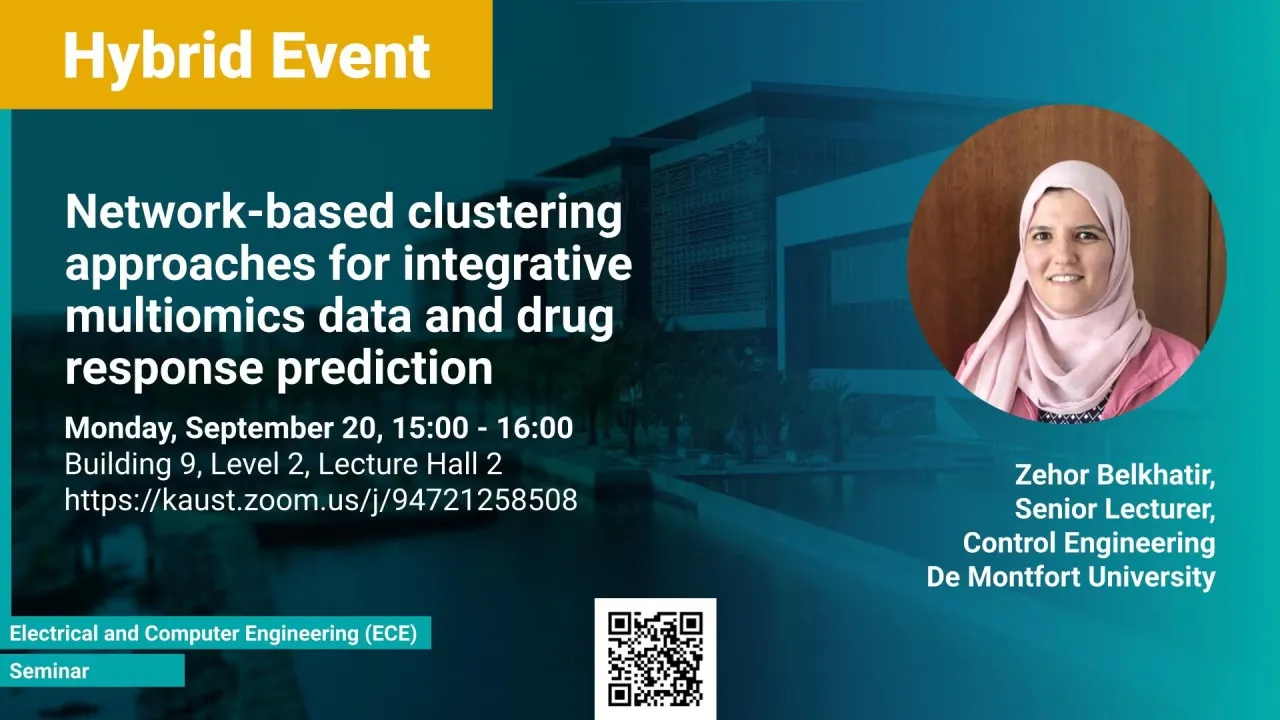
Network-based clustering approaches for integrative multiomics data and drug response prediction
- Zehor Belkhatir, Senior Lecturer, Control Engineering, De Montfort University
B1 L2 H2
Biological data sets, such as gene expressions, copy number alteration, and pharmacogenomics, are often high-dimensional and thus difficult to analyze and interpret. This talk presents two data analysis methodologies that we recently developed based on network analysis via Wasserstein optimal transport combined with unsupervised classification techniques.
Overview
Abstract
Biological data sets, such as gene expressions, copy number alteration, and pharmacogenomics, are often high-dimensional and thus difficult to analyze and interpret. This talk presents two data analysis methodologies that we recently developed based on network analysis via Wasserstein optimal transport combined with unsupervised classification techniques. The proposed prediction pipelines were designed to address the following two research questions: (i) The multiomics data integration challenge that mainly resulted from the remarkable growth of multi-platform genomic profiles; and (ii) the effective linkage of anticancer drug sensitivity to detailed genomic information. The first part of the talk will emphasize on our proposed method of aggregating multiomics and Wasserstein distance clustering, namely aWCluster, to perform the hierarchical clustering of invasive breast carcinoma from The Cancer Genome Atlas (TCGA) project. The subtypes were characterized by the concordant effect of mRNA expression, DNA copy number alteration, DNA methylation, and the interaction network connectivity of the gene products. We show in this part that aWCluster when applied to breast cancer TCGA data, successfully recovered the known PAM50 molecular subtypes.
Moreover, a gene ontology enrichment analysis of significant genes in the low survival subgroup leads to the well-known phenomenon of tumor hypoxia and the transcription factor ETS1, whose expression is induced by hypoxia. The second part of the talk highlights the pipeline we proposed for predicting drug sensitivity in cancer cell lines, which considers both cell line genomic features and drug chemical features. We will show in this part that prior clustering of the heterogeneous cell lines and structurally diverse drugs improve the accuracy of the prediction; and facilities the interpretability of the results and identification of molecular biomarkers, which are significant for both clustering of the cell lines and predicting the drug response.
Brief Biography
Zehor Belkhatir is currently a faculty member (Senior Lecturer in Control Engineering) and Program Leader of BEng/MEng Mechatronics at De Montfort University, Leicester, United Kingdom. She pursued her education in Algeria until she received her MEng. and M.Sc. degrees from “École Nationale Polytechnique” of Algiers in Automatics and Control Systems Engineering. Then, she carried on her doctoral studies at King Abdullah University of Science and Technology (KAUST), Jeddah, Saudi Arabia, where she received her Ph.D. degree in Electrical Engineering. Before joining De Montfort University, she was a Lecturer in Control Engineering at the University of Leicester, and she also held a Postdoctoral Research Scholar position at Memorial Sloan Kettering Cancer Center (MSKCC) in New York, USA. Dr. Belkhatir’s research interests encompass work across the fields of applied mathematics, control systems theory, and data analysis. A common thread in her research is in understanding experimentally constrained complex systems, with an emphasis on systems belonging to the bio-medical/logical fields with severe constraints on information collection from controlled experiments and invasive sensors, by developing theoretical estimation and control techniques and computational tools that rely on mathematical or datadriven models.