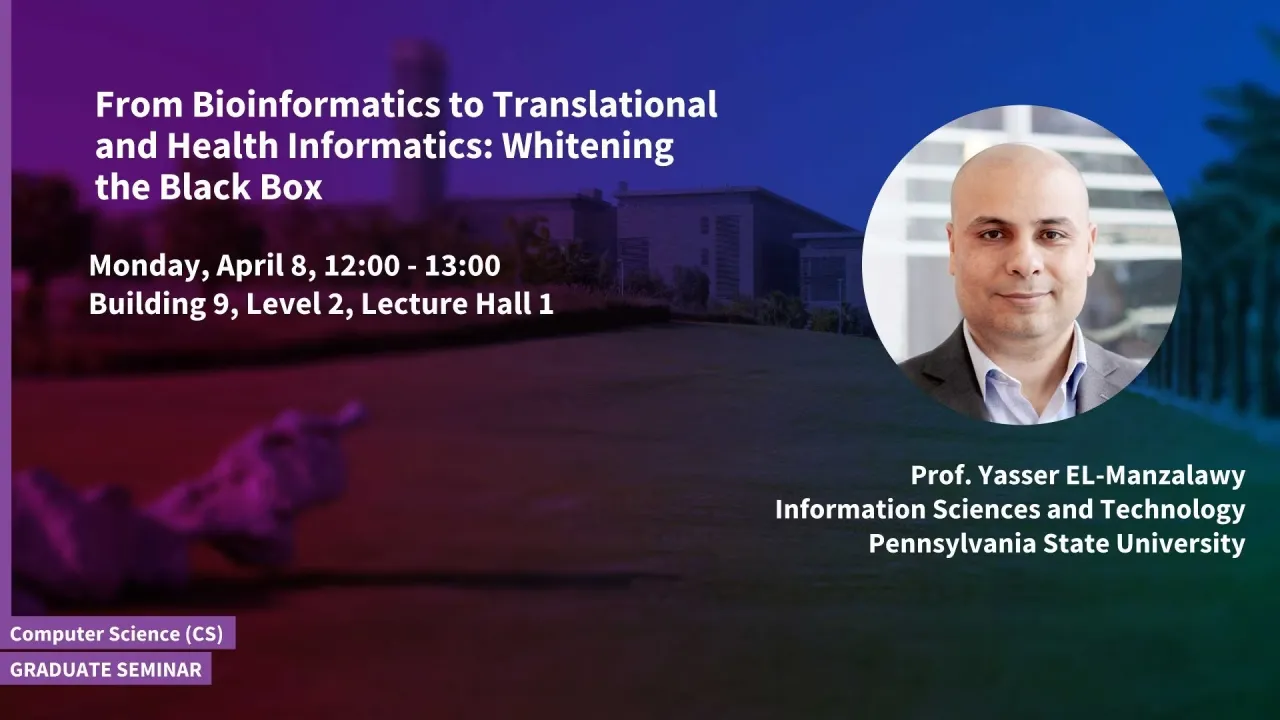
From Bioinformatics to Translational and Health Informatics: Whitening the Black Box
- Prof. Yasser EL-Manzalawy, Information Sciences and Technology, Pennsylvania State University
B9 H1
Machine learning has been extensively used in developing predictive models for a variety of bioinformatics tools. In many of these applications, to achieve the highest possible predictive performance, black box models (e.g., SVMs and Neural Networks) had been preferred over white box models (e.g., Decision Trees and Rule-based models). First, I argue that sacrificing interpretability for the sake of performance is a reasonable decision for many bioinformatics applications. However, I will demonstrate that uncareful utilization of black box models can lead to misinterpretations of the results.
Overview
Abstract
Machine learning has been extensively used in developing predictive models for a variety of bioinformatics tools. In many of these applications, to achieve the highest possible predictive performance, black box models (e.g., SVMs and Neural Networks) had been preferred over white box models (e.g., Decision Trees and Rule-based models). First, I argue that sacrificing interpretability for the sake of performance is a reasonable decision for many bioinformatics applications. However, I will demonstrate that uncareful utilization of black box models can lead to misinterpretations of the results. Second, I argue that interpretability of predictive models is essential for adopting these models in translational and healthcare settings. Unfortunately, using white box models is very challenging due to the high dimensionality, sparsity, and heterogeneity of the data. Alternatively, one can use a black box model augmented with interpretable predictions. Using a simple notion of interpretable predictions, I will present examples from my recent research on developing interpretable models for biomarker discovery from multi-omics and metagenomics data. I will conclude the talk with some discussions on the promise and challenges of adapting these approaches for integrative and predictive analyses of multi-omics, environmental, imaging, and phenotype data extracted from EHR systems.
Brief Biography
Dr. El-Manzalawy is an Assistant Professor at Geisinger Health System and an Adjunct Assistant Professor at College of Information Sciences and Technology, Pennsylvania State University. He holds a PhD in Computer Science from Iowa State University. His long-term research goal is to advance our understanding and make sense of big data in life and health sciences through developing and applying integrative informatics tools and methodologies to enable and accelerate translational science. To date, he has developed several novel algorithmic solutions and machine learning based tools for vaccine informatics, structural bioinformatics, computational genomics, metagenomics, multi-omics data integration, and mHealth. His current research interests focus on the development of novel methodologies, frameworks, and algorithms for integrative analysis of heterogeneous data sources in EHR (including genomics, omics, microbiome, imaging, environmental, and wearables) relevant for precision medicine.
Refreshments: Light lunch will be provided.