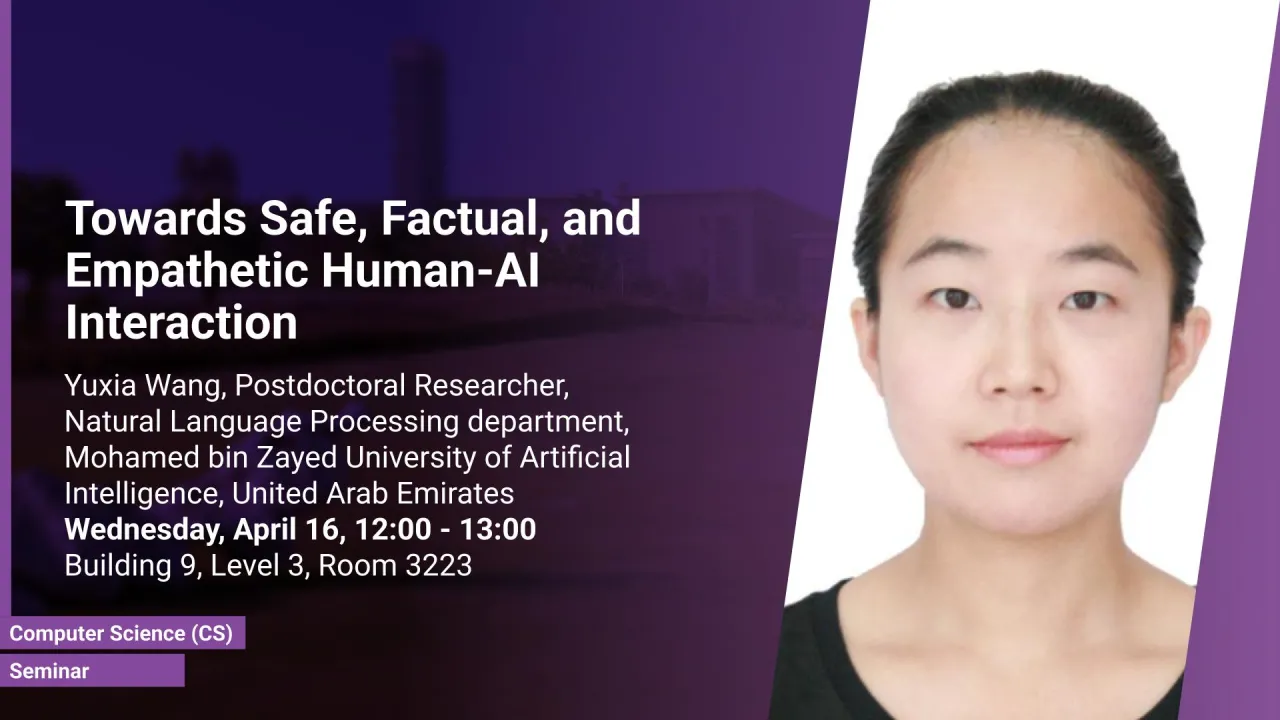
Towards Safe, Factual, and Empathetic Human-AI Interaction
This talk will explore the challenges of evaluating and improving LLM factuality, safety, and cultural sensitivity, addressing questions about hallucination rates, retrieval-augmented generation efficacy, fact-checking limitations, regional policy alignment, and the necessity of machine-generated content detection.
Overview
It is well known that LLMs sometimes hallucinate, generate factually incorrect content and follow risky instructions eliciting harmful responses. How many percent of content would be false on average? Does retrieval-augmented generation help? What’s the bottleneck for current fact-checking systems? Some responses are harmless in Australia while they may be sensitive and offensive in China and Arabic regions, and vice versa, why and how to align with region- and culture-specific policies? When the model outputs are safe, factual, and empathetic, why do we still need to detect machine-generated content? In this talk, I will introduce my research plans and my recent work on LLM factuality and safety evaluation, exploring answers of these questions with you together.
Presenters
Yuxia Wang, Postdoctoral Researcher, Natural Language Processing department, Mohamed bin Zayed University of Artificial Intelligence
Brief Biography
Yuxia Wang is a postdoctoral researcher in the NLP department at MBZUAI. Her research focuses on evaluating and enhancing LLMs in terms of factuality, safety, and empathy, with an emphasis on multilingual extension and multi-objective optimization in her recent studies. She also works on machine-generated content detection to mitigate the misuse of LLMs. Yuxia completed her PhD from The University of Melbourne, where she specialized in semantic textual similarity and uncertainty estimation for textual regression tasks, particularly in the clinical domain.