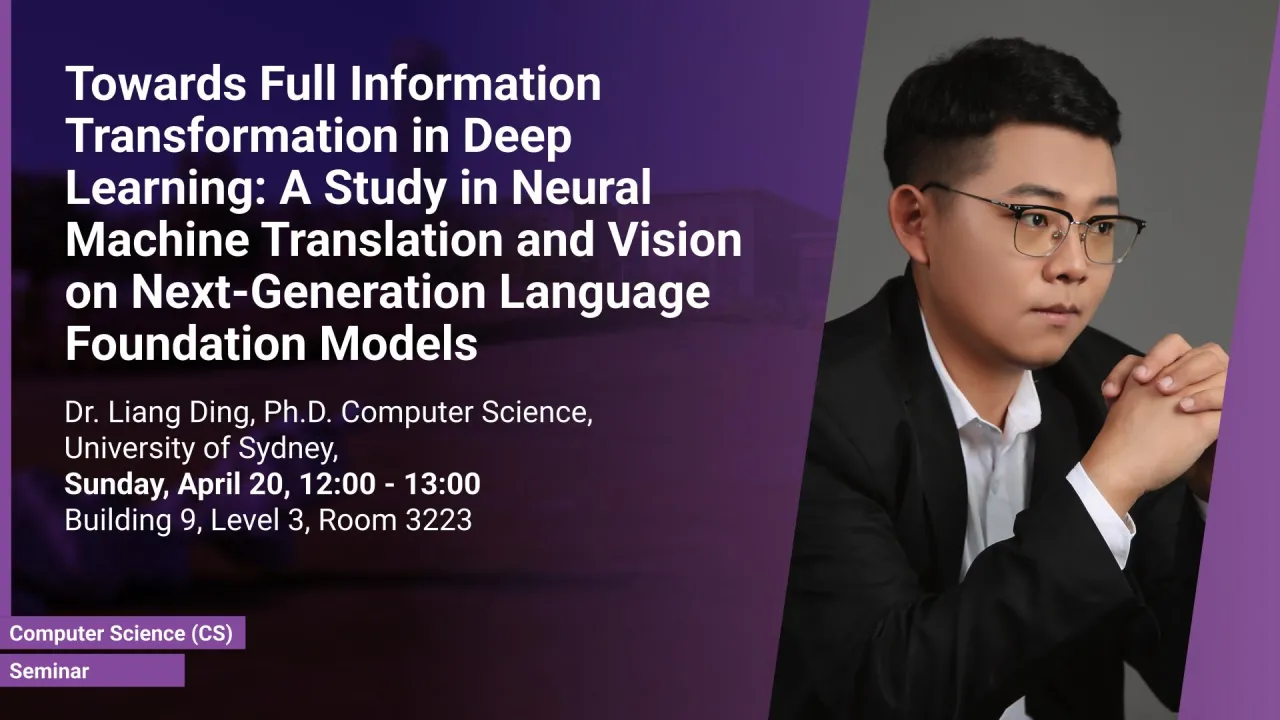
Towards Full Information Transformation in Deep Learning: A Study in Neural Machine Translation and Vision on Next-Generation Language Foundation Models
This seminar will present LLM advancements to address alignment and efficiency challenges in neural machine translation, aiming to balance decoding speed and translation quality towards building sufficient, efficient, and trustworthy next-generation language models for diverse real-world applications.
Overview
Large deep learning models face interpretability and efficiency challenges, especially in sequence-to-sequence tasks like neural machine translation (NMT). Two primary issues in NMT are: (1) translation errors due to misalignment between source and target representations and insufficient target-side modeling, and (2) high decoding latency by autoregressive frameworks. In this seminar, Dr. Liang Ding will present key advancements to address these challenges. He introduces methods to enhance alignment between source and target sequences by reordering high-level knowledge in the position encoding module of Transformers, and a self-evolution learning approach to fully exploit the data. He also discusses solutions to mitigate lexical choice errors in fast non-autoregressive translation, particularly for low-frequency terms, which can lead to inaccuracies, ultimately achieving a better balance between decoding speed and translation quality. Building on these sufficient and efficient advancements in NMT, Dr. Ding will provide an outlook on how to build sufficient, efficient, and trustworthy next-generation language foundation models. He will explore how these models can empower real-world applications, e.g., healthcare, business, robotics, and more.
Presenters
Dr. Liang Ding
Brief Biography
Dr. Liang Ding is a founding member and principal researcher in large language models at an AI research startup that has raised over 50 million USD. He holds a Ph.D. from the University of Sydney and has over nine years of experience in NLP and AI. His research, focusing on language model pretraining, alignment, multilinguality, and evaluation, has resulted in numerous publications in top venues, including ACL, EMNLP, ICLR, NeurIPS, and IEEE TPAMI, with over 4,100 citations and an h-index of 37. His work was selected for the Best Paper Nomination at ACL 2023 and has led to 20 patents, applied in industry solutions like Baidu DuerOS, JD Health, and Tencent Transmart. Dr. Ding has won over 10 prestigious AI competitions, including GLUE/SuperGLUE, WMT (2019–2022), and IWSLT 2021, surpassing human performance on GLUE tasks and outperforming institutes like Google, Meta, and OpenAI. He has served as Area and Session Chair for major NLP/AI conferences (e.g., ACL, EMNLP, NeurIPS) and led a project that received the 2022 Superior AI Leader Award at the World Artificial Intelligence Conference. In teaching, he has been an adjunct lecturer for NLP courses at NTU Singapore, Zhejiang University, Fudan University, and USTC, supervising numerous students. His ongoing research aims to develop efficient, robust, and trustworthy LLMs, with applications in healthcare, business, robotics, and more.