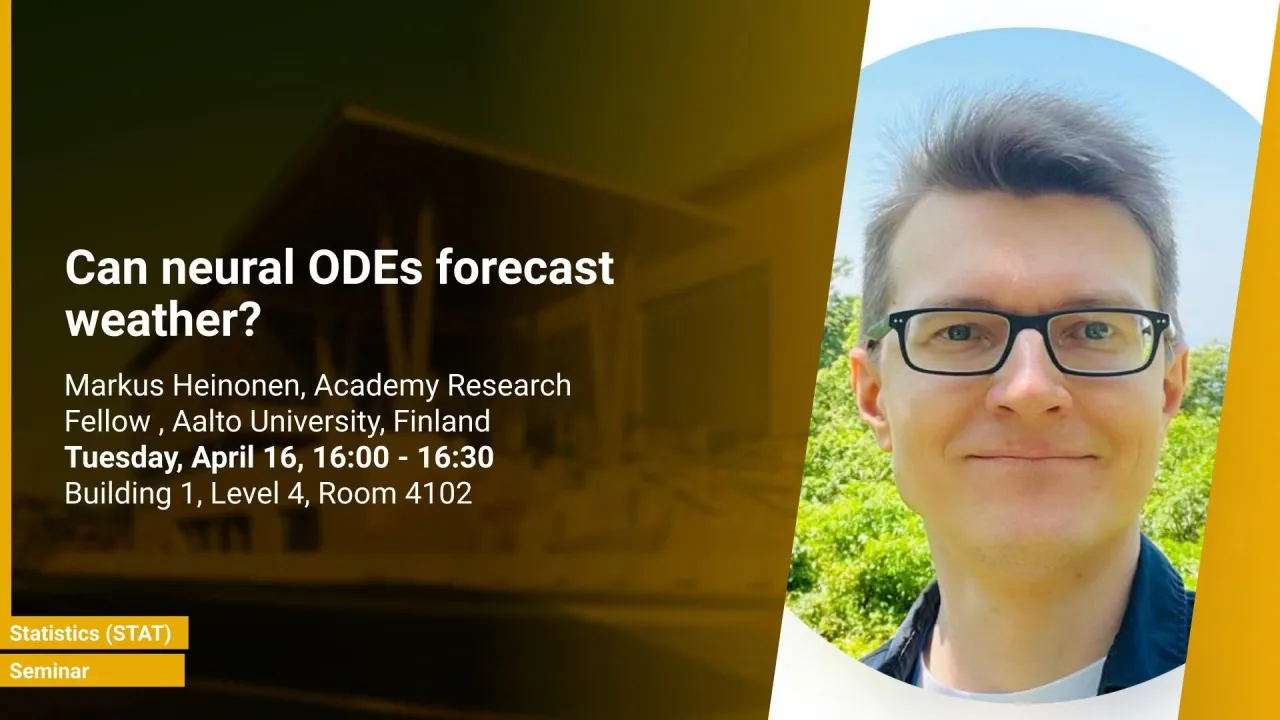
Can neural ODEs forecast weather?
- Dr. Markus Heinonen, Academy Research Fellow, Aalto Univeristy, Finland
B1 L4 R 4102
Neural ODEs have surfaced in the last decade as a new perspective on modelling dynamics by learning the time-derivative that drives the system evolution forward as a neural network.
Overview
Abstract
Neural ODEs have surfaced in the last decade as a new perspective on modelling dynamics by learning the time-derivative that drives the system evolution forward as a neural network. While succesful on systems of limited complexity, large-scale demonstrations have been lacking. Recently large autoregressive transformer models have been developed with breakthrough global weather forecasting performances, but at times with little consideration of the underlying dynamics. We consider global weather as a continuous-time PDE with mass-preserving dynamics, and show how simple convolution networks can achieve remarkable weather prediction performance with just a few million parameters. This talk is based on the paper “ClimODE: Climate Forecasting With Physics-informed Neural ODEs” accepted for ICLR’24 oral presentation.
Brief Biography
Markus Heinonen is an Academy Research Fellow at Aalto University, Finland with a PhD from University of Helsinki in 2013. His research interests are centered on probabilistic machine learning with emphasis on understanding uncertainty of deep learning with Bayesian perspectives to neural networks. In addition he has worked on incorporating neural networks and Gaussian processes into learning ODE, SDE and PDE dynamics.