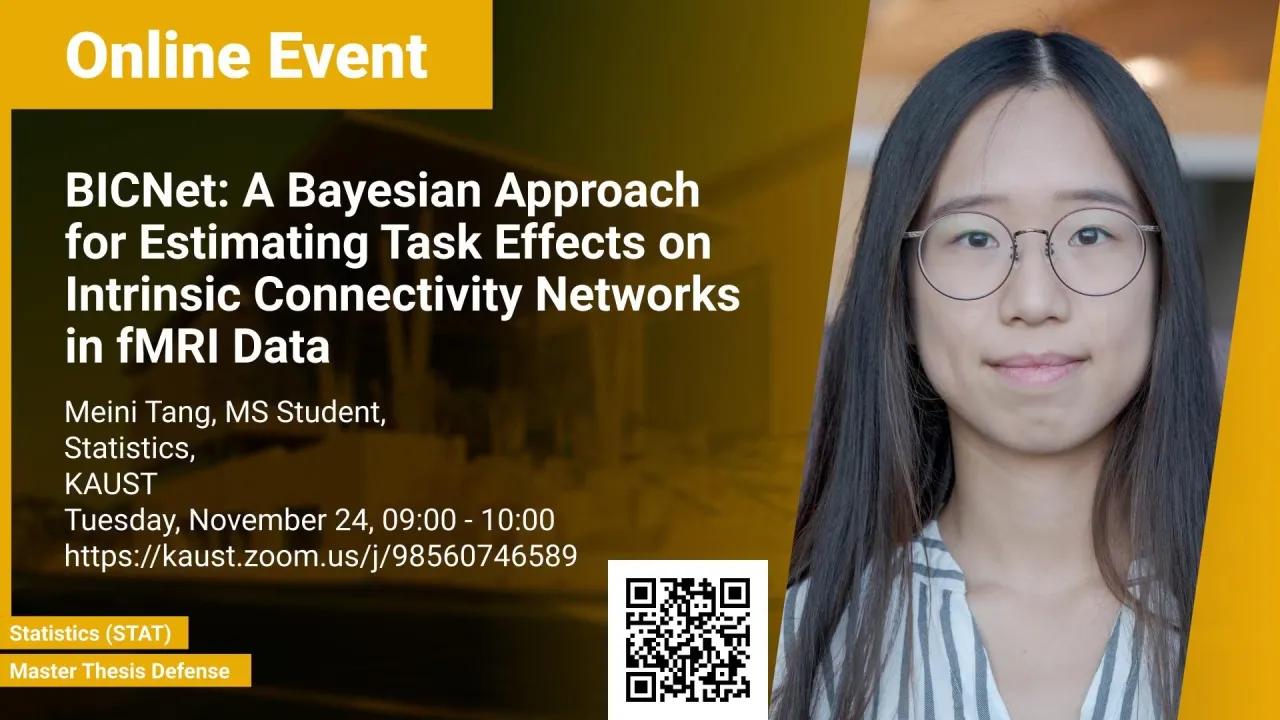
BICNet: A Bayesian Approach for Estimating Task Effects on Intrinsic Connectivity Networks in fMRI Data
Intrinsic connectivity networks (ICNs) refer to brain functional networks that are consistently found under various conditions, during tasks or at rest. Some studies demonstrated that while some stimuli do not impact intrinsic connectivity, other stimuli actually activate intrinsic connectivity through suppression, excitation, moderation or modification. Most analyses of fMRI data use ad-hoc methods to estimate the latent structure of ICNs. Modeling the effects on ICNs has also not been fully investigated. We propose a Bayesian Intrinsic Connectivity Network (BICNet) model, an extended Bayesian dynamic sparse latent factor model, to identify the ICNs and quantify task-related effects on the ICNs. BICNet has the following advantages: (1) It simultaneously identifies the individual and group-level ICNs; (2) It robustly identifies ICNs by jointly modeling rfMRI and tfMRI; (3) Compared to ICA-based methods, it can quantify the difference of ICN amplitudes across different states; (4) The sparsity of ICNs automatically performs feature selection, instead of ad-hoc thresholding. We apply BICNet to the rfMRI and language tfMRI data from the HCP and identify several ICNs related to distinct language processing functions.
Overview
Abstract
Intrinsic connectivity networks (ICNs) refer to brain functional networks that are consistently found under various conditions, during tasks or at rest. Some studies demonstrated that while some stimuli do not impact intrinsic connectivity, other stimuli actually activate intrinsic connectivity through suppression, excitation, moderation or modification. Most analyses of fMRI data use ad-hoc methods to estimate the latent structure of ICNs. Modeling the effects on ICNs has also not been fully investigated. We propose a Bayesian Intrinsic Connectivity Network (BICNet) model, an extended Bayesian dynamic sparse latent factor model, to identify the ICNs and quantify task-related effects on the ICNs. BICNet has the following advantages: (1) It simultaneously identifies the individual and group-level ICNs; (2) It robustly identifies ICNs by jointly modeling rfMRI and tfMRI; (3) Compared to ICA-based methods, it can quantify the difference of ICN amplitudes across different states; (4) The sparsity of ICNs automatically performs feature selection, instead of ad-hoc thresholding. We apply BICNet to the rfMRI and language tfMRI data from the HCP and identify several ICNs related to distinct language processing functions.
Brief Biography
- Started M.Sc in Spring 2019 under the supervision of Prof. Hernando Ombao at King Abdullah University of Science and Technology
- Obtained B.Sc in Applied Statistics from Sun Yat-sen University in China.
- Research interests: time series analysis, brain-network analysis, Bayesian statistics, and machine learning