkers. I will finally discuss how large-scale phenotypic assays enabled us to identity genes underlying morphogenesis and biofilm formation.
Overview
Abstract
Bacterial phenotype in natural settings is determined by complex interactions between genes, hosts, and the environment. However, until recently, the limitations of methods and tools prevented a full characterization of the underpinning genetic mechanisms for phenotypes of clinical significance, such as antibiotic resistance and virulence. These limitations have been addressed with the recent advent of high-throughput sequencing and phenotypic assays. A large volume of data, along with powerful analytic and predictive tools, have revealed genotype-phenotype map for pathogenic traits in a broad range of bacterial strains. In this talk, I will first present how the application of phylogenetic and phylodynamic methods to whole genome sequencing data of multidrug resistant bacterial pathogens provided an in-depth understanding of the epidemiology and evolution of these strains on epidemiological time scales. I will then discuss the characterization of the genomic repertoire of bacterial traits using a combination of machine learning, whole genome sequencing and large-scale phenotypic assays. I will then present the leverage of predictive modelling to predict bacterial features, e.g. antimicrobial resistance, growth, and horizontal gene transfer, from genomic biomarkers. I will finally discuss how large-scale phenotypic assays enabled us to identity genes underlying morphogenesis and biofilm formation. Altogether, these approaches offer novel data driven frameworks in microbial precision and translational medicine.
Brief Biography
Dr. Moradigaravand is an assistant professor and group leader at the BESE department, KAUST. Prior to this position, he was a junior group leader and bioinformatics lead scientist at the University of Birmingham, Wellcome Sanger Institute and University of Cambridge. He obtained his PhD from ETH Zurich in theoretical population genetics. The infectious disease epidemiology lab will investigate next generation sequencing (NGS) and large-scale phenome data of microbial strains using a variety of statistical genetic, phylogenetic, and machine learning methods, to decipher the evolution and epidemiology of bacterial infectious diseases in Gram-negative and Gram-positive bacterial strains. The lab will also extensively utilize machine learning approaches to unravel the genotype-phenotype map for bacterial clinical features from genomic and metagenomic data.