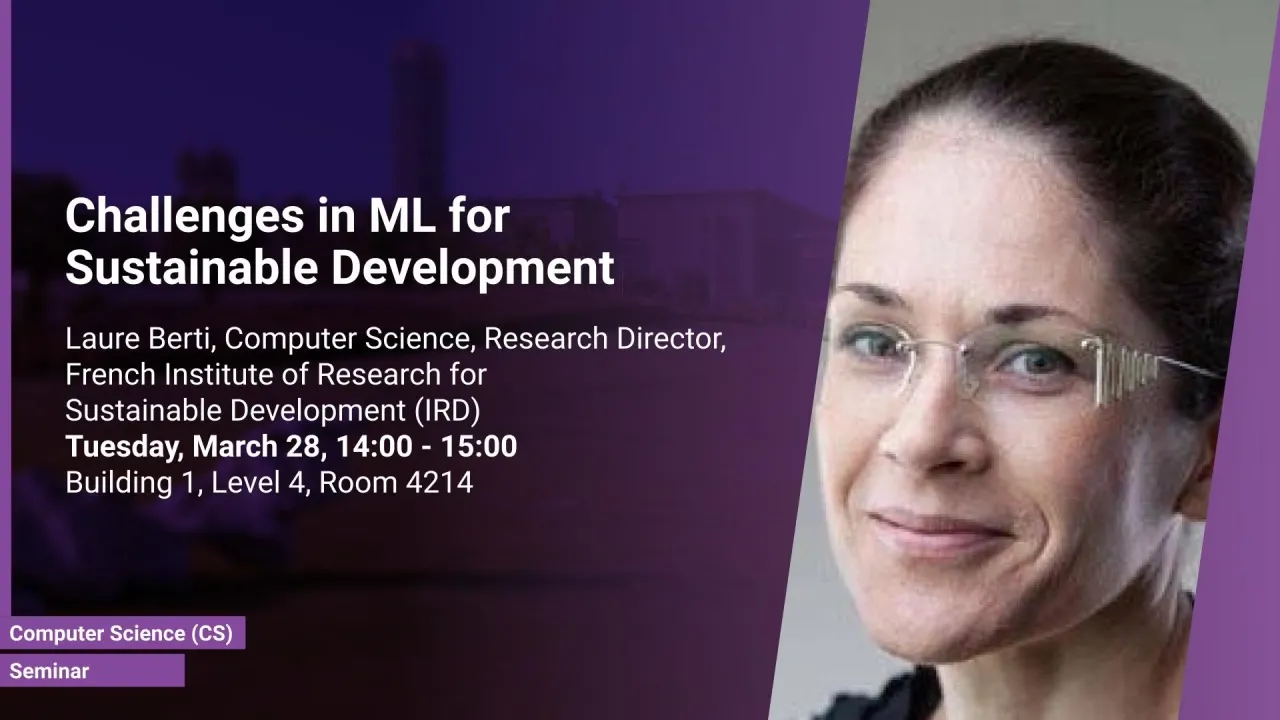
Challenges in ML for Sustainable Development
This talk will present recent approaches for mitigation and adaptation, for which data analytics and ML are parts of the solution in a larger context of interdisciplinary and methodological research and innovations.
Overview
Abstract
Machine learning (ML) techniques can offer powerful tools for addressing some of the greatest challenges faced by humanity and helping our society to adapt to a rapidly changing climate and extreme conditions, respond to disasters and pandemic crises, and eventually reach the United Nations Sustainable Development Goals (UN SDGs) by 2030.
This talk will present recent approaches for mitigation and adaptation, for which data analytics and ML are parts of the solution in a larger context of interdisciplinary and methodological research and innovations. For example, predicting poverty or biodiversity loss at the scale of a continent, a country or a region requires multi-modal and multi-source data fusion combining satellite imagery with other relevant data sources at various spatial and temporal scales, handling noisy and missing ground truth data, and ensembling multiple physics/economics-based and ML models to improve prediction and provide quantified uncertainties. The talk will highlight current theoretical and practical challenges that need to be addressed to better equip our necessary transition toward sustainability with robust ML-based tools and actionable insights to support decision-making.
Brief Biography
Laure Berti-Équille is a Research Director at IRD, the French Research Institute for Sustainable Development. Before she was a full professor in Computer Science at Aix-Marseille University (France), a senior scientist at Qatar Computing Research Institute in Doha (Qatar), a tenured associate professor at the University of Rennes 1 (France), and a 2-years visiting researcher at AT&T Labs Research in New Jersey (USA) as a recipient of the prestigious European Marie Curie Outgoing Fellowship. In 2022, she was a visiting scientist at MIT LIDS (Massachusetts Institute of Technology - Laboratory for Information & Decision Systems) in the DataToAI group. Her current research interests are on the inter-play of data management and machine learning with a focus on anomaly detection, data cleaning and preparation, and data fusion. She has more than 100 publications in major conferences or journals along with two books, and 10 co-edited proceedings. She has co-organized numerous workshops on information and data quality in conjunction with top conferences such as SIGMOD and VLDB. She has given several tutorials and keynote talks on data curation and data engineering for applied machine learning (KDD'22 & '21, ICDE'18 & '16, CIKM'15, KDD'09, ICDM'09). She served in many program committees of international conferences and was an Associate Editor of the VLDB Journal and the ACM Information and Data Quality Journal. She has been leading projects with grants from the French National Agency of Research (ANR), the French National Research Council (CNRS), the Belmont Forum, and the European Union. She is an IEEE and ACM senior member.