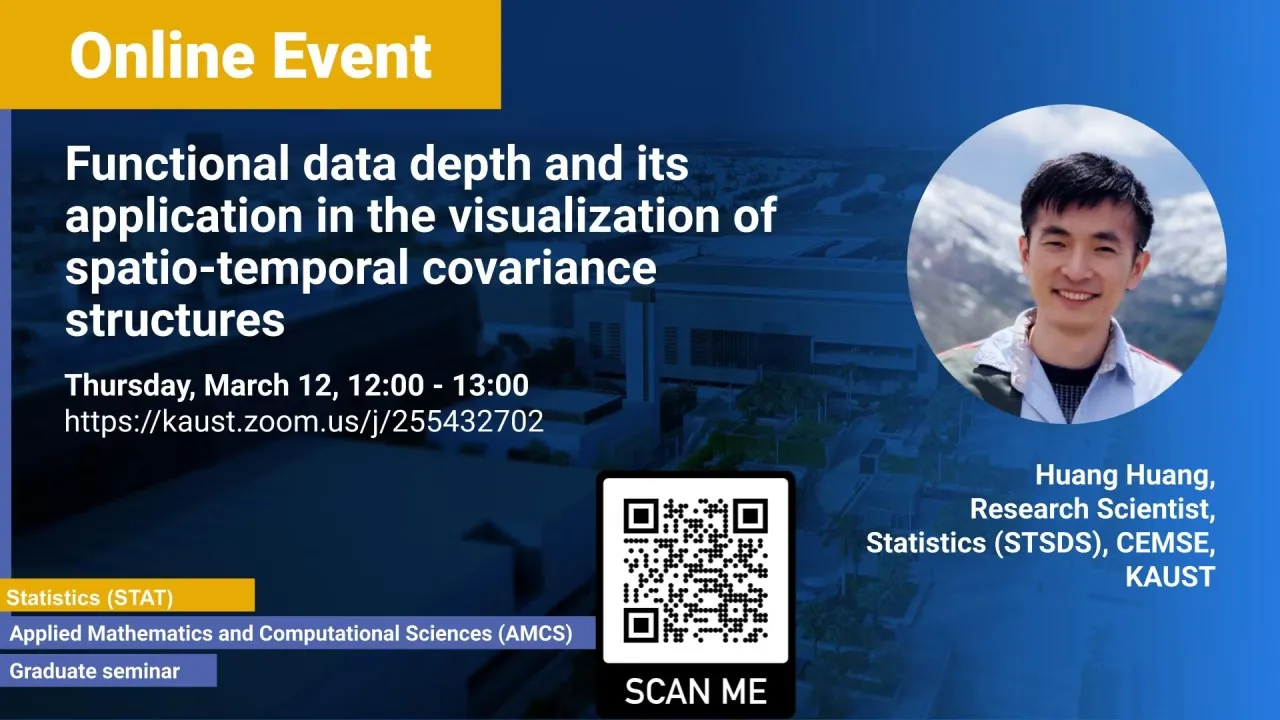
Functional data depth and its application in the visualization of spatio-temporal covariance structures
Functional data analysis is a very active research area due to the overwhelming existence of functional data. In the first part of this talk, I will introduce how functional data depth is used to carry out exploratory data analysis and explain recently-developed depth techniques. In the second part, I will discuss spatio-temporal statistical modeling. It is challenging to build realistic space-time models and assess the validity of the model, especially when datasets are large. I will present a set of visualization tools we developed using functional data analysis techniques for visualizing covariance structures of univariate and multivariate spatio-temporal processes. I will illustrate the performance of the proposed methods in the exploratory data analysis of spatio-temporal data.
Overview
Abstract
Functional data analysis is a very active research area due to the overwhelming existence of functional data. In the first part of this talk, I will introduce how functional data depth is used to carry out exploratory data analysis and explain recently-developed depth techniques. In the second part, I will discuss spatio-temporal statistical modeling. It is challenging to build realistic space-time models and assess the validity of the model, especially when datasets are large. I will present a set of visualization tools we developed using functional data analysis techniques for visualizing covariance structures of univariate and multivariate spatio-temporal processes. I will illustrate the performance of the proposed methods in the exploratory data analysis of spatio-temporal data.
Brief Biography
Huang Huang is a research scientist in the Spatio-Temporal Statistics & Data Science group at KAUST. Before working at KAUST, he did research on statistical computing for climate applications as a postdoc at the National Center for Atmospheric Research (NCAR), the Statistical and Applied Mathematical Sciences Institute (SAMSI), and Duke University. His research interest includes spatio-temporal statistics, functional data analysis, Bayesian modeling, machine learning, and high-performance computing for large datasets.