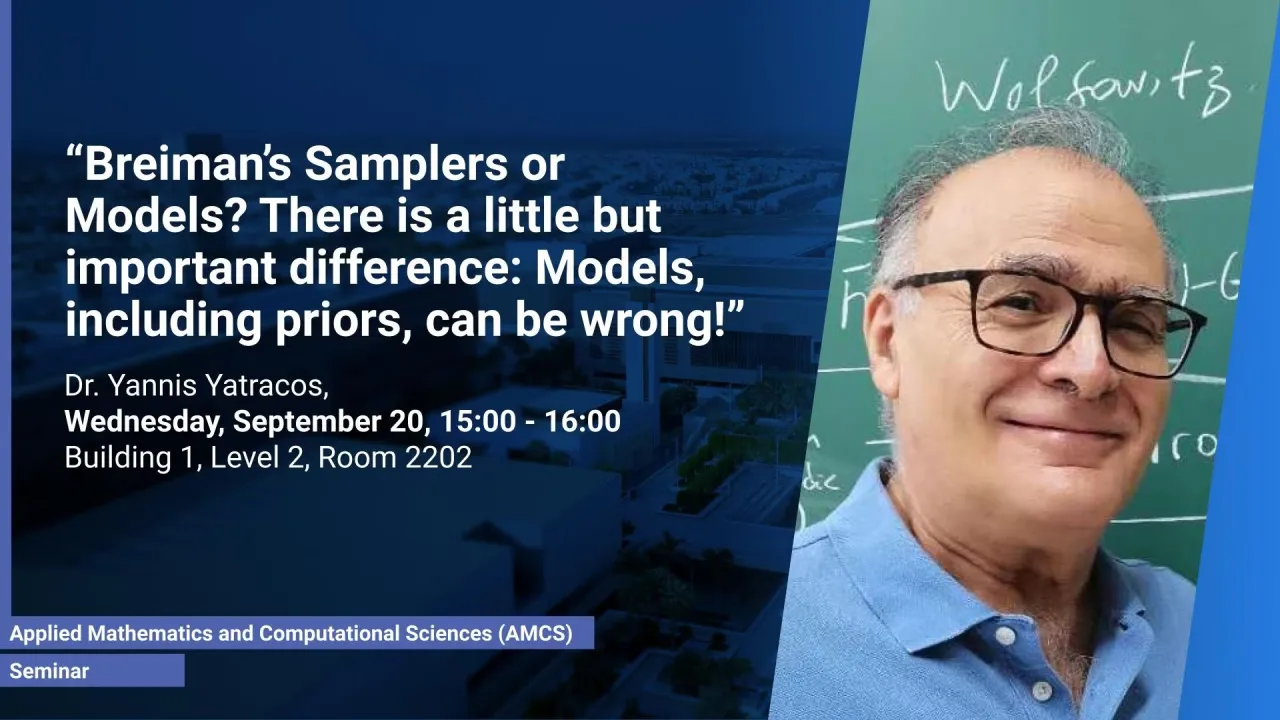
Breiman’s Samplers or Models? There is a little but important difference: Models, including priors, can be wrong!
- Dr. Yannis Yatracos
B1 L2 R2202
Breiman (2001) urged statisticians to provide tools when the data, X=s(θ,Y); sampler s is available as Black-Box, parameter θεΘ, Y is random, either observed or latent. The paper’s discussants, D. R. Cox and B. Efron, looked at the problem as X-prediction, surprisingly neglecting the statistical inference for θ, and disagreed with the main thrust of the paper.
Overview
Abstract
Breiman (2001) urged statisticians to provide tools when the data, X=s(θ,Y); sampler s is available as Black-Box, parameter θεΘ, Y is random, either observed or latent. The paper’s discussants, D. R. Cox and B. Efron, looked at the problem as X-prediction, surprisingly neglecting the statistical inference for θ, and disagreed with the main thrust of the paper. Consequently, mathematical statisticians ignored Breiman’s suggestion! However, computer scientists work with X=s(θ,Y), calling s learning machine. In this talk, following Breiman, statistical inference tools are presented for θ: a) The Empirical Discrimination Index (EDI), to detect θ-discrimination and identifiability. b) Matching estimates of θ with upper bounds on the errors that depend on the “massiveness” of Θ. c) For known stochastic models of X, Laplace’s 1774 Principle for inverse probability is proved without Bayes rule, and for unknown X-models, an Approximate Inverse/Fiducial distribution for θ is obtained. The approach can also be used in ABC, providing F-ABC, that includes all θ* drawn from a Θ-sampler, unlike the Rubin (1984) ABC-rejection method followed until now. The results in a) are unique in the literature (YY, 2023). Mild assumptions are needed in b) and c), unlike existing results that need strong and often unverifiable assumptions. The errors’ upper bounds in b) have the same rate, independent of the data dimension. When Θ is subset of Rm, m unknown, the rate can be [mn (log n)/n]1/2 in probability, with mn increasing to infinity as slow as we wish; when m is known, mn=m. Approximate Fiducial distributions and F-ABC posteriors in c) are obtained for any data dimension. Thus, when X=s(θ,Υ) and a cdf, Fθ, is assumed for X, it seems logical to use instead the sampler, s, and a)-c), since Fθ and an assumed θ-prior may be wrong.
Brief Biography
Yannis Yatracos obtained his Ph.D. from UC Berkeley under Lucien Le Cam. His regular appointments include Tsinghua, Rutgers, Columbia, UCSB, University of Montreal, University of Marseille (at Luminy), Cyprus U. of Technology and the National University of Singapore (NUS). Yannis started his research in density estimation, with calculation of rates of convergence via Minimum Distance methods. He extended these results in non-parametric regression and continued his research in other areas of Statistics and in Actuarial Science. Yannis investigated pathologies of plug-in-methods, like the bootstrap and the MLE, and the limitations of Wasserstein MDE. His most recent research interests include: a) Cluster and Structure Detection for High Dimensional Data, and b) Statistical Foundations of Data Science, in particular for Learning Machines. Yannis is IMS and ASA Fellow, Elected ISI member and was Associate member of the Society of Actuaries (U.S.A.).