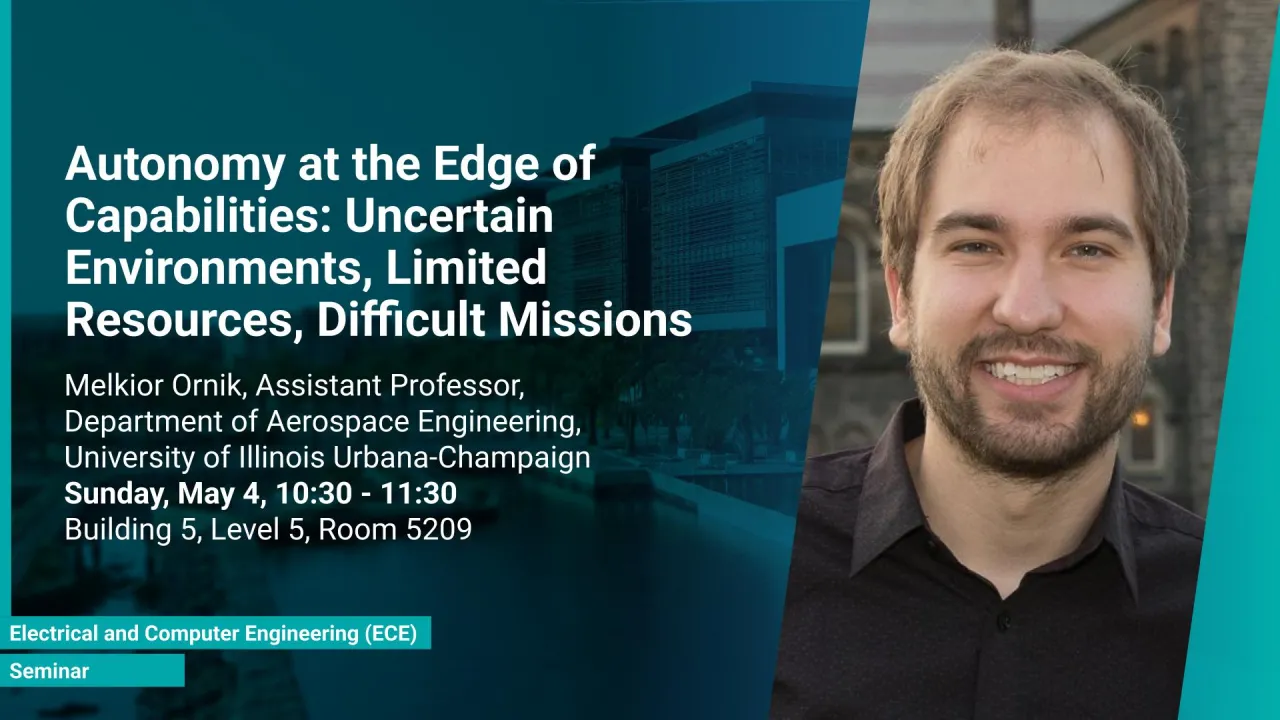
Autonomy at the Edge of Capabilities: Uncertain Environments, Limited Resources, Difficult Missions
This seminar introduces a planning strategy for complex autonomous systems that integrates machine learning with structure-driven abstraction and mission decomposition to achieve computationally tractable, scalable, and high-performing policies despite environmental and agent constraints.
Overview
Fast, successful, and efficient planning is a core challenge of high-level autonomy in complex environments. The obstacles are seemingly insurmountable. Individual agents often face constraints in terms of resource and compute availability, limited sensing and communication capabilities, and lack of a priori knowledge about the operating environment. Planning for large teams is burdened by either curse of dimensionality or complex organizational patterns of decentralization. As a result, standard machine learning methods are largely infeasible — for instance, due to lack of training data or the size of the state space — while human-driven solutions or simple heuristics often produce vastly suboptimal plans. The purpose of this talk is to propose a middle road. We will consider three broad problems in planning: budget-constrained teams, task-aware data collection, and time-optimal multi-target planning. Across two domains — infrastructure maintenance and maritime autonomy — we show that understanding the structure of agent interactions and the interplay between environment and mission progress is key in developing meaningful, computationally tractable policies. Consequently, our strategies combine machine learning reasoning with high-level structure-driven abstraction and mission decomposition. Early empirical work demonstrates that such an approach greatly outperforms existing benchmarks while retaining the capability to operate at impressively large scales.
Presenters
Melkior Ornik, Assistant Professor, Department of Aerospace Engineering, University of Illinois Urbana-Champaign
Brief Biography
Melkior Ornik is an assistant professor in the Department of Aerospace Engineering at the University of Illinois Urbana-Champaign, also affiliated with the Coordinated Science Laboratory and the Department of Electrical and Computer Engineering, as well as the Discovery Partners Institute in Chicago. He received his Ph.D. degree from the University of Toronto in 2017. His research focuses on developing theory and algorithms for control, learning and task planning in autonomous systems that operate in uncertain, changing, or adversarial environments, as well as in scenarios where only limited knowledge of the system is available. He is a senior member of IEEE and AIAA, his recent work has been extensively funded by the US federal government grants, and he has been selected for two federal Young Investigator Program awards.